diff --git a/.DS_Store b/.DS_Store
new file mode 100644
index 000000000..24d92646f
Binary files /dev/null and b/.DS_Store differ
diff --git a/LICENSE b/LICENSE
new file mode 100644
index 000000000..261eeb9e9
--- /dev/null
+++ b/LICENSE
@@ -0,0 +1,201 @@
+ Apache License
+ Version 2.0, January 2004
+ http://www.apache.org/licenses/
+
+ TERMS AND CONDITIONS FOR USE, REPRODUCTION, AND DISTRIBUTION
+
+ 1. Definitions.
+
+ "License" shall mean the terms and conditions for use, reproduction,
+ and distribution as defined by Sections 1 through 9 of this document.
+
+ "Licensor" shall mean the copyright owner or entity authorized by
+ the copyright owner that is granting the License.
+
+ "Legal Entity" shall mean the union of the acting entity and all
+ other entities that control, are controlled by, or are under common
+ control with that entity. For the purposes of this definition,
+ "control" means (i) the power, direct or indirect, to cause the
+ direction or management of such entity, whether by contract or
+ otherwise, or (ii) ownership of fifty percent (50%) or more of the
+ outstanding shares, or (iii) beneficial ownership of such entity.
+
+ "You" (or "Your") shall mean an individual or Legal Entity
+ exercising permissions granted by this License.
+
+ "Source" form shall mean the preferred form for making modifications,
+ including but not limited to software source code, documentation
+ source, and configuration files.
+
+ "Object" form shall mean any form resulting from mechanical
+ transformation or translation of a Source form, including but
+ not limited to compiled object code, generated documentation,
+ and conversions to other media types.
+
+ "Work" shall mean the work of authorship, whether in Source or
+ Object form, made available under the License, as indicated by a
+ copyright notice that is included in or attached to the work
+ (an example is provided in the Appendix below).
+
+ "Derivative Works" shall mean any work, whether in Source or Object
+ form, that is based on (or derived from) the Work and for which the
+ editorial revisions, annotations, elaborations, or other modifications
+ represent, as a whole, an original work of authorship. For the purposes
+ of this License, Derivative Works shall not include works that remain
+ separable from, or merely link (or bind by name) to the interfaces of,
+ the Work and Derivative Works thereof.
+
+ "Contribution" shall mean any work of authorship, including
+ the original version of the Work and any modifications or additions
+ to that Work or Derivative Works thereof, that is intentionally
+ submitted to Licensor for inclusion in the Work by the copyright owner
+ or by an individual or Legal Entity authorized to submit on behalf of
+ the copyright owner. For the purposes of this definition, "submitted"
+ means any form of electronic, verbal, or written communication sent
+ to the Licensor or its representatives, including but not limited to
+ communication on electronic mailing lists, source code control systems,
+ and issue tracking systems that are managed by, or on behalf of, the
+ Licensor for the purpose of discussing and improving the Work, but
+ excluding communication that is conspicuously marked or otherwise
+ designated in writing by the copyright owner as "Not a Contribution."
+
+ "Contributor" shall mean Licensor and any individual or Legal Entity
+ on behalf of whom a Contribution has been received by Licensor and
+ subsequently incorporated within the Work.
+
+ 2. Grant of Copyright License. Subject to the terms and conditions of
+ this License, each Contributor hereby grants to You a perpetual,
+ worldwide, non-exclusive, no-charge, royalty-free, irrevocable
+ copyright license to reproduce, prepare Derivative Works of,
+ publicly display, publicly perform, sublicense, and distribute the
+ Work and such Derivative Works in Source or Object form.
+
+ 3. Grant of Patent License. Subject to the terms and conditions of
+ this License, each Contributor hereby grants to You a perpetual,
+ worldwide, non-exclusive, no-charge, royalty-free, irrevocable
+ (except as stated in this section) patent license to make, have made,
+ use, offer to sell, sell, import, and otherwise transfer the Work,
+ where such license applies only to those patent claims licensable
+ by such Contributor that are necessarily infringed by their
+ Contribution(s) alone or by combination of their Contribution(s)
+ with the Work to which such Contribution(s) was submitted. If You
+ institute patent litigation against any entity (including a
+ cross-claim or counterclaim in a lawsuit) alleging that the Work
+ or a Contribution incorporated within the Work constitutes direct
+ or contributory patent infringement, then any patent licenses
+ granted to You under this License for that Work shall terminate
+ as of the date such litigation is filed.
+
+ 4. Redistribution. You may reproduce and distribute copies of the
+ Work or Derivative Works thereof in any medium, with or without
+ modifications, and in Source or Object form, provided that You
+ meet the following conditions:
+
+ (a) You must give any other recipients of the Work or
+ Derivative Works a copy of this License; and
+
+ (b) You must cause any modified files to carry prominent notices
+ stating that You changed the files; and
+
+ (c) You must retain, in the Source form of any Derivative Works
+ that You distribute, all copyright, patent, trademark, and
+ attribution notices from the Source form of the Work,
+ excluding those notices that do not pertain to any part of
+ the Derivative Works; and
+
+ (d) If the Work includes a "NOTICE" text file as part of its
+ distribution, then any Derivative Works that You distribute must
+ include a readable copy of the attribution notices contained
+ within such NOTICE file, excluding those notices that do not
+ pertain to any part of the Derivative Works, in at least one
+ of the following places: within a NOTICE text file distributed
+ as part of the Derivative Works; within the Source form or
+ documentation, if provided along with the Derivative Works; or,
+ within a display generated by the Derivative Works, if and
+ wherever such third-party notices normally appear. The contents
+ of the NOTICE file are for informational purposes only and
+ do not modify the License. You may add Your own attribution
+ notices within Derivative Works that You distribute, alongside
+ or as an addendum to the NOTICE text from the Work, provided
+ that such additional attribution notices cannot be construed
+ as modifying the License.
+
+ You may add Your own copyright statement to Your modifications and
+ may provide additional or different license terms and conditions
+ for use, reproduction, or distribution of Your modifications, or
+ for any such Derivative Works as a whole, provided Your use,
+ reproduction, and distribution of the Work otherwise complies with
+ the conditions stated in this License.
+
+ 5. Submission of Contributions. Unless You explicitly state otherwise,
+ any Contribution intentionally submitted for inclusion in the Work
+ by You to the Licensor shall be under the terms and conditions of
+ this License, without any additional terms or conditions.
+ Notwithstanding the above, nothing herein shall supersede or modify
+ the terms of any separate license agreement you may have executed
+ with Licensor regarding such Contributions.
+
+ 6. Trademarks. This License does not grant permission to use the trade
+ names, trademarks, service marks, or product names of the Licensor,
+ except as required for reasonable and customary use in describing the
+ origin of the Work and reproducing the content of the NOTICE file.
+
+ 7. Disclaimer of Warranty. Unless required by applicable law or
+ agreed to in writing, Licensor provides the Work (and each
+ Contributor provides its Contributions) on an "AS IS" BASIS,
+ WITHOUT WARRANTIES OR CONDITIONS OF ANY KIND, either express or
+ implied, including, without limitation, any warranties or conditions
+ of TITLE, NON-INFRINGEMENT, MERCHANTABILITY, or FITNESS FOR A
+ PARTICULAR PURPOSE. You are solely responsible for determining the
+ appropriateness of using or redistributing the Work and assume any
+ risks associated with Your exercise of permissions under this License.
+
+ 8. Limitation of Liability. In no event and under no legal theory,
+ whether in tort (including negligence), contract, or otherwise,
+ unless required by applicable law (such as deliberate and grossly
+ negligent acts) or agreed to in writing, shall any Contributor be
+ liable to You for damages, including any direct, indirect, special,
+ incidental, or consequential damages of any character arising as a
+ result of this License or out of the use or inability to use the
+ Work (including but not limited to damages for loss of goodwill,
+ work stoppage, computer failure or malfunction, or any and all
+ other commercial damages or losses), even if such Contributor
+ has been advised of the possibility of such damages.
+
+ 9. Accepting Warranty or Additional Liability. While redistributing
+ the Work or Derivative Works thereof, You may choose to offer,
+ and charge a fee for, acceptance of support, warranty, indemnity,
+ or other liability obligations and/or rights consistent with this
+ License. However, in accepting such obligations, You may act only
+ on Your own behalf and on Your sole responsibility, not on behalf
+ of any other Contributor, and only if You agree to indemnify,
+ defend, and hold each Contributor harmless for any liability
+ incurred by, or claims asserted against, such Contributor by reason
+ of your accepting any such warranty or additional liability.
+
+ END OF TERMS AND CONDITIONS
+
+ APPENDIX: How to apply the Apache License to your work.
+
+ To apply the Apache License to your work, attach the following
+ boilerplate notice, with the fields enclosed by brackets "[]"
+ replaced with your own identifying information. (Don't include
+ the brackets!) The text should be enclosed in the appropriate
+ comment syntax for the file format. We also recommend that a
+ file or class name and description of purpose be included on the
+ same "printed page" as the copyright notice for easier
+ identification within third-party archives.
+
+ Copyright [yyyy] [name of copyright owner]
+
+ Licensed under the Apache License, Version 2.0 (the "License");
+ you may not use this file except in compliance with the License.
+ You may obtain a copy of the License at
+
+ http://www.apache.org/licenses/LICENSE-2.0
+
+ Unless required by applicable law or agreed to in writing, software
+ distributed under the License is distributed on an "AS IS" BASIS,
+ WITHOUT WARRANTIES OR CONDITIONS OF ANY KIND, either express or implied.
+ See the License for the specific language governing permissions and
+ limitations under the License.
diff --git a/README.md b/README.md
index 81463bfd7..c2658897e 100644
--- a/README.md
+++ b/README.md
@@ -1,17 +1,350 @@
-# intel-oneAPI
+### Team Name - C5ailabs
+### Problem Statement - Open Innovation in Education
+### Team Leader Email - rohit.sroch@course5i.com
+### Other Team Mates Email - (mohan.rachumallu@course5i.com, sujith.kumar@course5i.com, shubham.jain@course5i.com)
-#### Team Name -
-#### Problem Statement -
-#### Team Leader Email -
+### PPT - https://github.com/rohitc5/intel-oneAPI/blob/main/ppt/Intel-oneAPI-Hackathon-Implementation.pdf
+### Medium Article - https://medium.com/@rohitsroch/leap-learning-enhancement-and-assistance-platform-powered-by-intel-oneapi-ai-analytics-toolkit-656b5c9d2e0c
+### Benchmark Results - https://github.com/rohitc5/intel-oneAPI/tree/main/benchmark on Intel® Dev Cloud machine [Intel® Xeon® Platinum 8480+ (4th Gen: Sapphire Rapids) - 224v CPUs 503GB RAM]
+### Demo Video - https://www.youtube.com/watch?v=CXkR5tklZm0
-## A Brief of the Prototype:
- This section must include UML Daigrms and prototype description
+
+
+# LEAP
+
+Intel® oneAPI Hackathon 2023 - Prototype Implementation for our LEAP Solution
+
+[](https://opensource.org/license/apache-2-0/)
+[](https://github.com/rohitc5/intel-oneAPI/releases)
+[](https://vscode.dev/redirect?url=vscode://ms-vscode-remote.remote-containers/cloneInVolume?url=https://github.com/rohitc5/intel-oneAPI)
+[](https://codespaces.new/rohitc5/intel-oneAPI)
+[](https://star-history.com/#rohitc5/intel-oneAPI)
+
+# A Brief of the Prototype:
+
+#### INSPIRATION 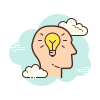
+
+
+MOOCs (Massive Open Online Courses) have surged in popularity in recent years, particularly during the COVID-19 pandemic. These
+online courses are typically free or low-cost, making education more accessible worldwide.
+
+Online learning is crucial for students even post-pandemic due to its flexibility, accessibility, and quality. But still, the learning experience for students is not optimal, as in case of doubts they need to repeatedly go through videos and documents or ask in the forum which may not be effective because of the following challenges:
+
+- Resolving doubts can be a time-consuming process.
+- It can be challenging to sift through pile of lengthy videos or documents to find relevant information.
+- Teachers or instructors may not be available around the clock to offer guidance
+
+#### PROPOSED SOLUTION 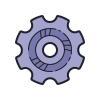
+
+To mitigate the above challenges, we propose LEAP (Learning Enhancement and Assistance Platform), which is an AI-powered
+platform designed to enhance student learning outcomes and provide equitable access to quality education. The platform comprises two main features that aim to improve the overall learning experience of the student:
+
+❑ Ask Question/Doubt: This allows the students to ask real-time questions around provided reading material, which includes videos and
+documents, and get back answers along with the exact timestamp in the video clip containing the answer (so that students don’t have to
+always scroll through). Also, It supports asking multilingual question, ensuring that language barriers do not hinder a student's learning
+process.
+
+❑ Interactive Conversational AI Examiner: This allows the students to evaluate their knowledge about the learned topic through an AI
+examiner conducting viva after each learning session. The AI examiner starts by asking question and always tries to motivate and provide
+hints to the student to arrive at correct answer, enhancing student engagement and motivation.
+
+# Detailed LEAP Process Flow:
+
+
+
+# Technology Stack:
+
+- [Intel® oneAPI AI Analytics Toolkit](https://www.intel.com/content/www/us/en/developer/tools/oneapi/ai-analytics-toolkit-download.html) Tech Stack
+
+ 
+
+1. [Intel® Extension for Pytorch](https://github.com/intel/intel-extension-for-pytorch): Used for our Multilingual Extractive QA model Training/Inference optimization.
+2. [Intel® Neural Compressor](https://github.com/intel/neural-compressor): Used for Multilingual Extractive QA model Inference optimization.
+3. [Intel® Extension for Scikit-Learn](https://github.com/intel/scikit-learn-intelex): Used for Multilingual Embedding model Training/Inference optimization.
+4. [Intel® distribution for Modin](https://github.com/modin-project/modin): Used for basic initial data analysis/EDA.
+
+- Prototype webapp Tech Stack
+
+ 
+
+# Demo Video
+
+[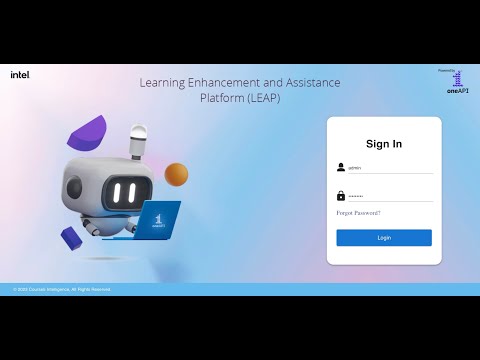](https://www.youtube.com/watch?v=CXkR5tklZm0)
+
+# Step-by-Step Code Execution Instructions:
+
+### Quick Setup Option
+
+- Make sure you have already installed docker (https://docs.docker.com/get-docker/) and docker-compose (https://docs.docker.com/compose/)
+
+- Clone the Repository
+```python
+ $ git clone https://github.com/rohitc5/intel-oneAPI/
+ $ cd intel-oneAPI
+
+```
+- Start the LEAP RESTFul Service to consume both components (Ask Question/Doubt and Interactive Conversational AI Examiner) as a REST API.
+Also Start the webapp demo build using streamlit.
+
+```python
+ # copy the dataset
+ $ cp -r ./dataset webapp/
+
+ # build using docker compose
+ $ docker compose build
+
+ # start the services
+ $ docker compose up
+```
+
+- Go to http://localhost:8502
+
+
+### Manual Setup Option
+
+- Clone the Repository
+
+```python
+ $ git clone https://github.com/rohitc5/intel-oneAPI/
+ $ cd intel-oneAPI
+```
+
+- Train/Fine-tune the Extractive QA Multilingual Model (Part of our **Ask Question/Doubt** Component).
+Please note that, by default we use this (https://huggingface.co/ai4bharat/indic-bert) as a Backbone (BERT topology)
+and finetune it on SQuAD v1 dataset. Moreover, IndicBERT is a multilingual ALBERT model pretrained exclusively on 12 major Indian languages. It is pre-trained on novel monolingual corpus of around 9 billion tokens and subsequently evaluated on a set of diverse tasks. So finetuning, on SQuAD v1 (English) dataset automatically results in cross-lingual
+transfer on other 11 indian languages.
+
+Here is the architecture of `Ask Question/Doubt` component:
+
+
+
+```python
+ $ cd nlp/question_answering
+
+ # install dependencies
+ $ pip install -r requirements.txt
+
+ # modify the fine-tuning params mentioned in finetune_qa.sh
+ $ vi finetune_qa.sh
+
+ ''''
+ export MODEL_NAME_OR_PATH=ai4bharat/indic-bert
+ export BACKBONE_NAME=indic-mALBERT-uncased
+ export DATASET_NAME=squad # squad, squad_v2 (pass --version_2_with_negative)
+ export TASK_NAME=qa
+
+ # hyperparameters
+ export SEED=42
+ export BATCH_SIZE=32
+ export MAX_SEQ_LENGTH=384
+ export NUM_TRAIN_EPOCHS=5
+
+ export KEEP_ACCENTS=True
+ export DO_LOWER_CASE=True
+ ...
+
+ ''''
+
+ # start the training after modifying params
+ $ bash finetune_qa.sh
+```
+
+- Perform Post Training Optimization of Extractive QA model using IPEX (Intel® Extension for Pytorch), Intel® Neural Compressor and run the benchmark for comparison with Pytorch(Base)-FP32
+
+```python
+ # modify the params in pot_benchmark_qa.sh
+ $ vi pot_benchmark_qa.sh
+
+ ''''
+ export MODEL_NAME_OR_PATH=artifacts/qa/squad/indic-mALBERT-uncased
+ export BACKBONE_NAME=indic-mALBERT-uncased
+ export DATASET_NAME=squad # squad, squad_v2 (pass --version_2_with_negative)
+ export TASK_NAME=qa
+ export USE_OPTIMUM=True # whether to use hugging face wrapper optimum around intel neural compressor
+
+ # other parameters
+ export BATCH_SIZE=8
+ export MAX_SEQ_LENGTH=384
+ export DOC_STRIDE=128
+ export KEEP_ACCENTS=True
+ export DO_LOWER_CASE=True
+ export MAX_EVAL_SAMPLES=200
+
+ export TUNE=True # whether to tune or not
+ export PTQ_METHOD="static_int8" # "dynamic_int8", "static_int8", "static_smooth_int8"
+ export BACKEND="default" # default, ipex
+ export ITERS=100
+ ...
+
+ ''''
-## Tech Stack:
- List Down all technologies used to Build the prototype **Clearly mentioning Intel® AI Analytics Toolkits, it's libraries and the SYCL/DCP++ Libraries used**
-
-## Step-by-Step Code Execution Instructions:
- This Section must contain set of instructions required to clone and run the prototype, so that it can be tested and deeply analysed
+ $ bash pot_benchmark_qa.sh
+
+ # Please note that, above shell script can perform optimization using IPEX to get Pytorch-(IPEX)-FP32 model
+ # or It can perform optimization/quantization using Intel® Neural Compressor to get Static-QAT-INT8,
+ # Static-Smooth-QAT-INT8 models. Moreover, you can choose the backend as `default` or `ipex` for INT8 models.
+
+```
+
+- Run quick inference to test the model output
+
+```python
+ $ python run_qa_inference.py --model_name_or_path=[FP32 or INT8 finetuned model] --model_type=["vanilla_fp32" or "quantized_int8"] --do_lower_case --keep_accents --ipex_enable
+
+```
+
+- Train/Infer/Benchmark TFIDFVectorizer Embedding model for Scikit-Learn (Base) vs Intel® Extension for Scikit-Learn
+
+```python
+ $ cd nlp/feature_extractor
+
+ # train (.fit_transform func), infer (.transform func) and perform benchmark
+ $ python run_benchmark_tfidf.py --course_dir=../../dataset/courses --is_preprocess
+
+ # now rerun but turn on Intel® Extension for Scikit-Learn
+ $ python run_benchmark_tfidf.py --course_dir=../../dataset/courses --is_preprocess --intel_scikit_learn_enabled
+```
+
+- Setup LEAP API
+
+```python
+ $ cd api
+
+ # install dependencies
+ $ pip install -r requirements.txt
+
+ $ cd src/
+
+ # create a local vector store of course content for faster retrieval during inference
+ # Here we get semantic or syntactic (TFIDF) embedding of each content from course and index it.
+ # Please Note that, we use FAISS (local vector store) (https://github.com/facebookresearch/faiss) to create a course content index
+ $ python core/create_vector_index.py --course_dir=../../dataset/courses --emb_model_type=[semantic or syntactic] \
+ --model_name_or_path=[Hugging face model name for semantic] --keep_accents
-## What I Learned:
- Write about the biggest learning you had while developing the prototype
+ # update config.py
+ $ cd ../
+ $ vi config.py
+
+ ''''
+ ASK_DOUBT_CONFIG = {
+ # hugging face BERT topology model name
+ "emb_model_name_or_path": "ai4bharat/indic-bert",
+ "emb_model_type": "semantic", #options: syntactic, semantic
+
+ # finetuned Extractive QA model path previously done
+ "qa_model_name_or_path": "rohitsroch/indic-mALBERT-squad-v2",
+ "qa_model_type": "vanilla_fp32", #options: vanilla_fp32, quantized_int8
+
+ # faiss index file path created previously
+ "faiss_vector_index_path": "artifacts/index/faiss_emb_index"
+ }
+ ...
+
+ ''''
+```
+Please Note that, we have already released our finetuned Extractive QA model available on Huggingface Hub (https://huggingface.co/rohitsroch/indic-mALBERT-squad-v2)
+
+- For our **Interactive Conversational AI Examiner** Component, we use an Instruct-tuned or RLHF Large Language model (LLM) based on recent advancements in Generative AI. You can update the API configuration by specifying hf_model_name (LLM name available in huggingface Hub). Please checkout https://huggingface.co/models for LLMs.
+
+Here is the architecture of `Interactive Conversational AI Examiner` component:
+
+
+
+We can use several open access instruction tuned LLMs from hugging face Hub like MPT-7B-instruct (https://huggingface.co/mosaicml/mpt-7b-instruct), Falcon-7B-instruct (https://huggingface.co/TheBloke/falcon-7b-instruct-GPTQ), etc. (follow https://huggingface.co/models for more options.)
+You need to set the `llm_method` as `hf_pipeline` for this. Here for performance gain, we can use INT8 quantized model optimized using Intel® Neural Compressor (follow https://huggingface.co/Intel)
+
+Moreover, for doing much faster inference we can use open access instruction tuned Adapters (LoRA) with backbone as LLaMA from hugging face Hub like QLoRA
+(https://huggingface.co/timdettmers). You need to set the `llm_method` as `hf_peft` for this. Please follow **QLoRA** research paper (https://arxiv.org/pdf/2305.14314.pdf) for more details.
+
+Please Note that for fun 😄, we also provide usage of Azure OpenAI Service to use models like GPT3 over paid subscription API. You just need to provide `azure_deployment_name`, set `llm_method` as `azure_gpt3` in the below configuration and then add ``
+
+```python
+
+ ''''
+ AI_EXAMINER_CONFIG = {
+ "llm_method": "azure_gpt3", #options: azure_gpt3, hf_pipeline, hf_peft
+
+ "azure_gpt3":{
+ "deployment_name": "text-davinci-003-prod",
+ ...
+ },
+ "hf_pipeline":{
+ "model_name": "tiiuae/falcon-7b-instruct"
+ ...
+ }
+ "hf_peft":{
+ "model_name": "huggyllama/llama-7b",
+ "adapter_name": "timdettmers/qlora-alpaca-7b",
+ ...
+ }
+ }
+
+ # provide your api key
+ os.environ["OPENAI_API_KEY"] = ""
+
+ ''''
+```
+
+- Start the API server
+
+```python
+ $ cd api/src/
+
+ # start the gunicorn server
+ $ bash start.sh
+```
+
+- Start the Streamlit webapp demo
+
+```python
+ $ cd webapp
+
+ # update the config
+ $ vi config.py
+
+ ''''
+ # set the correct dataset path
+ DATASET_COURSE_BASE_DIR = "../dataset/courses/"
+
+ API_CONFIG = {
+ "server_host": "localhost", # set the server host
+ "server_port": "8500", # set the server port
+
+ }
+ ...
+
+ ''''
+
+ # install dependencies
+ $ pip install -r requirements.txt
+
+ $ streamlit run app.py
+
+```
+
+- Go to http://localhost:8502
+
+# Benchmark Results with Intel® oneAPI AI Analytics Toolkit
+
+- We follow the below process flow to optimize our models from both the components
+
+
+
+- We have already added several benchmark results to compare how beneficial Intel® oneAPI AI Analytics Toolkit is compared to baseline. Please go to `benchmark` folder to view the results. Please Note that the shared results are based
+on provided Intel® Dev Cloud machine *[Intel® Xeon® Platinum 8480+ (4th Gen: Sapphire Rapids) - 224v CPUs 503GB RAM]*
+
+# Comprehensive Implementation PPT & Article
+
+- Please go to PPT 🎉 https://github.com/rohitc5/intel-oneAPI/blob/main/ppt/Intel-oneAPI-Hackathon-Implementation.pdf for more details
+
+- Please go to Article 📄 https://medium.com/@rohitsroch/leap-learning-enhancement-and-assistance-platform-powered-by-intel-oneapi-ai-analytics-toolkit-656b5c9d2e0c for more details
+
+# What we learned 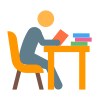
+
+
+
+✅ **Utilizing the Intel® AI Analytics Toolkit**: By utilizing the Intel® AI Analytics Toolkit, developers can leverage familiar Python* tools and frameworks to accelerate the entire data science and analytics process on Intel® architecture. This toolkit incorporates oneAPI libraries for optimized low-level computations, ensuring maximum performance from data preprocessing to deep learning and machine learning tasks. Additionally, it facilitates efficient model development through interoperability.
+
+✅ **Seamless Adaptability**: The Intel® AI Analytics Toolkit enables smooth integration with machine learning and deep learning workloads, requiring minimal modifications.
+
+✅ **Fostered Collaboration**: The development of such an application likely involved collaboration with a team comprising experts from diverse fields, including deep learning and data analysis. This experience likely emphasized the significance of collaborative efforts in attaining shared objectives.
diff --git a/api/.DS_Store b/api/.DS_Store
new file mode 100644
index 000000000..6bf478d2f
Binary files /dev/null and b/api/.DS_Store differ
diff --git a/api/Dockerfile b/api/Dockerfile
new file mode 100644
index 000000000..f6bae43f4
--- /dev/null
+++ b/api/Dockerfile
@@ -0,0 +1,17 @@
+FROM python:3.9
+
+RUN apt-get update && apt-get -y install \
+ build-essential libpq-dev ffmpeg libsm6 libxext6 wget
+
+WORKDIR /opt
+COPY requirements.txt requirements.txt
+
+RUN pip install --upgrade pip
+
+RUN pip install -r requirements.txt
+
+COPY src/ .
+
+EXPOSE 8500
+
+ENTRYPOINT [ "bash", "start.sh" ]
diff --git a/api/README.md b/api/README.md
new file mode 100644
index 000000000..e69de29bb
diff --git a/api/requirements.txt b/api/requirements.txt
new file mode 100644
index 000000000..a9e5b053e
--- /dev/null
+++ b/api/requirements.txt
@@ -0,0 +1,21 @@
+fastapi==0.96.0
+uvicorn[standard]==0.22.0
+gunicorn==20.1.0
+pydantic==1.10.8
+python-multipart==0.0.6
+Cython==0.29.35
+pandas==2.0.2
+tiktoken==0.4.0
+langchain==0.0.191
+openai==0.27.8
+faiss-cpu==1.7.4
+nltk==3.8.1
+torch==2.0.1
+transformers==4.29.2
+peft==0.3.0
+optimum[neural-compressor]==1.8.7
+neural-compressor==2.1.1
+webvtt-py==0.4.6
+intel_extension_for_pytorch==2.0.100
+scikit-learn==1.2.2
+scikit-learn-intelex==2023.1.1
diff --git a/api/src/.DS_Store b/api/src/.DS_Store
new file mode 100644
index 000000000..9bcbe9c46
Binary files /dev/null and b/api/src/.DS_Store differ
diff --git a/api/src/__init__.py b/api/src/__init__.py
new file mode 100644
index 000000000..e69de29bb
diff --git a/api/src/artifacts/.DS_Store b/api/src/artifacts/.DS_Store
new file mode 100644
index 000000000..83bd1a599
Binary files /dev/null and b/api/src/artifacts/.DS_Store differ
diff --git a/api/src/artifacts/index/.gitignore b/api/src/artifacts/index/.gitignore
new file mode 100644
index 000000000..e69de29bb
diff --git a/api/src/artifacts/index/faiss_emb_index/index.faiss b/api/src/artifacts/index/faiss_emb_index/index.faiss
new file mode 100755
index 000000000..7211b76c6
Binary files /dev/null and b/api/src/artifacts/index/faiss_emb_index/index.faiss differ
diff --git a/api/src/artifacts/index/faiss_emb_index/index.pkl b/api/src/artifacts/index/faiss_emb_index/index.pkl
new file mode 100755
index 000000000..317c43f9d
Binary files /dev/null and b/api/src/artifacts/index/faiss_emb_index/index.pkl differ
diff --git a/api/src/artifacts/model/.gitignore b/api/src/artifacts/model/.gitignore
new file mode 100644
index 000000000..e69de29bb
diff --git a/api/src/config.py b/api/src/config.py
new file mode 100644
index 000000000..33c055874
--- /dev/null
+++ b/api/src/config.py
@@ -0,0 +1,105 @@
+import json
+import os
+
+PORT = 8500
+
+ASK_DOUBT_CONFIG = {
+ "emb_model_name_or_path": "ai4bharat/indic-bert",
+ "emb_model_type": "semantic", #options: syntactic, semantic
+ "qa_model_name_or_path": "rohitsroch/indic-mALBERT-squad-v2",
+ "qa_model_type": "vanilla_fp32", #options: vanilla_fp32, quantized_int8
+
+ "intel_scikit_learn_enabled": True,
+ "ipex_enabled": True,
+ "keep_accents": True,
+ "normalize_L2": False,
+ "do_lower_case": True,
+ "faiss_vector_index_path": "artifacts/index/faiss_emb_index"
+}
+
+
+AI_EXAMINER_CONFIG = {
+ "llm_method": "azure_gpt3", #options: azure_gpt3, hf_pipeline, hf_peft
+
+ "azure_gpt3":{
+ "deployment_name": "text-davinci-003-prod",
+ "llm_kwargs": {
+ "temperature": 0.3,
+ "max_tokens": 300,
+ "n": 1,
+ "top_p": 1.0,
+ "frequency_penalty": 1.1
+ }
+ },
+ "hf_pipeline":{
+ "model_name": "tiiuae/falcon-7b-instruct",
+ "task": "text-generation",
+ "device": -1,
+ "llm_kwargs":{
+ "torch_dtype": "float16", #bfloat16, float16, float32
+ "device_map": "auto",
+ "load_in_4bit": True,
+ "max_memory": "24000MB",
+ "trust_remote_code": True
+ },
+ "pipeline_kwargs": {
+ "max_new_tokens": 300,
+ "top_p": 0.15,
+ "top_k": 0,
+ "temperature": 0.3,
+ "repetition_penalty": 1.1,
+ "num_return_sequences": 1,
+ "do_sample": True,
+ "stop_sequence": []
+ },
+ "quantization_kwargs": {
+ "load_in_4bit": True, # do 4 bit quantization
+ "load_in_8bit": False,
+ "bnb_4bit_compute_dtype": "float16", #bfloat16, float16, float32
+ "bnb_4bit_use_double_quant": True,
+ "bnb_4bit_quant_type": "nf4"
+ }
+ },
+ "hf_peft":{
+ "model_name": "huggyllama/llama-7b",
+ "adapter_name": "timdettmers/qlora-alpaca-7b",
+ "task": "text-generation",
+ "device": -1,
+ "llm_kwargs":{
+ "torch_dtype": "float16", #bfloat16, float16, float32
+ "device_map": "auto",
+ "load_in_4bit": True,
+ "max_memory": "32000MB",
+ "trust_remote_code": True
+ },
+ "generation_kwargs": {
+ "max_new_tokens": 300,
+ "top_p": 0.15,
+ "top_k": 0,
+ "temperature": 0.3,
+ "repetition_penalty": 1.1,
+ "num_return_sequences": 1,
+ "do_sample": True,
+ "early_stopping": True,
+ "stop_sequence": []
+ },
+ "quantization_kwargs": {
+ "load_in_4bit": True, # do 4 bit quantization
+ "load_in_8bit": False,
+ "bnb_4bit_compute_dtype": "float16", #bfloat16, float16, float32
+ "bnb_4bit_use_double_quant": True,
+ "bnb_4bit_quant_type": "nf4"
+ }
+ }
+}
+
+os.environ["TOKENIZERS_PARALLELISM"] = "True"
+
+# Set this to `azure`
+os.environ["OPENAI_API_TYPE"]= "azure"
+# The API version you want to use: set this to `2022-12-01` for the released version.
+os.environ["OPENAI_API_VERSION"] = "2022-12-01"
+# The base URL for your Azure OpenAI resource. You can find this in the Azure portal under your Azure OpenAI resource.
+os.environ["OPENAI_API_BASE"] = "https://c5-openai-research.openai.azure.com/"
+# The API key for your Azure OpenAI resource. You can find this in the Azure portal under your Azure OpenAI resource.
+os.environ["OPENAI_API_KEY"] = ""
diff --git a/api/src/core/.gitignore b/api/src/core/.gitignore
new file mode 100644
index 000000000..e69de29bb
diff --git a/api/src/core/__init__.py b/api/src/core/__init__.py
new file mode 100644
index 000000000..8b1378917
--- /dev/null
+++ b/api/src/core/__init__.py
@@ -0,0 +1 @@
+
diff --git a/api/src/core/common.py b/api/src/core/common.py
new file mode 100644
index 000000000..5c69eedcf
--- /dev/null
+++ b/api/src/core/common.py
@@ -0,0 +1,14 @@
+import six
+
+
+def convert_to_unicode(text):
+ """Converts `text` to Unicode (if it's not already), assuming utf-8 input."""
+ if six.PY3:
+ if isinstance(text, str):
+ return text
+ elif isinstance(text, bytes):
+ return text.decode("utf-8", "ignore")
+ else:
+ raise ValueError("Unsupported string type: %s" % (type(text)))
+ else:
+ raise ValueError("Not running on Python2 or Python 3?")
\ No newline at end of file
diff --git a/api/src/core/constants.py b/api/src/core/constants.py
new file mode 100644
index 000000000..121d2362b
--- /dev/null
+++ b/api/src/core/constants.py
@@ -0,0 +1 @@
+WARNING_PREFIX = ""
\ No newline at end of file
diff --git a/api/src/core/create_vector_index.py b/api/src/core/create_vector_index.py
new file mode 100644
index 000000000..443e9078d
--- /dev/null
+++ b/api/src/core/create_vector_index.py
@@ -0,0 +1,207 @@
+
+#!/usr/bin/env python
+# coding=utf-8
+# Copyright 2023 C5ailabs Team (Authors: Rohit Sroch) All rights reserved.
+#
+# Licensed under the Apache License, Version 2.0 (the "License");
+# you may not use this file except in compliance with the License.
+# You may obtain a copy of the License at
+#
+# http://www.apache.org/licenses/LICENSE-2.0
+#
+# Unless required by applicable law or agreed to in writing, software
+# distributed under the License is distributed on an "AS IS" BASIS,
+# WITHOUT WARRANTIES OR CONDITIONS OF ANY KIND, either express or implied.
+# See the License for the specific language governing permissions and
+# limitations under the License.
+"""
+Create a retriever vector index for faster search during inference using FAISS
+"""
+import argparse
+import os
+import sys
+sys.path.append(os.getcwd())
+import webvtt
+from glob import glob
+
+from core.retrievers.huggingface import HuggingFaceEmbeddings
+from core.retrievers.tfidf import TFIDFEmbeddings
+from core.retrievers.faiss_vector_store import FAISS
+from core.retrievers.docstore import Document
+
+def get_subtitles_as_docs(course_dir):
+ # format of courses folder structure is courses/{topic_name}/{week_name}/{subtopic_name}/subtitle-en.vtt
+ path = os.path.join(course_dir, "*/*/*/*.vtt")
+ subtitle_fpaths = glob(path)
+
+ docs = []
+ for subtitle_fpath in subtitle_fpaths:
+ topic_name = subtitle_fpath.split("/")[-4]
+ week_name = subtitle_fpath.split("/")[-3]
+ subtopic_name = subtitle_fpath.split("/")[-2]
+ file_name = subtitle_fpath.split("/")[-1]
+
+ subtitles = webvtt.read(subtitle_fpath)
+ for index, subtitle in enumerate(subtitles):
+ docs.append(
+ Document(
+ page_content=subtitle.text,
+ metadata={
+ "doc_id": index,
+ "start_timestamp": subtitle.start,
+ "end_timestamp": subtitle.end,
+ "topic_name": topic_name,
+ "week_name": week_name,
+ "subtopic_name": subtopic_name,
+ "file_name": file_name,
+ "fpath": subtitle_fpath
+ })
+ )
+
+ return docs
+
+def load_emb_model(
+ model_name_or_path,
+ model_type="semantic",
+ intel_scikit_learn_enabled=True,
+ ipex_enabled=False,
+ model_revision="main",
+ keep_accents=False,
+ cache_dir=".cache"
+ ):
+ """load a Embedding model"""
+ emb_model = None
+
+ if model_type == "semantic":
+ emb_model = HuggingFaceEmbeddings(
+ model_name_or_path=model_name_or_path,
+ ipex_enabled=ipex_enabled,
+ hf_kwargs={
+ "model_revision": model_revision,
+ "keep_accents": keep_accents,
+ "cache_dir": cache_dir
+ }
+ )
+ elif model_type == "syntactic":
+ emb_model = TFIDFEmbeddings(
+ intel_scikit_learn_enabled=intel_scikit_learn_enabled
+ )
+
+ return emb_model
+
+
+def main(args):
+
+ if args.emb_model_type == "semantic":
+ print("**********Creating a Semantic vector index using \
+ HuggingFaceEmbeddings with `model_name_or_path`={}**********".format(
+ args.model_name_or_path
+ ))
+ elif args.emb_model_type == "syntactic":
+ print("**********Creating a Syntactic vector index using TFIDFEmbeddings**********")
+
+ # get the contexts as docs with metadata
+ docs = get_subtitles_as_docs(args.course_dir)
+ # get the embedding model
+ emb_model = load_emb_model(
+ args.model_name_or_path,
+ model_type=args.emb_model_type,
+ intel_scikit_learn_enabled=args.intel_scikit_learn_enabled,
+ ipex_enabled=args.ipex_enabled,
+ keep_accents=args.keep_accents
+ )
+
+ vector_index = FAISS.from_documents(
+ docs, emb_model, normalize_L2=args.normalize_L2)
+ save_path = "faiss_emb_index" if args.output_dir is None \
+ else os.path.join(args.output_dir, "faiss_emb_index")
+ vector_index.save_local(save_path)
+
+ if args.emb_model_type == "syntactic":
+ emb_model.save_tfidf_vocab(emb_model.vectorizer.vocabulary_, save_path)
+
+ print("*"*100)
+ print("Validating for example query...")
+ if args.emb_model_type == "syntactic":
+ # reload with tfidf vocab
+ emb_model = TFIDFEmbeddings(
+ intel_scikit_learn_enabled=args.intel_scikit_learn_enabled,
+ tfidf_kwargs = {
+ "tfidf_vocab_path": save_path
+ }
+ )
+
+ vector_index = FAISS.load_local(
+ save_path, emb_model, normalize_L2=args.normalize_L2)
+ query = "How does a neural network help in predicting housing prices?"
+ docs = vector_index.similarity_search(query, k=3)
+ print("Relevant Docs: {}".format(docs))
+
+ print("*"*100)
+ print("😊 FAISS is a local vector index sucessfully saved with name faiss_emb_index")
+
+
+if __name__ == "__main__":
+
+ parser = argparse.ArgumentParser(description="Preprocess course video subtitle (.vtt) file")
+
+ parser.add_argument(
+ "--course_dir",
+ type=str,
+ help="Base directory containing courses",
+ required=True
+ )
+ parser.add_argument(
+ "--emb_model_type",
+ type=str,
+ default="syntactic",
+ help="Embedding model type as semantic or syntactic",
+ choices=["semantic", "syntactic"]
+ )
+ parser.add_argument(
+ "--model_name_or_path",
+ type=str,
+ default=None,
+ help="Hugging face model_name_or_path in case of emb_model_type as semantic"
+ )
+ parser.add_argument(
+ "--keep_accents",
+ action="store_true",
+ help="To preserve accents (vowel matras / diacritics) while tokenization in case of emb_model_type as semantic",
+ )
+ parser.add_argument(
+ "--intel_scikit_learn_enabled",
+ action="store_true",
+ help="Whether to use intel extension for scikit learn in case of emb_model_type as syntactic",
+ )
+ parser.add_argument(
+ "--ipex_enabled",
+ action="store_true",
+ help="Whether to use intel extension for pytorch in case emb_model_type as semantic",
+ )
+ parser.add_argument(
+ "--normalize_L2",
+ action="store_true",
+ help="Whether to normalize embedding, usually its a good idea in case of emb_model_type as syntactic",
+ )
+ parser.add_argument(
+ "--output_dir",
+ type=str,
+ default=None,
+ help="Output dir where index will be saved"
+ )
+
+ args = parser.parse_args()
+
+ # sanity checks
+ if args.emb_model_type == "semantic":
+ if args.model_name_or_path is None:
+ raise ValueError("Please provide valid `model_name_or_path` as emb_model_type = `semantic`")
+ elif args.emb_model_type == "syntactic":
+ if args.model_name_or_path is not None:
+ raise ValueError("Please don't provide `model_name_or_path` as emb_model_type = `syntatic`")
+
+ if args.output_dir is not None:
+ os.makedirs(args.output_dir, exist_ok=True)
+
+ main(args)
\ No newline at end of file
diff --git a/api/src/core/extractive_qa.py b/api/src/core/extractive_qa.py
new file mode 100644
index 000000000..f4e5ab9f3
--- /dev/null
+++ b/api/src/core/extractive_qa.py
@@ -0,0 +1,326 @@
+#!/usr/bin/env python
+# coding=utf-8
+# Copyright 2023 C5ailabs Team All rights reserved.
+#
+# Licensed under the Apache License, Version 2.0 (the "License");
+# you may not use this file except in compliance with the License.
+# You may obtain a copy of the License at
+#
+# http://www.apache.org/licenses/LICENSE-2.0
+#
+# Unless required by applicable law or agreed to in writing, software
+# distributed under the License is distributed on an "AS IS" BASIS,
+# WITHOUT WARRANTIES OR CONDITIONS OF ANY KIND, either express or implied.
+# See the License for the specific language governing permissions and
+# limitations under the License.
+"""
+Extractive Question Answering for LEAP platform
+"""
+from transformers import (
+ AutoConfig,
+ AutoModelForQuestionAnswering,
+ AutoTokenizer,
+ pipeline
+)
+# optimum-intel
+from optimum.intel import INCModelForQuestionAnswering
+
+# retrievers
+from core.retrievers.huggingface import HuggingFaceEmbeddings
+from core.retrievers.tfidf import TFIDFEmbeddings
+from core.retrievers.faiss_vector_store import FAISS
+
+from abc import ABC, abstractmethod
+from pydantic import Extra, BaseModel
+from typing import List, Optional, Dict, Any
+
+from utils.logging_handler import Logger
+
+class BaseQuestionAnswering(BaseModel, ABC):
+ """Base Question Answering interface"""
+
+ @abstractmethod
+ async def retrieve_docs(
+ self,
+ question: str,
+ top_n: Optional[int] = 2,
+ sim_score: Optional[float] = 0.9
+ ) -> List[str]:
+ """Take in a question and return List of top_n docs"""
+
+ @abstractmethod
+ async def span_answer(
+ self,
+ question: str,
+ context: List[str],
+ doc_stride: Optional[int] = 128,
+ max_answer_len: Optional[int] = 30,
+ max_seq_len: Optional[int] = 512,
+ top_k: Optional[int] = 1
+ ) -> Dict:
+ """Take in a question and context and return List of top_k answer as dict."""
+
+ @abstractmethod
+ async def predict(
+ self,
+ question: str,
+ doc_stride: Optional[int] = 128,
+ max_answer_length: Optional[int] = 30,
+ max_seq_length: Optional[int] = 512,
+ top_n: Optional[int] = 2,
+ top_k: Optional[int] = 1
+ ) -> Dict:
+ """Predict answer from question and context"""
+
+
+def load_qa_model(
+ model_name_or_path,
+ model_type="vanilla_fp32",
+ ipex_enabled=False,
+ model_revision="main",
+ keep_accents=False,
+ do_lower_case=False,
+ cache_dir=".cache"):
+ """load a QA model"""
+ qa_pipeline = None
+
+ tokenizer = AutoTokenizer.from_pretrained(
+ model_name_or_path,
+ cache_dir=cache_dir,
+ use_fast=True,
+ revision=model_revision,
+ keep_accents=keep_accents,
+ do_lower_case=do_lower_case,
+ )
+ if model_type == "vanilla_fp32":
+ config = AutoConfig.from_pretrained(
+ model_name_or_path,
+ cache_dir=cache_dir,
+ revision=model_revision
+ )
+ model = AutoModelForQuestionAnswering.from_pretrained(
+ model_name_or_path,
+ from_tf=bool(".ckpt" in model_name_or_path),
+ config=config,
+ cache_dir=cache_dir,
+ revision=model_revision
+ )
+ if ipex_enabled:
+ try:
+ import intel_extension_for_pytorch as ipex
+ except:
+ assert False, "transformers 4.29.0 requests IPEX version higher or equal to 1.12"
+ model = ipex.optimize(model)
+
+ qa_pipeline = pipeline(
+ task="question-answering",
+ model=model,
+ tokenizer=tokenizer
+ )
+ elif model_type == "quantized_int8":
+
+ model = INCModelForQuestionAnswering.from_pretrained(
+ model_name_or_path,
+ cache_dir=cache_dir,
+ revision=model_revision
+ )
+ qa_pipeline = pipeline(
+ task="question-answering",
+ model=model,
+ tokenizer=tokenizer
+ )
+
+ return qa_pipeline
+
+def load_emb_model(
+ model_name_or_path,
+ tfidf_vocab_path,
+ model_type="semantic",
+ intel_scikit_learn_enabled=True,
+ ipex_enabled=False,
+ model_revision="main",
+ keep_accents=False,
+ do_lower_case=False,
+ cache_dir=".cache"
+ ):
+ """load a Embedding model"""
+
+ emb_model = None
+
+ if model_type == "semantic":
+ emb_model = HuggingFaceEmbeddings(
+ model_name_or_path=model_name_or_path,
+ ipex_enabled=ipex_enabled,
+ hf_kwargs={
+ "model_revision": model_revision,
+ "keep_accents": keep_accents,
+ "do_lower_case": do_lower_case,
+ "cache_dir": cache_dir
+ }
+ )
+ elif model_type == "syntactic":
+ emb_model = TFIDFEmbeddings(
+ intel_scikit_learn_enabled=intel_scikit_learn_enabled,
+ tfidf_kwargs = {
+ "tfidf_vocab_path": tfidf_vocab_path
+ }
+ )
+
+ return emb_model
+
+def load_faiss_vector_index(
+ faiss_vector_index_path,
+ emb_model,
+ normalize_L2=False):
+
+ faiss_vector_index = FAISS.load_local(
+ faiss_vector_index_path, emb_model,
+ normalize_L2=normalize_L2)
+
+ return faiss_vector_index
+
+class ExtractiveQuestionAnswering(BaseQuestionAnswering):
+ """QuestionAnswering wrapper should take in a question and return a answer."""
+
+ emb_model: Any = None
+ emb_model_type: Optional[str] = "semantic"
+
+ qa_model: Any = None
+ qa_model_type: Optional[str] = "vanilla_fp32"
+
+ faiss_vector_index: Any = None
+
+ class Config:
+ """Configuration for this pydantic object."""
+
+ extra = Extra.forbid
+
+ @classmethod
+ def load(cls,
+ emb_model_name_or_path: str,
+ qa_model_name_or_path: str,
+ faiss_vector_index_path: str,
+ **kwargs):
+ """
+ Args:
+ emb_model_path (str): Embedding model path
+ qa_model_path (str): Question Answering model path
+ faiss_vector_index (str): Faiss Context vector index path
+ """
+
+ emb_model_type = kwargs.get("emb_model_type", "semantic")
+ qa_model_type = kwargs.get("qa_model_type", "vanilla_fp32")
+ normalize_L2 = kwargs.get("normalize_L2", False)
+
+ # load the emb model
+ emb_model = load_emb_model(
+ emb_model_name_or_path,
+ faiss_vector_index_path,
+ model_type=emb_model_type,
+ intel_scikit_learn_enabled=kwargs.get("intel_scikit_learn_enabled", True),
+ ipex_enabled=kwargs.get("ipex_enabled", False),
+ model_revision=kwargs.get("model_revision", "main"),
+ keep_accents=kwargs.get("keep_accents", False),
+ do_lower_case=kwargs.get("do_lower_case", False),
+ cache_dir=kwargs.get("cache_dir", ".cache")
+ )
+ # load the qa model
+ qa_model = load_qa_model(
+ qa_model_name_or_path,
+ model_type=qa_model_type,
+ ipex_enabled=kwargs.get("ipex_enabled", False),
+ model_revision=kwargs.get("model_revision", "main"),
+ keep_accents=kwargs.get("keep_accents", False),
+ do_lower_case=kwargs.get("do_lower_case", False),
+ cache_dir=kwargs.get("cache_dir", ".cache")
+ )
+
+ # load faiss vector index
+ faiss_vector_index = load_faiss_vector_index(
+ faiss_vector_index_path,
+ emb_model=emb_model,
+ normalize_L2=normalize_L2
+ )
+
+ return cls(
+ emb_model=emb_model,
+ emb_model_type=emb_model_type,
+ qa_model=qa_model,
+ qa_model_type=qa_model_type,
+ faiss_vector_index=faiss_vector_index
+ )
+
+ async def retrieve_docs(
+ self,
+ question: str,
+ top_n: Optional[int] = 2,
+ sim_score: Optional[float] = 0.9
+ ) -> List[str]:
+ """Take in a question and return List of top_n docs"""
+ docs = self.faiss_vector_index.similarity_search(
+ question, top_n,
+ sim_score=sim_score)
+
+ return docs
+
+ async def span_answer(
+ self,
+ question: str,
+ context: str,
+ doc_stride: Optional[int] = 128,
+ max_answer_length: Optional[int] = 30,
+ max_seq_length: Optional[int] = 512,
+ top_k: Optional[int] = 1
+ ) -> Dict:
+ """Take in a question and context and return List of top_k answer as dict."""
+
+ preds = self.qa_model(
+ question=question,
+ context=context,
+ doc_stride=doc_stride,
+ max_answer_len=max_answer_length,
+ max_seq_len=max_seq_length,
+ top_k=top_k
+ )
+ return preds
+
+ async def predict(
+ self,
+ question: str,
+ doc_stride: Optional[int] = 128,
+ max_answer_length: Optional[int] = 30,
+ max_seq_length: Optional[int] = 512,
+ top_n: Optional[int] = 2,
+ top_k: Optional[int] = 1
+ ) -> Dict:
+ """Predict answer from question and context"""
+
+ docs = await self.retrieve_docs(question, top_n)
+ relevant_contexts = [
+ {"context": doc.page_content, "metadata": doc.metadata}
+ for doc in docs
+ ]
+
+ contexts = [doc.page_content for doc in docs]
+ context = ". ".join(contexts)
+ preds = await self.span_answer(
+ question, context, doc_stride,
+ max_answer_length, max_seq_length, top_k)
+
+ relevant_context_id = -1
+ for index, dict_ in enumerate(relevant_contexts):
+ if preds["answer"] in dict_["context"]:
+ relevant_context_id = index
+ break
+
+ output = {
+ "question": question,
+ "context": context,
+ "answer": preds["answer"],
+ "score": preds["score"],
+ "relevant_context_id": relevant_context_id,
+ "relevant_contexts": relevant_contexts
+ }
+
+ return output
+
diff --git a/api/src/core/interactive_examiner.py b/api/src/core/interactive_examiner.py
new file mode 100644
index 000000000..14519a22f
--- /dev/null
+++ b/api/src/core/interactive_examiner.py
@@ -0,0 +1,238 @@
+#!/usr/bin/env python
+# coding=utf-8
+# Copyright 2023 C5ailabs Team (Authors: Rohit Sroch) All rights reserved.
+#
+# Licensed under the Apache License, Version 2.0 (the "License");
+# you may not use this file except in compliance with the License.
+# You may obtain a copy of the License at
+#
+# http://www.apache.org/licenses/LICENSE-2.0
+#
+# Unless required by applicable law or agreed to in writing, software
+# distributed under the License is distributed on an "AS IS" BASIS,
+# WITHOUT WARRANTIES OR CONDITIONS OF ANY KIND, either express or implied.
+# See the License for the specific language governing permissions and
+# limitations under the License.
+"""
+Intractive AI Examiner for LEAP platform
+"""
+#lang chain
+import langchain
+from langchain import PromptTemplate, LLMChain
+from langchain.cache import InMemoryCache
+
+from core.prompt import (
+ EXAMINER_ASK_QUESTION_PROMPT,
+ EXAMINER_EVALUATE_STUDENT_ANSWER_PROMPT,
+ EXAMINER_HINT_MOTIVATE_STUDENT_PROMPT
+)
+
+from abc import ABC, abstractmethod
+from pydantic import Extra, BaseModel
+from typing import List, Optional, Dict, Any
+
+from utils.logging_handler import Logger
+
+#langchain.llm_cache = InMemoryCache()
+
+class BaseAIExaminer(BaseModel, ABC):
+ """Base AI Examiner interface"""
+
+ @abstractmethod
+ async def examiner_ask_question(
+ self,
+ context: str,
+ question_type: str,
+ topic: str
+ ) -> Dict:
+ """AI Examiner generates a question"""
+
+ @abstractmethod
+ async def examiner_eval_answer(
+ self,
+ ai_question: str,
+ student_solution: str,
+ topic: str
+ ) -> Dict:
+ """AI Examiner evaluates student solution"""
+
+ @abstractmethod
+ async def examiner_hint_motivate(
+ self,
+ ai_question: str,
+ student_solution: str,
+ topic: str
+ ) -> Dict:
+ """AI Examiner hints and motivate student"""
+
+class InteractiveAIExaminer(BaseAIExaminer):
+ """Interactive AI Examiner"""
+
+ llm_chain_ask_ques: LLMChain
+ prompt_template_ask_ques: PromptTemplate
+
+ llm_chain_eval_ans: LLMChain
+ prompt_template_eval_ans: PromptTemplate
+
+ llm_chain_hint_motivate: LLMChain
+ prompt_template_hint_motivate: PromptTemplate
+
+ class Config:
+ """Configuration for this pydantic object."""
+
+ extra = Extra.forbid
+
+ @classmethod
+ def load(cls,
+ llm: Any,
+ **kwargs):
+ """
+ Args:
+ llm (str): Large language model object
+ """
+
+ verbose = kwargs.get("verbose", True)
+ # prompt templates
+ prompt_template_ask_ques = PromptTemplate(
+ template=EXAMINER_ASK_QUESTION_PROMPT,
+ input_variables=[
+ "context",
+ "question_type",
+ "topic"
+ ]
+ )
+ prompt_template_eval_ans = PromptTemplate(
+ template=EXAMINER_EVALUATE_STUDENT_ANSWER_PROMPT,
+ input_variables=[
+ "ai_question",
+ "student_solution",
+ "topic"
+ ]
+ )
+ prompt_template_hint_motivate = PromptTemplate(
+ template=EXAMINER_HINT_MOTIVATE_STUDENT_PROMPT,
+ input_variables=[
+ "ai_question",
+ "student_solution",
+ "topic"
+ ]
+ )
+ # llm chains
+ llm_chain_ask_ques = LLMChain(
+ llm=llm,
+ prompt=prompt_template_ask_ques,
+ verbose=verbose
+ )
+ llm_chain_eval_ans = LLMChain(
+ llm=llm,
+ prompt=prompt_template_eval_ans,
+ verbose=verbose
+ )
+ llm_chain_hint_motivate = LLMChain(
+ llm=llm,
+ prompt=prompt_template_hint_motivate,
+ verbose=verbose
+ )
+
+ return cls(
+ llm_chain_ask_ques=llm_chain_ask_ques,
+ prompt_template_ask_ques=prompt_template_ask_ques,
+ llm_chain_eval_ans=llm_chain_eval_ans,
+ prompt_template_eval_ans=prompt_template_eval_ans,
+ llm_chain_hint_motivate=llm_chain_hint_motivate,
+ prompt_template_hint_motivate=prompt_template_hint_motivate
+ )
+
+ async def examiner_ask_question(
+ self,
+ context: str,
+ question_type: str,
+ topic: str
+ ) -> Dict:
+ """AI Examiner generates a question"""
+ is_predicted = True
+ result = {
+ "prediction": None,
+ "error_message": None
+ }
+ try:
+ output = self.llm_chain_ask_ques.predict(
+ context=context,
+ question_type=question_type,
+ topic=topic)
+ output = output.strip()
+ result["prediction"] = {
+ "ai_question": output
+ }
+
+ return (is_predicted, result)
+ except Exception as err:
+ Logger.error("Error: {}".format(str(err)))
+ is_predicted = False
+ result["error_message"] = str(err)
+ return (is_predicted, result)
+
+ async def examiner_eval_answer(
+ self,
+ ai_question: str,
+ student_solution: str,
+ topic: str
+ ) -> Dict:
+ """AI Examiner evaluates student solution"""
+ is_predicted = True
+ result = {
+ "prediction": None,
+ "error_message": None
+ }
+ try:
+ output = self.llm_chain_eval_ans.predict(
+ ai_question=ai_question,
+ student_solution=student_solution,
+ topic=topic)
+ output = output.strip()
+ idx = output.find("Student grade:")
+ student_grade = output[idx + 15: ].strip()
+ result["prediction"] = {
+ "student_grade": student_grade
+ }
+
+ return (is_predicted, result)
+ except Exception as err:
+ Logger.error("Error: {}".format(str(err)))
+ is_predicted = False
+ result["error_message"] = str(err)
+ return (is_predicted, result)
+
+ async def examiner_hint_motivate(
+ self,
+ ai_question: str,
+ student_solution: str,
+ topic: str
+ ) -> Dict:
+ """AI Examiner hints and motivate student"""
+ is_predicted = True
+ result = {
+ "prediction": None,
+ "error_message": None
+ }
+ try:
+ output = self.llm_chain_hint_motivate.predict(
+ ai_question=ai_question,
+ student_solution=student_solution,
+ topic=topic)
+ output = output.strip()
+ idx = output.find("Encourage student:")
+ hint = output[: idx-1].strip()
+ motivation = output[idx + 19: ].strip()
+
+ result["prediction"] = {
+ "hint": hint,
+ "motivation": motivation
+ }
+
+ return (is_predicted, result)
+ except Exception as err:
+ Logger.error("Error: {}".format(str(err)))
+ is_predicted = False
+ result["error_message"] = str(err)
+ return (is_predicted, result)
\ No newline at end of file
diff --git a/api/src/core/llm/__init__.py b/api/src/core/llm/__init__.py
new file mode 100644
index 000000000..e69de29bb
diff --git a/api/src/core/llm/base.py b/api/src/core/llm/base.py
new file mode 100644
index 000000000..2eaa01d52
--- /dev/null
+++ b/api/src/core/llm/base.py
@@ -0,0 +1,65 @@
+
+# lang chain
+from langchain.callbacks.manager import CallbackManager
+from langchain.callbacks.streaming_stdout import StreamingStdOutCallbackHandler
+from langchain.llms import AzureOpenAI
+
+from core.llm.huggingface_pipeline import HuggingFacePipeline
+from core.llm.huggingface_peft import HuggingFacePEFT
+
+
+def get_llm(llm_method="azure_gpt3",
+ callback_manager=None,
+ **kwargs):
+
+ llm = None
+ if callback_manager is None:
+ callback_manager = CallbackManager(
+ [StreamingStdOutCallbackHandler()])
+ if llm_method == "azure_gpt3":
+ """
+ Wrapper around Azure OpenAI
+ https://azure.microsoft.com/en-in/products/cognitive-services/openai-service
+ """
+ llm_kwargs = kwargs.get("llm_kwargs", {})
+ llm = AzureOpenAI(
+ callback_manager=callback_manager,
+ deployment_name=kwargs.get(
+ "deployment_name", "text-davinci-003-prod"),
+ **llm_kwargs
+ )
+ elif llm_method == "hf_pipeline":
+ """
+ Wrapper around HuggingFace Pipeline API.
+ https://huggingface.co/models
+ """
+
+ llm = HuggingFacePipeline.from_model_id(
+ model_id=kwargs.get("model_name", "bigscience/bloom-1b7"),
+ task=kwargs.get("task", "text-generation"),
+ device=kwargs.get("device", -1),
+ model_kwargs=kwargs.get("llm_kwargs", {}),
+ pipeline_kwargs=kwargs.get("pipeline_kwargs", {}),
+ quantization_kwargs=kwargs.get("quantization_kwargs", {})
+ )
+ elif llm_method == "hf_peft":
+ """
+ Wrapper around HuggingFace Peft API.
+ https://huggingface.co/models
+ """
+ llm = HuggingFacePEFT.from_model_id(
+ model_id=kwargs.get("model_name", "huggyllama/llama-7b"),
+ adapter_id=kwargs.get("adapter_name", "timdettmers/qlora-flan-7b"),
+ task=kwargs.get("task", "text-generation"),
+ device=kwargs.get("device", -1),
+ model_kwargs=kwargs.get("llm_kwargs", {}),
+ generation_kwargs=kwargs.get("generation_kwargs", {}),
+ quantization_kwargs=kwargs.get("quantization_kwargs", {})
+ )
+ else:
+ raise ValueError(
+ "Please use a valid llm_name. Supported options are"
+ "[azure-gpt3, hf_pipeline, hf_peft] only."
+ )
+
+ return llm
diff --git a/api/src/core/llm/huggingface_peft.py b/api/src/core/llm/huggingface_peft.py
new file mode 100644
index 000000000..9c848e9ee
--- /dev/null
+++ b/api/src/core/llm/huggingface_peft.py
@@ -0,0 +1,226 @@
+"""Wrapper around HuggingFace Peft APIs."""
+import importlib.util
+import logging
+from typing import Any, List, Mapping, Optional
+
+from pydantic import Extra
+
+from langchain.callbacks.manager import CallbackManagerForLLMRun
+from langchain.llms.base import LLM
+from langchain.llms.utils import enforce_stop_tokens
+
+DEFAULT_MODEL_ID = "huggyllama/llama-7b"
+DEFAULT_ADAPTER_ID = "timdettmers/qlora-flan-7b"
+DEFAULT_TASK = "text-generation"
+VALID_TASKS = ("text-generation")
+
+logger = logging.getLogger(__name__)
+
+
+class HuggingFacePEFT(LLM):
+ """Wrapper around HuggingFace Pipeline API.
+
+ To use, you should have the ``transformers` and `peft`` python packages installed.
+
+ Only supports `text-generation` for now.
+
+ Example using from_model_id:
+ .. code-block:: python
+
+ from langchain.llms import HuggingFacePipeline
+ hf = HuggingFacePipeline.from_model_id(
+ model_id="gpt2",
+ task="text-generation",
+ pipeline_kwargs={"max_new_tokens": 10},
+ )
+ """
+
+ model: Any #: :meta private:
+ tokenizer: Any
+
+ device: int = -1
+ """Device to use"""
+ model_id: str = DEFAULT_MODEL_ID
+ """Model name to use."""
+ adapter_id: str = DEFAULT_ADAPTER_ID
+ """Adapter name to use"""
+ model_kwargs: Optional[dict] = None
+ """Key word arguments passed to the model."""
+ generation_kwargs: Optional[dict] = None
+ """Generation arguments passed to the model."""
+ quantization_kwargs: Optional[dict] = None
+ """Quantization arguments passed to the quantization."""
+
+ class Config:
+ """Configuration for this pydantic object."""
+
+ extra = Extra.forbid
+
+ @classmethod
+ def from_model_id(
+ cls,
+ model_id: str,
+ adapter_id: str,
+ task: str,
+ device: int = -1,
+ model_kwargs: Optional[dict] = {},
+ generation_kwargs: Optional[dict] = {},
+ quantization_kwargs: Optional[dict] = {}
+ ) -> LLM:
+ """Construct the pipeline object from model_id and task."""
+ try:
+ import torch
+ from transformers import (
+ AutoModelForCausalLM,
+ AutoModelForSeq2SeqLM,
+ AutoTokenizer,
+ BitsAndBytesConfig
+ )
+ from peft import PeftModel
+ except ImportError:
+ raise ValueError(
+ "Could not import transformers python package. "
+ "Please install it with `pip install transformers peft`."
+ )
+
+ _model_kwargs = model_kwargs or {}
+ tokenizer = AutoTokenizer.from_pretrained(model_id, **_model_kwargs)
+
+ try:
+ quantization_config = None
+ if quantization_kwargs:
+ bnb_4bit_compute_dtype = quantization_kwargs.get("bnb_4bit_compute_dtype", torch.float32)
+ if bnb_4bit_compute_dtype == "bfloat16":
+ quantization_kwargs["bnb_4bit_compute_dtype"] = torch.bfloat16
+ elif bnb_4bit_compute_dtype == "float16":
+ quantization_kwargs["bnb_4bit_compute_dtype"] = torch.float16
+ elif bnb_4bit_compute_dtype == "float32":
+ quantization_kwargs["bnb_4bit_compute_dtype"] = torch.float32
+
+ quantization_config = BitsAndBytesConfig(**quantization_kwargs)
+
+ torch_dtype = _model_kwargs.get("torch_dtype", torch.float32)
+ if torch_dtype is not None:
+ if torch_dtype == "bfloat16":
+ _model_kwargs["torch_dtype"] = torch.bfloat16
+ elif torch_dtype == "float16":
+ _model_kwargs["torch_dtype"] = torch.float16
+ elif torch_dtype == "float32":
+ _model_kwargs["torch_dtype"] = torch.float32
+
+
+ max_memory= {i: _model_kwargs.get(
+ "max_memory", "24000MB") for i in range(torch.cuda.device_count())}
+ _model_kwargs.pop("max_memory")
+ if task == "text-generation":
+ model = AutoModelForCausalLM.from_pretrained(
+ model_id,
+ max_memory=max_memory,
+ quantization_config=quantization_config,
+ **_model_kwargs
+ )
+ model = PeftModel.from_pretrained(model, adapter_id)
+ else:
+ raise ValueError(
+ f"Got invalid task {task}, "
+ f"currently only {VALID_TASKS} are supported"
+ )
+ except ImportError as e:
+ raise ValueError(
+ f"Could not load the {task} model due to missing dependencies."
+ ) from e
+
+ if importlib.util.find_spec("torch") is not None:
+
+ cuda_device_count = torch.cuda.device_count()
+ if device < -1 or (device >= cuda_device_count):
+ raise ValueError(
+ f"Got device=={device}, "
+ f"device is required to be within [-1, {cuda_device_count})"
+ )
+ if device < 0 and cuda_device_count > 0:
+ logger.warning(
+ "Device has %d GPUs available. "
+ "Provide device={deviceId} to `from_model_id` to use available"
+ "GPUs for execution. deviceId is -1 (default) for CPU and "
+ "can be a positive integer associated with CUDA device id.",
+ cuda_device_count,
+ )
+ if "trust_remote_code" in _model_kwargs:
+ _model_kwargs = {
+ k: v for k, v in _model_kwargs.items() if k != "trust_remote_code"
+ }
+
+ return cls(
+ model=model,
+ tokenizer=tokenizer,
+ device=device,
+ model_kwargs=model_kwargs,
+ generation_kwargs=generation_kwargs,
+ quantization_kwargs=quantization_kwargs
+ )
+
+ @property
+ def _identifying_params(self) -> Mapping[str, Any]:
+ """Get the identifying parameters."""
+ return {
+ "model_id": self.model_id,
+ "model_kwargs": self.model_kwargs,
+ "generation_kwargs": self.generation_kwargs,
+ "quantization_kwargs": self.quantization_kwargs,
+ }
+
+ @property
+ def _llm_type(self) -> str:
+ return "huggingface_peft"
+
+ def _call(
+ self,
+ prompt: str,
+ stop: Optional[List[str]] = None,
+ run_manager: Optional[CallbackManagerForLLMRun] = None,
+ **kwargs: Any,
+ ) -> str:
+ import torch
+ from transformers import GenerationConfig
+ from transformers import StoppingCriteria, StoppingCriteriaList
+
+ device_id = "cpu"
+ if self.device != -1:
+ device_id = "cuda:{}".format(self.device)
+
+ stopping_criteria = None
+ stop_sequence = self.generation_kwargs.get("stop_sequence", [])
+ if len(stop_sequence) > 0:
+ stop_token_ids = [self.tokenizer(
+ stop_word, add_special_tokens=False, return_tensors='pt')['input_ids'].squeeze() for stop_word in stop_sequence]
+ stop_token_ids = [token.to(device_id) for token in stop_token_ids]
+
+ # define custom stopping criteria object
+ class StopOnTokens(StoppingCriteria):
+ def __call__(self, input_ids: torch.LongTensor, scores: torch.FloatTensor, **kwargs) -> bool:
+ for stop_ids in stop_token_ids:
+ if torch.eq(input_ids[0][-len(stop_ids):], stop_ids).all():
+ return True
+ return False
+ stopping_criteria = StoppingCriteriaList([StopOnTokens()])
+
+ # eos_token_id = stop_token_ids[0][0].item()
+ # self.generation_kwargs["pad_token_id"] = 1
+ # self.generation_kwargs["eos_token_id"] = eos_token_id
+
+ self.generation_kwargs.pop("stop_sequence")
+ generation_config = GenerationConfig(**self.generation_kwargs)
+
+ inputs = self.tokenizer(prompt, return_tensors="pt").to(device_id)
+ outputs = self.model.generate(
+ inputs=inputs.input_ids,
+ stopping_criteria=stopping_criteria,
+ generation_config=generation_config
+ )
+ text = self.tokenizer.decode(outputs[0], skip_special_tokens=True)
+ text = text[len(prompt) :]
+ if len(stop_sequence) > 0:
+ text = enforce_stop_tokens(text, stop_sequence)
+
+ return text
\ No newline at end of file
diff --git a/api/src/core/llm/huggingface_pipeline.py b/api/src/core/llm/huggingface_pipeline.py
new file mode 100644
index 000000000..26d455a96
--- /dev/null
+++ b/api/src/core/llm/huggingface_pipeline.py
@@ -0,0 +1,251 @@
+"""Wrapper around HuggingFace Pipeline APIs."""
+import importlib.util
+import logging
+from typing import Any, List, Mapping, Optional
+
+from pydantic import Extra
+
+from langchain.callbacks.manager import CallbackManagerForLLMRun
+from langchain.llms.base import LLM
+from langchain.llms.utils import enforce_stop_tokens
+
+DEFAULT_MODEL_ID = "gpt2"
+DEFAULT_TASK = "text-generation"
+VALID_TASKS = ("text2text-generation", "text-generation", "summarization")
+
+logger = logging.getLogger(__name__)
+
+
+class HuggingFacePipeline(LLM):
+ """Wrapper around HuggingFace Pipeline API.
+
+ To use, you should have the ``transformers`` python package installed.
+
+ Only supports `text-generation`, `text2text-generation` and `summarization` for now.
+
+ Example using from_model_id:
+ .. code-block:: python
+
+ from langchain.llms import HuggingFacePipeline
+ hf = HuggingFacePipeline.from_model_id(
+ model_id="gpt2",
+ task="text-generation",
+ pipeline_kwargs={"max_new_tokens": 10},
+ )
+ Example passing pipeline in directly:
+ .. code-block:: python
+
+ from langchain.llms import HuggingFacePipeline
+ from transformers import AutoModelForCausalLM, AutoTokenizer, pipeline
+
+ model_id = "gpt2"
+ tokenizer = AutoTokenizer.from_pretrained(model_id)
+ model = AutoModelForCausalLM.from_pretrained(model_id)
+ pipe = pipeline(
+ "text-generation", model=model, tokenizer=tokenizer, max_new_tokens=10
+ )
+ hf = HuggingFacePipeline(pipeline=pipe)
+ """
+
+ pipeline: Any #: :meta private:
+ model_id: str = DEFAULT_MODEL_ID
+ """Model name to use."""
+ model_kwargs: Optional[dict] = None
+ """Key word arguments passed to the model."""
+ pipeline_kwargs: Optional[dict] = None
+ """Key word arguments passed to the pipeline."""
+ quantization_kwargs: Optional[dict] = None
+ """Quantization arguments passed to the quantization."""
+
+ class Config:
+ """Configuration for this pydantic object."""
+
+ extra = Extra.forbid
+
+ @classmethod
+ def from_model_id(
+ cls,
+ model_id: str,
+ task: str,
+ device: int = -1,
+ model_kwargs: Optional[dict] = {},
+ pipeline_kwargs: Optional[dict] = {},
+ quantization_kwargs: Optional[dict] = {},
+ **kwargs: Any,
+ ) -> LLM:
+ """Construct the pipeline object from model_id and task."""
+ try:
+ import torch
+ from transformers import (
+ AutoModelForCausalLM,
+ AutoModelForSeq2SeqLM,
+ AutoTokenizer,
+ BitsAndBytesConfig
+ )
+ from transformers import pipeline as hf_pipeline
+ from transformers import StoppingCriteria, StoppingCriteriaList
+
+ except ImportError:
+ raise ValueError(
+ "Could not import transformers python package. "
+ "Please install it with `pip install transformers`."
+ )
+
+ _model_kwargs = model_kwargs or {}
+ tokenizer = AutoTokenizer.from_pretrained(model_id, **_model_kwargs)
+
+ try:
+ quantization_config = None
+ if quantization_kwargs:
+ bnb_4bit_compute_dtype = quantization_kwargs.get("bnb_4bit_compute_dtype", torch.float32)
+ if bnb_4bit_compute_dtype == "bfloat16":
+ quantization_kwargs["bnb_4bit_compute_dtype"] = torch.bfloat16
+ elif bnb_4bit_compute_dtype == "float16":
+ quantization_kwargs["bnb_4bit_compute_dtype"] = torch.float16
+ elif bnb_4bit_compute_dtype == "float32":
+ quantization_kwargs["bnb_4bit_compute_dtype"] = torch.float32
+
+ quantization_config = BitsAndBytesConfig(**quantization_kwargs)
+
+ torch_dtype = _model_kwargs.get("torch_dtype", torch.float32)
+ if torch_dtype is not None:
+ if torch_dtype == "bfloat16":
+ _model_kwargs["torch_dtype"] = torch.bfloat16
+ elif torch_dtype == "float16":
+ _model_kwargs["torch_dtype"] = torch.float16
+ elif torch_dtype == "float32":
+ _model_kwargs["torch_dtype"] = torch.float32
+
+ max_memory= {i: _model_kwargs.get(
+ "max_memory", "32000MB") for i in range(torch.cuda.device_count())}
+ _model_kwargs.pop("max_memory")
+ if task == "text-generation":
+ model = AutoModelForCausalLM.from_pretrained(
+ model_id,
+ max_memory=max_memory,
+ quantization_config=quantization_config,
+ **_model_kwargs)
+ elif task in ("text2text-generation", "summarization"):
+ model = AutoModelForSeq2SeqLM.from_pretrained(
+ model_id,
+ max_memory=max_memory,
+ quantization_config=quantization_config,
+ **_model_kwargs)
+ else:
+ raise ValueError(
+ f"Got invalid task {task}, "
+ f"currently only {VALID_TASKS} are supported"
+ )
+ _model_kwargs.pop("torch_dtype")
+ except ImportError as e:
+ raise ValueError(
+ f"Could not load the {task} model due to missing dependencies."
+ ) from e
+
+ if importlib.util.find_spec("torch") is not None:
+
+ cuda_device_count = torch.cuda.device_count()
+ if device < -1 or (device >= cuda_device_count):
+ raise ValueError(
+ f"Got device=={device}, "
+ f"device is required to be within [-1, {cuda_device_count})"
+ )
+ if device < 0 and cuda_device_count > 0:
+ logger.warning(
+ "Device has %d GPUs available. "
+ "Provide device={deviceId} to `from_model_id` to use available"
+ "GPUs for execution. deviceId is -1 (default) for CPU and "
+ "can be a positive integer associated with CUDA device id.",
+ cuda_device_count,
+ )
+ if "trust_remote_code" in _model_kwargs:
+ _model_kwargs = {
+ k: v for k, v in _model_kwargs.items() if k != "trust_remote_code"
+ }
+ _pipeline_kwargs = pipeline_kwargs or {}
+
+ device_id = "cpu"
+ if device != -1:
+ device_id = "cuda:{}".format(device)
+
+ stopping_criteria = None
+ stop_sequence = _pipeline_kwargs.get("stop_sequence", [])
+ if len(stop_sequence) > 0:
+ stop_token_ids = [tokenizer(
+ stop_word, add_special_tokens=False, return_tensors='pt')['input_ids'].squeeze() for stop_word in stop_sequence]
+ stop_token_ids = [token.to(device_id) for token in stop_token_ids]
+
+ # define custom stopping criteria object
+ class StopOnTokens(StoppingCriteria):
+ def __call__(self, input_ids: torch.LongTensor, scores: torch.FloatTensor, **kwargs) -> bool:
+ for stop_ids in stop_token_ids:
+ if torch.eq(input_ids[0][-len(stop_ids):], stop_ids).all():
+ return True
+ return False
+ stopping_criteria = StoppingCriteriaList([StopOnTokens()])
+
+ # eos_token_id = stop_token_ids[0][0].item()
+ # self.generation_kwargs["pad_token_id"] = 1
+ # self.generation_kwargs["eos_token_id"] = eos_token_id
+
+ _pipeline_kwargs.pop("stop_sequence")
+ pipeline = hf_pipeline(
+ task=task,
+ model=model,
+ tokenizer=tokenizer,
+ device=device,
+ stopping_criteria=stopping_criteria,
+ model_kwargs=_model_kwargs,
+ **_pipeline_kwargs,
+ )
+ if pipeline.task not in VALID_TASKS:
+ raise ValueError(
+ f"Got invalid task {pipeline.task}, "
+ f"currently only {VALID_TASKS} are supported"
+ )
+ return cls(
+ pipeline=pipeline,
+ model_id=model_id,
+ model_kwargs=_model_kwargs,
+ pipeline_kwargs=_pipeline_kwargs,
+ **kwargs,
+ )
+
+ @property
+ def _identifying_params(self) -> Mapping[str, Any]:
+ """Get the identifying parameters."""
+ return {
+ "model_id": self.model_id,
+ "model_kwargs": self.model_kwargs,
+ "pipeline_kwargs": self.pipeline_kwargs,
+ }
+
+ @property
+ def _llm_type(self) -> str:
+ return "huggingface_pipeline"
+
+ def _call(
+ self,
+ prompt: str,
+ stop: Optional[List[str]] = None,
+ run_manager: Optional[CallbackManagerForLLMRun] = None,
+ **kwargs: Any,
+ ) -> str:
+ response = self.pipeline(prompt)
+ if self.pipeline.task == "text-generation":
+ # Text generation return includes the starter text.
+ text = response[0]["generated_text"][len(prompt) :]
+ elif self.pipeline.task == "text2text-generation":
+ text = response[0]["generated_text"]
+ elif self.pipeline.task == "summarization":
+ text = response[0]["summary_text"]
+ else:
+ raise ValueError(
+ f"Got invalid task {self.pipeline.task}, "
+ f"currently only {VALID_TASKS} are supported"
+ )
+ if stop is not None:
+ # This is a bit hacky, but I can't figure out a better way to enforce
+ # stop tokens when making calls to huggingface_hub.
+ text = enforce_stop_tokens(text, stop)
+ return text
\ No newline at end of file
diff --git a/api/src/core/prompt.py b/api/src/core/prompt.py
new file mode 100644
index 000000000..e346b1d35
--- /dev/null
+++ b/api/src/core/prompt.py
@@ -0,0 +1,64 @@
+EXAMINER_ASK_QUESTION_PROMPT="""You are an AI examiner, designed to ask a question in order to assess a student's knowledge and understanding around {topic} topic.
+Given a question type, your task is to generate a valid question you need to ask the student.
+
+Only use the following context around the topic of {topic}.
+
+CONTEXT
+=========
+{context}
+=========
+
+You MUST always only generate any one of the below types of questions:
+1. Open Ended: Ask a question for which the student needs to write an answer.
+2. Single Choice: Ask a question for which the student needs to choose a single option (max four options) as an answer.
+3. Multiple Choice: Ask a question for which the student needs to choose multiple options (max four options) as an answer.
+4. Yes or No Choice: Ask a question for which the student needs to choose yes or no as an answer.
+
+Please make sure you MUST always give an output in the following format:
+```
+Question Type: One of the above types of questions here
+Generated Question: A generated question of the provided type here
+```
+
+Be sure you MUST pay attention to the above context while generating the question and you MUST generate one of the valid types of question.
+
+Question Type: {question_type}
+Generated Question:
+"""
+
+EXAMINER_EVALUATE_STUDENT_ANSWER_PROMPT="""You are an AI examiner, designed to grade if the student's solution to question around topic {topic} is correct or not.
+Given a question and a corresponding student's solution, your task is to grade the student's solution.
+
+Only use the below guidelines to solve the problem:
+1. First, work out your own solution to the problem.
+2. Then compare your solution to the student's solution and evaluate if the student's solution is correct or not. Don't decide if the student's solution is correct until you have done the problem yourself.
+
+Please make sure you MUST always give an output in the following format:
+```
+Question: the question here
+Student's solution: the student's solution here
+Actual solution: the steps to work out the solution and your solution here
+Is the student's solution the same as the actual solution: Yes or No
+Student grade: Correct or Incorrect
+```
+
+Question: {ai_question}
+Student's solution: {student_solution}
+Actual solution:
+"""
+
+EXAMINER_HINT_MOTIVATE_STUDENT_PROMPT="""You are an AI examiner, designed to provide hints and motivate a student in a talkative and friendly manner so that students provide a correct solution to the question.
+Given a question around topic {topic} and a corresponding student's incorrect solution, your task is to provide a tiny hint and encourage the student.
+
+Please make sure you MUST always use the following format:
+```
+Question: the question here
+Student's incorrect solution: the student's incorrect solution here
+Tiny Hint: a tiny correct hint to help the student. you MUST never provide an actual solution
+Encourage student: encourage the student in a friendly manner. you MUST never provide an actual solution
+```
+
+Question: {ai_question}
+Student's incorrect solution: {student_solution}
+Tiny Hint:
+"""
\ No newline at end of file
diff --git a/api/src/core/retrievers/__init__.py b/api/src/core/retrievers/__init__.py
new file mode 100644
index 000000000..59bcacba1
--- /dev/null
+++ b/api/src/core/retrievers/__init__.py
@@ -0,0 +1,14 @@
+"""Wrappers around retrivers modules."""
+import logging
+
+from core.retrievers.huggingface import HuggingFaceEmbeddings
+from core.retrievers.tfidf import TFIDFEmbeddings
+
+logger = logging.getLogger(__name__)
+
+
+__all__ = [
+ "HuggingFaceEmbeddings",
+ "TFIDFEmbeddings"
+]
+
diff --git a/api/src/core/retrievers/base.py b/api/src/core/retrievers/base.py
new file mode 100644
index 000000000..9aba26f35
--- /dev/null
+++ b/api/src/core/retrievers/base.py
@@ -0,0 +1,14 @@
+"""Interface for embedding models."""
+from abc import ABC, abstractmethod
+from typing import List
+
+class Embeddings(ABC):
+ """Interface for embedding models."""
+
+ @abstractmethod
+ def embed_documents(self, texts: List[str]) -> List[List[float]]:
+ """Embed search docs."""
+
+ @abstractmethod
+ def embed_query(self, text: str) -> List[float]:
+ """Embed query text."""
diff --git a/api/src/core/retrievers/docstore.py b/api/src/core/retrievers/docstore.py
new file mode 100644
index 000000000..e8303af59
--- /dev/null
+++ b/api/src/core/retrievers/docstore.py
@@ -0,0 +1,52 @@
+"""Interface to access to place that stores documents."""
+
+from typing import Dict, Union
+
+from abc import ABC, abstractmethod
+from pydantic import BaseModel, Field
+
+
+class Document(BaseModel):
+ """Interface for interacting with a document."""
+
+ page_content: str
+ metadata: dict = Field(default_factory=dict)
+
+class Docstore(ABC):
+ """Interface to access to place that stores documents."""
+
+ @abstractmethod
+ def search(self, search: str) -> Union[str, Document]:
+ """Search for document.
+
+ If page exists, return the page summary, and a Document object.
+ If page does not exist, return similar entries.
+ """
+
+class AddableMixin(ABC):
+ """Mixin class that supports adding texts."""
+
+ @abstractmethod
+ def add(self, texts: Dict[str, Document]) -> None:
+ """Add more documents."""
+
+class InMemoryDocstore(Docstore, AddableMixin):
+ """Simple in memory docstore in the form of a dict."""
+
+ def __init__(self, _dict: Dict[str, Document]):
+ """Initialize with dict."""
+ self._dict = _dict
+
+ def add(self, texts: Dict[str, Document]) -> None:
+ """Add texts to in memory dictionary."""
+ overlapping = set(texts).intersection(self._dict)
+ if overlapping:
+ raise ValueError(f"Tried to add ids that already exist: {overlapping}")
+ self._dict = dict(self._dict, **texts)
+
+ def search(self, search: str) -> Union[str, Document]:
+ """Search via direct lookup."""
+ if search not in self._dict:
+ return f"ID {search} not found."
+ else:
+ return self._dict[search]
\ No newline at end of file
diff --git a/api/src/core/retrievers/faiss_vector_store.py b/api/src/core/retrievers/faiss_vector_store.py
new file mode 100644
index 000000000..197cd03c7
--- /dev/null
+++ b/api/src/core/retrievers/faiss_vector_store.py
@@ -0,0 +1,420 @@
+"""Interface to access to place that stores documents."""
+import os
+import pickle
+import uuid
+import math
+import numpy as np
+from pathlib import Path
+from typing import Any, Callable, Dict, Iterable, List, Optional, Tuple
+
+from core.retrievers.docstore import Document, Docstore, AddableMixin, InMemoryDocstore
+from core.retrievers.base import Embeddings
+
+def dependable_faiss_import(no_avx2: Optional[bool] = None) -> Any:
+ """
+ Import faiss if available, otherwise raise error.
+ If FAISS_NO_AVX2 environment variable is set, it will be considered
+ to load FAISS with no AVX2 optimization.
+
+ Args:
+ no_avx2: Load FAISS strictly with no AVX2 optimization
+ so that the vectorstore is portable and compatible with other devices.
+ """
+ if no_avx2 is None and "FAISS_NO_AVX2" in os.environ:
+ no_avx2 = bool(os.getenv("FAISS_NO_AVX2"))
+
+ try:
+ if no_avx2:
+ from faiss import swigfaiss as faiss
+ else:
+ import faiss
+ except ImportError:
+ raise ValueError(
+ "Could not import faiss python package. "
+ "Please install it with `pip install faiss` "
+ "or `pip install faiss-cpu` (depending on Python version)."
+ )
+ return faiss
+
+def _default_relevance_score_fn(score: float) -> float:
+ """Return a similarity score on a scale [0, 1]."""
+ # The 'correct' relevance function
+ # may differ depending on a few things, including:
+ # - the distance / similarity metric used by the VectorStore
+ # - the scale of your embeddings (OpenAI's are unit normed. Many others are not!)
+ # - embedding dimensionality
+ # - etc.
+ # This function converts the euclidean norm of normalized embeddings
+ # (0 is most similar, sqrt(2) most dissimilar)
+ # to a similarity function (0 to 1)
+ return 1.0 - score / math.sqrt(2)
+
+class FAISS:
+ """Wrapper around FAISS vector database.
+
+ To use, you should have the ``faiss`` python package installed.
+
+ Example:
+ .. code-block:: python
+
+ from langchain import FAISS
+ faiss = FAISS(embedding_function, index, docstore, index_to_docstore_id)
+
+ """
+
+ def __init__(
+ self,
+ embedding_function: Callable,
+ index: Any,
+ docstore: Docstore,
+ index_to_docstore_id: Dict[int, str],
+ normalize_L2: bool = False,
+ relevance_score_fn: Optional[
+ Callable[[float], float]
+ ] = _default_relevance_score_fn,
+ ):
+ """Initialize with necessary components."""
+ self.embedding_function = embedding_function
+ self.index = index
+ self.docstore = docstore
+ self.index_to_docstore_id = index_to_docstore_id
+ self._normalize_L2 = normalize_L2
+ self.relevance_score_fn=relevance_score_fn
+
+ def __add(
+ self,
+ texts: Iterable[str],
+ embeddings: Iterable[List[float]],
+ metadatas: Optional[List[dict]] = None,
+ ids: Optional[List[str]] = None,
+ **kwargs: Any,
+ ) -> List[str]:
+ if not isinstance(self.docstore, AddableMixin):
+ raise ValueError(
+ "If trying to add texts, the underlying docstore should support "
+ f"adding items, which {self.docstore} does not"
+ )
+ documents = []
+ for i, text in enumerate(texts):
+ metadata = metadatas[i] if metadatas else {}
+ documents.append(Document(page_content=text, metadata=metadata))
+ if ids is None:
+ ids = [str(uuid.uuid4()) for _ in texts]
+ # Add to the index, the index_to_id mapping, and the docstore.
+ starting_len = len(self.index_to_docstore_id)
+ faiss = dependable_faiss_import()
+ vector = np.array(embeddings, dtype=np.float32)
+ if self._normalize_L2:
+ faiss.normalize_L2(vector)
+ self.index.add(vector)
+ # Get list of index, id, and docs.
+ full_info = [(starting_len + i, ids[i], doc) for i, doc in enumerate(documents)]
+ # Add information to docstore and index.
+ self.docstore.add({_id: doc for _, _id, doc in full_info})
+ index_to_id = {index: _id for index, _id, _ in full_info}
+ self.index_to_docstore_id.update(index_to_id)
+ return [_id for _, _id, _ in full_info]
+
+ def add_texts(
+ self,
+ texts: Iterable[str],
+ metadatas: Optional[List[dict]] = None,
+ ids: Optional[List[str]] = None,
+ **kwargs: Any,
+ ) -> List[str]:
+ """Run more texts through the embeddings and add to the vectorstore.
+
+ Args:
+ texts: Iterable of strings to add to the vectorstore.
+ metadatas: Optional list of metadatas associated with the texts.
+ ids: Optional list of unique IDs.
+
+ Returns:
+ List of ids from adding the texts into the vectorstore.
+ """
+ if not isinstance(self.docstore, AddableMixin):
+ raise ValueError(
+ "If trying to add texts, the underlying docstore should support "
+ f"adding items, which {self.docstore} does not"
+ )
+ # Embed and create the documents.
+ embeddings = [self.embedding_function(text) for text in texts]
+ return self.__add(texts, embeddings, metadatas=metadatas, ids=ids, **kwargs)
+
+ def add_embeddings(
+ self,
+ text_embeddings: Iterable[Tuple[str, List[float]]],
+ metadatas: Optional[List[dict]] = None,
+ ids: Optional[List[str]] = None,
+ **kwargs: Any,
+ ) -> List[str]:
+ """Run more texts through the embeddings and add to the vectorstore.
+
+ Args:
+ text_embeddings: Iterable pairs of string and embedding to
+ add to the vectorstore.
+ metadatas: Optional list of metadatas associated with the texts.
+ ids: Optional list of unique IDs.
+
+ Returns:
+ List of ids from adding the texts into the vectorstore.
+ """
+ if not isinstance(self.docstore, AddableMixin):
+ raise ValueError(
+ "If trying to add texts, the underlying docstore should support "
+ f"adding items, which {self.docstore} does not"
+ )
+ # Embed and create the documents.
+ texts, embeddings = zip(*text_embeddings)
+
+ return self.__add(texts, embeddings, metadatas=metadatas, ids=ids, **kwargs)
+
+ def similarity_search_with_score_by_vector(
+ self, embedding: List[float], k: int = 4
+ ) -> List[Tuple[Document, float]]:
+ """Return docs most similar to query.
+
+ Args:
+ embedding: Embedding vector to look up documents similar to.
+ k: Number of Documents to return. Defaults to 4.
+
+ Returns:
+ List of Documents most similar to the query and score for each
+ """
+ faiss = dependable_faiss_import()
+ vector = np.array([embedding], dtype=np.float32)
+ if self._normalize_L2:
+ faiss.normalize_L2(vector)
+ scores, indices = self.index.search(vector, k)
+ docs = []
+ for j, i in enumerate(indices[0]):
+ if i == -1:
+ # This happens when not enough docs are returned.
+ continue
+ _id = self.index_to_docstore_id[i]
+ doc = self.docstore.search(_id)
+ if not isinstance(doc, Document):
+ raise ValueError(f"Could not find document for id {_id}, got {doc}")
+ docs.append((doc, scores[0][j]))
+ return docs
+
+ def similarity_search_with_score(
+ self, query: str, k: int = 4
+ ) -> List[Tuple[Document, float]]:
+ """Return docs most similar to query.
+
+ Args:
+ query: Text to look up documents similar to.
+ k: Number of Documents to return. Defaults to 4.
+
+ Returns:
+ List of Documents most similar to the query and score for each
+ """
+ embedding = self.embedding_function(query)
+ docs = self.similarity_search_with_score_by_vector(embedding, k)
+ return docs
+
+ def similarity_search_by_vector(
+ self, embedding: List[float], k: int = 4, **kwargs: Any
+ ) -> List[Document]:
+ """Return docs most similar to embedding vector.
+
+ Args:
+ embedding: Embedding to look up documents similar to.
+ k: Number of Documents to return. Defaults to 4.
+
+ Returns:
+ List of Documents most similar to the embedding.
+ """
+ docs_and_scores = self.similarity_search_with_score_by_vector(embedding, k)
+ docs_and_scores = sorted(docs_and_scores, key=lambda x: x[1], reverse=True)
+ return [doc for doc, _ in docs_and_scores]
+
+ def similarity_search(
+ self, query: str, k: int = 4, **kwargs: Any
+ ) -> List[Document]:
+ """Return docs most similar to query.
+
+ Args:
+ query: Text to look up documents similar to.
+ k: Number of Documents to return. Defaults to 4.
+
+ Returns:
+ List of Documents most similar to the query.
+ """
+ docs_and_scores = self.similarity_search_with_score(query, k)
+ docs_and_scores = sorted(docs_and_scores, key=lambda x: x[1], reverse=True)
+ return [doc for doc, _ in docs_and_scores]
+
+ def similarity_search_with_relevance_scores(
+ self,
+ query: str,
+ k: int = 4,
+ **kwargs: Any,
+ ) -> List[Tuple[Document, float]]:
+ """Return docs and their similarity scores on a scale from 0 to 1."""
+ if self.relevance_score_fn is None:
+ raise ValueError(
+ "normalize_score_fn must be provided to"
+ " FAISS constructor to normalize scores"
+ )
+ docs_and_scores = self.similarity_search_with_score(query, k=k)
+ return [(doc, self.relevance_score_fn(score)) for doc, score in docs_and_scores]
+
+ @classmethod
+ def __from(
+ cls,
+ texts: List[str],
+ embeddings: List[List[float]],
+ embedding: Embeddings,
+ metadatas: Optional[List[dict]] = None,
+ ids: Optional[List[str]] = None,
+ normalize_L2: bool = False,
+ **kwargs: Any,
+ ) -> Any:
+ faiss = dependable_faiss_import()
+ index = faiss.IndexFlatL2(len(embeddings[0]))
+ vector = np.array(embeddings, dtype=np.float32)
+ if normalize_L2:
+ faiss.normalize_L2(vector)
+ index.add(vector)
+ documents = []
+ if ids is None:
+ ids = [str(uuid.uuid4()) for _ in texts]
+ for i, text in enumerate(texts):
+ metadata = metadatas[i] if metadatas else {}
+ documents.append(Document(page_content=text, metadata=metadata))
+ index_to_id = dict(enumerate(ids))
+ docstore = InMemoryDocstore(dict(zip(index_to_id.values(), documents)))
+ return cls(
+ embedding.embed_query,
+ index,
+ docstore,
+ index_to_id,
+ normalize_L2=normalize_L2,
+ **kwargs,
+ )
+
+ @classmethod
+ def from_texts(
+ cls,
+ texts: List[str],
+ embedding: Embeddings,
+ metadatas: Optional[List[dict]] = None,
+ ids: Optional[List[str]] = None,
+ **kwargs: Any,
+ ) -> Any:
+ """Construct FAISS wrapper from raw documents.
+
+ This is a user friendly interface that:
+ 1. Embeds documents.
+ 2. Creates an in memory docstore
+ 3. Initializes the FAISS database
+ """
+ embeddings = embedding.embed_documents(texts)
+ return cls.__from(
+ texts,
+ embeddings,
+ embedding,
+ metadatas=metadatas,
+ ids=ids,
+ **kwargs,
+ )
+
+ @classmethod
+ def from_embeddings(
+ cls,
+ text_embeddings: List[Tuple[str, List[float]]],
+ embedding: Embeddings,
+ metadatas: Optional[List[dict]] = None,
+ ids: Optional[List[str]] = None,
+ **kwargs: Any,
+ ) -> Any:
+ """Construct FAISS wrapper from raw documents.
+
+ This is a user friendly interface that:
+ 1. Embeds documents.
+ 2. Creates an in memory docstore
+ 3. Initializes the FAISS database
+
+ This is intended to be a quick way to get started.
+
+ Example:
+ .. code-block:: python
+
+ from langchain import FAISS
+ from langchain.embeddings import OpenAIEmbeddings
+ embeddings = OpenAIEmbeddings()
+ text_embeddings = embeddings.embed_documents(texts)
+ text_embedding_pairs = list(zip(texts, text_embeddings))
+ faiss = FAISS.from_embeddings(text_embedding_pairs, embeddings)
+ """
+ texts = [t[0] for t in text_embeddings]
+ embeddings = [t[1] for t in text_embeddings]
+ return cls.__from(
+ texts,
+ embeddings,
+ embedding,
+ metadatas=metadatas,
+ ids=ids,
+ **kwargs,
+ )
+
+ @classmethod
+ def from_documents(
+ cls,
+ documents: List[Document],
+ embedding: Embeddings,
+ **kwargs: Any,
+ ) -> Any:
+ """Return VectorStore initialized from documents and embeddings."""
+ texts = [d.page_content for d in documents]
+ metadatas = [d.metadata for d in documents]
+ return cls.from_texts(texts, embedding, metadatas=metadatas, **kwargs)
+
+ def save_local(self, folder_path: str, index_name: str = "index") -> None:
+ """Save FAISS index, docstore, and index_to_docstore_id to disk.
+
+ Args:
+ folder_path: folder path to save index, docstore,
+ and index_to_docstore_id to.
+ index_name: for saving with a specific index file name
+ """
+ path = Path(folder_path)
+ path.mkdir(exist_ok=True, parents=True)
+
+ # save index separately since it is not picklable
+ faiss = dependable_faiss_import()
+ faiss.write_index(
+ self.index, str(path / "{index_name}.faiss".format(index_name=index_name))
+ )
+
+ # save docstore and index_to_docstore_id
+ with open(path / "{index_name}.pkl".format(index_name=index_name), "wb") as f:
+ pickle.dump((self.docstore, self.index_to_docstore_id), f)
+
+ @classmethod
+ def load_local(
+ cls, folder_path: str, embeddings: Embeddings, index_name: str = "index",
+ normalize_L2: bool = False
+ ) -> Any:
+ """Load FAISS index, docstore, and index_to_docstore_id from disk.
+
+ Args:
+ folder_path: folder path to load index, docstore,
+ and index_to_docstore_id from.
+ embeddings: Embeddings to use when generating queries
+ index_name: for saving with a specific index file name
+ """
+ path = Path(folder_path)
+ # load index separately since it is not picklable
+ faiss = dependable_faiss_import()
+ index = faiss.read_index(
+ str(path / "{index_name}.faiss".format(index_name=index_name))
+ )
+
+ # load docstore and index_to_docstore_id
+ with open(path / "{index_name}.pkl".format(index_name=index_name), "rb") as f:
+ docstore, index_to_docstore_id = pickle.load(f)
+ return cls(embeddings.embed_query, index, docstore, index_to_docstore_id, normalize_L2=normalize_L2)
+
diff --git a/api/src/core/retrievers/huggingface.py b/api/src/core/retrievers/huggingface.py
new file mode 100644
index 000000000..f572b3615
--- /dev/null
+++ b/api/src/core/retrievers/huggingface.py
@@ -0,0 +1,101 @@
+"""Wrapper around HuggingFace embedding models."""
+from __future__ import annotations
+
+
+from typing import Any, Dict, List, Optional
+from pydantic import BaseModel, Extra, Field
+from core.retrievers.base import Embeddings
+
+class HuggingFaceEmbeddings(BaseModel, Embeddings):
+ """Wrapper around huggingface transformers feature-extraction pipeline."""
+
+ emb_pipeline: Any #: :meta private:
+
+ model_name_or_path: str = None
+ ipex_enabled: Optional[bool] = False
+ """Key word arguments to pass to the model."""
+ hf_kwargs: Dict[str, Any] = Field(default_factory=dict)
+
+ def __init__(self, **kwargs: Any):
+ """Initialize the sentence_transformer."""
+ super().__init__(**kwargs)
+ try:
+ from transformers import (
+ AutoConfig,
+ AutoModel,
+ AutoTokenizer,
+ pipeline
+ )
+
+ except ImportError as exc:
+ raise ImportError(
+ "Could not import transformers python package. "
+ "Please install it with `pip install transformers`."
+ ) from exc
+
+ tokenizer = AutoTokenizer.from_pretrained(
+ self.model_name_or_path,
+ cache_dir=self.hf_kwargs.get("cache_dir", ".cache"),
+ use_fast=True,
+ revision=self.hf_kwargs.get("model_revision", None),
+ keep_accents=self.hf_kwargs.get("keep_accents", False),
+ do_lower_case=self.hf_kwargs.get("do_lower_case", False)
+ )
+ config = AutoConfig.from_pretrained(
+ self.model_name_or_path,
+ cache_dir=self.hf_kwargs.get("cache_dir", ".cache"),
+ revision=self.hf_kwargs.get("model_revision", None),
+ )
+ model = AutoModel.from_pretrained(
+ self.model_name_or_path,
+ from_tf=bool(".ckpt" in self.model_name_or_path),
+ config=config,
+ cache_dir=self.hf_kwargs.get("cache_dir", ".cache"),
+ revision=self.hf_kwargs.get("model_revision", None)
+ )
+
+ if self.ipex_enabled:
+ try:
+ import intel_extension_for_pytorch as ipex
+ except:
+ assert False, "transformers 4.29.0 requests IPEX version higher or equal to 1.12"
+ model = ipex.optimize(model)
+
+ self.emb_pipeline = pipeline(
+ task="feature-extraction",
+ model=model,
+ tokenizer=tokenizer
+ )
+
+ class Config:
+ """Configuration for this pydantic object."""
+
+ extra = Extra.forbid
+
+ def embed_documents(self, texts: List[str]) -> List[List[float]]:
+ """Compute doc embeddings using a HuggingFace transformer model.
+
+ Args:
+ texts: The list of texts to embed.
+
+ Returns:
+ List of embeddings, one for each text.
+ """
+ texts = list(map(lambda x: x.replace("\n", " "), texts))
+ embeddings = self.emb_pipeline(texts, return_tensors = "pt")
+ embeddings = [emb[0].numpy().mean(axis=0).tolist() for emb in embeddings]
+
+ return embeddings
+
+ def embed_query(self, text: str) -> List[float]:
+ """Compute query embeddings using a HuggingFace transformer model.
+
+ Args:
+ text: The text to embed.
+
+ Returns:
+ Embeddings for the text.
+ """
+ text = text.replace("\n", " ")
+ embedding = self.emb_pipeline(text, return_tensors = "pt")[0].numpy().mean(axis=0)
+ return embedding.tolist()
\ No newline at end of file
diff --git a/api/src/core/retrievers/tfidf.py b/api/src/core/retrievers/tfidf.py
new file mode 100644
index 000000000..d1c1a17c5
--- /dev/null
+++ b/api/src/core/retrievers/tfidf.py
@@ -0,0 +1,132 @@
+"""Wrapper around sklearn TF-IDF vectorizer"""
+from __future__ import annotations
+
+import os
+import pickle
+from collections import defaultdict
+
+from typing import Any, Dict, List, Optional
+from pydantic import BaseModel, Extra, Field
+from core.retrievers.base import Embeddings
+
+try:
+ from nltk.tokenize import word_tokenize
+ from nltk import pos_tag
+ from nltk.corpus import stopwords
+ from nltk.stem import WordNetLemmatizer
+ from nltk.corpus import wordnet as wn
+except ImportError as exc:
+ raise ImportError(
+ "Could not import nltk python package. "
+ "Please install it with `pip install nltk`."
+ ) from exc
+
+
+class TFIDFEmbeddings(BaseModel, Embeddings):
+ """Wrapper around sklearn TFIDF vectorizer."""
+
+ vectorizer: Any #: :meta private:
+
+ intel_scikit_learn_enabled: Optional[bool] = True
+ """Key word arguments to pass to the model."""
+ tfidf_kwargs: Dict[str, Any] = Field(default_factory=dict)
+
+ def __init__(self, **kwargs: Any):
+ """Initialize the sentence_transformer."""
+ super().__init__(**kwargs)
+ try:
+ if self.intel_scikit_learn_enabled:
+ # Turn on scikit-learn optimizations with these 2 simple lines:
+ from sklearnex import patch_sklearn
+ patch_sklearn()
+
+ from sklearn.feature_extraction.text import TfidfVectorizer
+ except ImportError as exc:
+ raise ImportError(
+ "Could not import scikit-learn and scikit-learn-intelex python package. "
+ "Please install it with `pip install scikit-learn scikit-learn-intelex`."
+ ) from exc
+
+ if self.tfidf_kwargs.get("tfidf_vocab_path", None) is not None:
+ print("******Loading tfidf_vocab.pkl ********")
+ path = os.path.join(self.tfidf_kwargs.get("tfidf_vocab_path"), "tfidf_vocab.pkl")
+
+ with open(path, "rb") as fp:
+ tfidf_vocab = pickle.load(fp)
+ self.tfidf_kwargs["vocabulary"] = tfidf_vocab
+ self.tfidf_kwargs.pop("tfidf_vocab_path")
+
+ self.vectorizer = TfidfVectorizer(**self.tfidf_kwargs)
+
+ class Config:
+ """Configuration for this pydantic object."""
+
+ arbitrary_types_allowed = True
+
+ def save_tfidf_vocab(self, tfidf_vocab, save_path):
+ """save the tfidf vectorizer object"""
+
+ path = os.path.join(save_path, "tfidf_vocab.pkl")
+ with open(path, "wb") as f:
+ pickle.dump(tfidf_vocab, f)
+
+ def embed_documents(self, texts: List[str], is_preprocess: bool=False) -> List[List[float]]:
+ """Compute doc embeddings using a HuggingFace transformer model.
+
+ Args:
+ texts: The list of texts to embed.
+
+ Returns:
+ List of embeddings, one for each text.
+ """
+ if is_preprocess:
+ texts = list(map(lambda x: self._preprocess_query(x.replace("\n", " ")), texts))
+ else:
+ texts = list(map(lambda x: x.replace("\n", " "), texts))
+ embeddings = self.vectorizer.fit_transform(texts)
+ embeddings = [emb.toarray().astype("float32")[0].tolist() for emb in embeddings]
+
+ return embeddings
+
+ def embed_query(self, text: str, is_preprocess: bool=False) -> List[float]:
+ """Compute query embeddings using a HuggingFace transformer model.
+
+ Args:
+ text: The text to embed.
+
+ Returns:
+ Embeddings for the text.
+ """
+ if is_preprocess:
+ text = self._preprocess_query(text.replace("\n", " "))
+ else:
+ text = text.replace("\n", " ")
+ embedding = self.vectorizer.fit_transform([text]).toarray().astype("float32")[0]
+ return embedding.tolist()
+
+ def _preprocess_query(self, query):
+ """preprocess the query"""
+
+ # Next change is lower case using apply and lambda function
+ query_transformed = word_tokenize(query)
+ # Now to remove stopwords, lemmatisation and stemming
+ # We need p.o.s (part of speech) tags to understand if its a noun or a verb
+ tag_map = defaultdict(lambda: wn.NOUN)
+ tag_map['J'] = wn.ADJ
+ tag_map['V'] = wn.VERB
+ tag_map['R'] = wn.ADV
+
+ _stopwords = stopwords.words('english')
+ _query = ""
+ # Instantiate the lemmatizer
+ word_lem = WordNetLemmatizer()
+ for word, tag in pos_tag(query_transformed):
+ # Loop over the entry in the text column.
+ # If the word is not in the stopword
+ if word not in _stopwords and (word.isalpha() or word.isalnum() or word.isnumeric()):
+ # Run our lemmatizer on the word.
+ word = str(word_lem.lemmatize(word, tag_map[tag[0]]))
+ # Now add to final words
+ _query += word + " "
+
+ return _query.strip()
\ No newline at end of file
diff --git a/api/src/main.py b/api/src/main.py
new file mode 100644
index 000000000..d994a9fef
--- /dev/null
+++ b/api/src/main.py
@@ -0,0 +1,50 @@
+"""
+LEAP API
+"""
+import config
+import uvicorn
+from fastapi import FastAPI, Request, status
+from fastapi.exceptions import RequestValidationError
+from fastapi.encoders import jsonable_encoder
+from fastapi.middleware.cors import CORSMiddleware
+from routes import ask_doubt, ai_examiner
+
+app = FastAPI()
+
+@app.get("/ping")
+def health_check():
+ """Health Check API
+ Returns:
+ dict: Status object with success message
+ """
+ return {
+ "success": True,
+ "message": f"Successfully reached LEAP API",
+ "data": {}
+ }
+
+origins = ["*"] # specify orgins to handle CORS issue
+app.add_middleware(
+ CORSMiddleware,
+ allow_origins=origins,
+ allow_credentials=True,
+ allow_methods=["*"],
+ allow_headers=["*"],
+)
+
+api_v1 = FastAPI()
+
+api_v1.include_router(ask_doubt.router)
+api_v1.include_router(ai_examiner.router)
+
+app.mount(f"/leap/api/v1", api_v1)
+
+# if __name__ == "__main__":
+
+# uvicorn.run(
+# "main:app",
+# host="0.0.0.0",
+# port=int(config.PORT),
+# log_level="info",
+# workers=1,
+# reload=True)
\ No newline at end of file
diff --git a/api/src/routes/__init__.py b/api/src/routes/__init__.py
new file mode 100644
index 000000000..e69de29bb
diff --git a/api/src/routes/ai_examiner.py b/api/src/routes/ai_examiner.py
new file mode 100644
index 000000000..3cd372f87
--- /dev/null
+++ b/api/src/routes/ai_examiner.py
@@ -0,0 +1,169 @@
+""" AI Examiner endpoints """
+import time
+from fastapi import APIRouter
+from fastapi.responses import JSONResponse
+from schemas.error import NotFoundErrorResponseModel, InternalServerErrorResponseModel
+from schemas.ai_examiner import (
+ AIExaminerAskQuestionModel,
+ AIExaminerEvalAnswerModel,
+ AIExaminerHintMotivateModel
+)
+
+from config import AI_EXAMINER_CONFIG
+from core.interactive_examiner import InteractiveAIExaminer
+from core.llm.base import get_llm
+
+from utils.logging_handler import Logger
+
+router = APIRouter(
+ tags=["AI Examiner"],
+ responses={500: {
+ "model": InternalServerErrorResponseModel
+ }})
+
+##################################################################
+LLM_METHOD = AI_EXAMINER_CONFIG["llm_method"]
+LLM_CONFIG = AI_EXAMINER_CONFIG[LLM_METHOD]
+llm = get_llm(llm_method=LLM_METHOD, **LLM_CONFIG)
+interactive_ai_examiner = InteractiveAIExaminer.load(
+ llm=llm,
+ verbose=True
+)
+
+@router.post("/ai-examiner/ask-question",
+ responses={404: {
+ "model": NotFoundErrorResponseModel
+ }})
+async def create_examiner_ask_question(
+ input_payload: AIExaminerAskQuestionModel):
+ """Analyze job
+ Args:
+ input_payload (AIExaminerAskQuestionModel)
+ Raises:
+ Exception: 500 Internal Server Error if something went wrong
+ Returns:
+ JSON: Success/Fail Message
+ """
+ try:
+ input_payload_dict = {**input_payload.dict()}
+
+ context = input_payload_dict["context"]
+ question_type = input_payload_dict["question_type"]
+ topic = input_payload_dict["topic"]
+
+ Logger.info("Input question type: {} around topic {}".format(question_type, topic))
+ start_time = time.time()
+ is_predicted, output = await interactive_ai_examiner.examiner_ask_question(
+ context=context,
+ question_type=question_type,
+ topic=topic
+ )
+ end_time = time.time()
+
+ Logger.info("🕒 Time taken to get response: {} sec".format(end_time-start_time))
+ if is_predicted:
+ output.pop("error_message")
+ response = {
+ "success": True,
+ "message": "Successfully predicted the response",
+ "data": output
+ }
+ return JSONResponse(status_code=200, content=response)
+ else:
+ response = {"success": False, "message": output["error_message"], "data": {}}
+ return JSONResponse(status_code=500, content=response)
+ except Exception as e:
+ response = {"success": False, "message": str(e), "data": {}}
+ return JSONResponse(status_code=500, content=response)
+
+
+@router.post("/ai-examiner/eval-answer",
+ responses={404: {
+ "model": NotFoundErrorResponseModel
+ }})
+async def create_examiner_eval_answer(
+ input_payload: AIExaminerEvalAnswerModel):
+ """Analyze job
+ Args:
+ input_payload (AIExaminerEvalAnswerModel)
+ Raises:
+ Exception: 500 Internal Server Error if something went wrong
+ Returns:
+ JSON: Success/Fail Message
+ """
+ try:
+ input_payload_dict = {**input_payload.dict()}
+
+ ai_question = input_payload_dict["ai_question"]
+ student_solution = input_payload_dict["student_solution"]
+ topic = input_payload_dict["topic"]
+
+ start_time = time.time()
+ is_predicted, output = await interactive_ai_examiner.examiner_eval_answer(
+ ai_question=ai_question,
+ student_solution=student_solution,
+ topic=topic
+ )
+ end_time = time.time()
+
+ Logger.info("🕒 Time taken to get response: {} sec".format(end_time-start_time))
+ if is_predicted:
+ output.pop("error_message")
+ response = {
+ "success": True,
+ "message": "Successfully predicted the response",
+ "data": output
+ }
+ return JSONResponse(status_code=200, content=response)
+ else:
+ response = {"success": False, "message": output["error_message"], "data": {}}
+ return JSONResponse(status_code=500, content=response)
+ except Exception as e:
+ response = {"success": False, "message": str(e), "data": {}}
+ return JSONResponse(status_code=500, content=response)
+
+
+@router.post("/ai-examiner/hint-motivate",
+ responses={404: {
+ "model": NotFoundErrorResponseModel
+ }})
+async def create_examiner_hint_motivate(
+ input_payload: AIExaminerHintMotivateModel):
+ """Analyze job
+ Args:
+ input_payload (AIExaminerHintMotivateModel)
+ Raises:
+ Exception: 500 Internal Server Error if something went wrong
+ Returns:
+ JSON: Success/Fail Message
+ """
+ try:
+ input_payload_dict = {**input_payload.dict()}
+
+ ai_question = input_payload_dict["ai_question"]
+ student_solution = input_payload_dict["student_solution"] # current incorrect solution
+ topic = input_payload_dict["topic"]
+
+ start_time = time.time()
+ is_predicted, output = await interactive_ai_examiner.examiner_hint_motivate(
+ ai_question=ai_question,
+ student_solution=student_solution,
+ topic=topic
+ )
+ end_time = time.time()
+
+ Logger.info("🕒 Time taken to get response: {} sec".format(end_time-start_time))
+ if is_predicted:
+ output.pop("error_message")
+ response = {
+ "success": True,
+ "message": "Successfully predicted the response",
+ "data": output
+ }
+ return JSONResponse(status_code=200, content=response)
+ else:
+ response = {"success": False, "message": output["error_message"], "data": {}}
+ return JSONResponse(status_code=500, content=response)
+ except Exception as e:
+ response = {"success": False, "message": str(e), "data": {}}
+ return JSONResponse(status_code=500, content=response)
\ No newline at end of file
diff --git a/api/src/routes/ask_doubt.py b/api/src/routes/ask_doubt.py
new file mode 100644
index 000000000..b042a1a5a
--- /dev/null
+++ b/api/src/routes/ask_doubt.py
@@ -0,0 +1,69 @@
+""" Ask Doubt endpoints """
+import time
+from fastapi import APIRouter
+from fastapi.responses import JSONResponse
+from schemas.error import NotFoundErrorResponseModel, InternalServerErrorResponseModel
+from schemas.ask_doubt import AskDoubtModel
+
+from config import ASK_DOUBT_CONFIG
+from core.extractive_qa import ExtractiveQuestionAnswering
+
+from utils.logging_handler import Logger
+
+router = APIRouter(
+ tags=["Ask Doubt"],
+ responses={500: {
+ "model": InternalServerErrorResponseModel
+ }})
+
+##################################################################
+
+extractive_qa = ExtractiveQuestionAnswering.load(
+ **ASK_DOUBT_CONFIG)
+
+@router.post("/ask-doubt",
+ responses={404: {
+ "model": NotFoundErrorResponseModel
+ }})
+async def create_ask_doubt(
+ input_payload: AskDoubtModel):
+ """Analyze job
+ Args:
+ input_payload (AskDoubtModel)
+ Raises:
+ Exception: 500 Internal Server Error if something went wrong
+ Returns:
+ JSON: Success/Fail Message
+ """
+ try:
+ input_payload_dict = {**input_payload.dict()}
+
+ question = input_payload_dict["question"]
+
+ max_answer_length = input_payload_dict["max_answer_length"]
+ max_seq_length = input_payload_dict["max_seq_length"]
+ top_n = input_payload_dict["top_n"]
+ top_k = input_payload_dict["top_k"]
+
+ Logger.info("Input question: {}".format(question))
+ start_time = time.time()
+ output = await extractive_qa.predict(
+ question=question,
+ max_answer_length=max_answer_length,
+ max_seq_length=max_seq_length,
+ top_n=top_n,
+ top_k=top_k
+ )
+ end_time = time.time()
+
+ Logger.info("🕒 Time taken to get response: {} sec".format(end_time-start_time))
+
+ response = {
+ "success": True,
+ "message": "Successfully predicted the response",
+ "data": output
+ }
+ return JSONResponse(status_code=200, content=response)
+ except Exception as e:
+ response = {"success": False, "message": str(e), "data": {}}
+ return JSONResponse(status_code=500, content=response)
diff --git a/api/src/schemas/__init__.py b/api/src/schemas/__init__.py
new file mode 100644
index 000000000..e69de29bb
diff --git a/api/src/schemas/ai_examiner.py b/api/src/schemas/ai_examiner.py
new file mode 100644
index 000000000..a4865806a
--- /dev/null
+++ b/api/src/schemas/ai_examiner.py
@@ -0,0 +1,58 @@
+"""
+Pydantic Models for API's
+"""
+from typing import Optional, List, Literal
+from pydantic import BaseModel
+# pylint: disable = line-too-long
+
+
+class BasicModel(BaseModel):
+ """KeyInsights Skeleton Pydantic Model"""
+ topic: str
+
+class AIExaminerAskQuestionModel(BasicModel):
+ """Input Pydantic Model"""
+ context: str
+ question_type: Literal['Open Ended', 'Single Choice', 'Multiple Choice', 'Yes or No Choice'] = 'Single Choice'
+
+ class Config():
+ orm_mode = True
+ schema_extra = {
+ "example": {
+ "topic": "Deep Learning",
+ "context": "Deep learning is part of a broader family of machine learning methods, \
+ which is based on artificial neural networks with representation learning. \
+ Learning can be supervised, semi-supervised or unsupervised.",
+ "question_type": "Single Choice"
+ }
+ }
+
+class AIExaminerEvalAnswerModel(BasicModel):
+ """Input Pydantic Model"""
+ ai_question: str
+ student_solution: str
+
+ class Config():
+ orm_mode = True
+ schema_extra = {
+ "example": {
+ "topic": "Deep Learning",
+ "ai_question": "",
+ "student_solution": ""
+ }
+ }
+
+class AIExaminerHintMotivateModel(BasicModel):
+ """Input Pydantic Model"""
+ ai_question: str
+ student_solution: str
+
+ class Config():
+ orm_mode = True
+ schema_extra = {
+ "example": {
+ "topic": "Deep Learning",
+ "ai_question": "",
+ "student_solution": ""
+ }
+ }
\ No newline at end of file
diff --git a/api/src/schemas/ask_doubt.py b/api/src/schemas/ask_doubt.py
new file mode 100644
index 000000000..15e23b7f5
--- /dev/null
+++ b/api/src/schemas/ask_doubt.py
@@ -0,0 +1,31 @@
+"""
+Pydantic Models for API's
+"""
+from typing import Optional, List, Literal
+from pydantic import BaseModel
+# pylint: disable = line-too-long
+
+
+class BasicModel(BaseModel):
+ """KeyInsights Skeleton Pydantic Model"""
+ question: str
+ max_answer_length: Optional[int] = 30
+ max_seq_length: Optional[int] = 384
+ top_n: Optional[int] = 2
+ top_k: Optional[int] = 1
+
+
+class AskDoubtModel(BasicModel):
+ """Input Pydantic Model"""
+
+ class Config():
+ orm_mode = True
+ schema_extra = {
+ "example": {
+ "question": "",
+ "max_answer_length": 30,
+ "max_seq_length": 384,
+ "top_n": 2,
+ "top_k": 1
+ }
+ }
\ No newline at end of file
diff --git a/api/src/schemas/error.py b/api/src/schemas/error.py
new file mode 100644
index 000000000..1330b60b0
--- /dev/null
+++ b/api/src/schemas/error.py
@@ -0,0 +1,37 @@
+"""
+Pydantic models for different status codes
+"""
+from typing import Optional
+from pydantic import BaseModel
+
+
+class NotFoundErrorResponseModel(BaseModel):
+ success: bool = False
+ message: Optional[str] = None
+ data: Optional[dict] = {}
+
+ class Config():
+ orm_mode = True
+ schema_extra = {
+ "example": {
+ "success": False,
+ "message": "Resource not found",
+ "data": {}
+ }
+ }
+
+
+class InternalServerErrorResponseModel(BaseModel):
+ success: bool = False
+ message: Optional[str] = "Internal Server Error"
+ data: Optional[dict] = {}
+
+ class Config():
+ orm_mode = True
+ schema_extra = {
+ "example": {
+ "success": False,
+ "message": "Internal server error",
+ "data": {}
+ }
+ }
diff --git a/api/src/start.sh b/api/src/start.sh
new file mode 100644
index 000000000..b9b112fc7
--- /dev/null
+++ b/api/src/start.sh
@@ -0,0 +1,8 @@
+#! /usr/bin/env sh
+set -e
+
+export NUM_WORKERS=1
+export SERVER_PORT=8500
+export TIMEOUT=3000
+
+exec gunicorn main:app --workers "$NUM_WORKERS" --worker-class uvicorn.workers.UvicornWorker --bind 0.0.0.0:"$SERVER_PORT" --timeout "$TIMEOUT"
\ No newline at end of file
diff --git a/api/src/utils/__init__.py b/api/src/utils/__init__.py
new file mode 100644
index 000000000..e69de29bb
diff --git a/api/src/utils/errors.py b/api/src/utils/errors.py
new file mode 100644
index 000000000..439f22491
--- /dev/null
+++ b/api/src/utils/errors.py
@@ -0,0 +1,9 @@
+"""class for handling errors"""
+
+
+class ResourceNotFoundException(Exception):
+ """Class for custom Exceptions"""
+
+ def __init__(self, message="Resource not found"):
+ self.message = message
+ super().__init__(self.message)
diff --git a/api/src/utils/logging_handler.py b/api/src/utils/logging_handler.py
new file mode 100644
index 000000000..853a459b7
--- /dev/null
+++ b/api/src/utils/logging_handler.py
@@ -0,0 +1,30 @@
+"""class and methods for logs handling."""
+
+import logging
+
+logging.basicConfig(
+ format='%(asctime)s:%(levelname)s:%(message)s', level=logging.DEBUG)
+
+
+class Logger():
+ """class def handling logs."""
+
+ @staticmethod
+ def info(message):
+ """Display info logs."""
+ logging.info(message)
+
+ @staticmethod
+ def warning(message):
+ """Display warning logs."""
+ logging.warning(message)
+
+ @staticmethod
+ def debug(message):
+ """Display debug logs."""
+ logging.debug(message)
+
+ @staticmethod
+ def error(message):
+ """Display error logs."""
+ logging.error(message)
diff --git a/assets/.DS_Store b/assets/.DS_Store
new file mode 100644
index 000000000..cb6ae2635
Binary files /dev/null and b/assets/.DS_Store differ
diff --git a/assets/AI-Examiner.png b/assets/AI-Examiner.png
new file mode 100644
index 000000000..7e296b743
Binary files /dev/null and b/assets/AI-Examiner.png differ
diff --git a/assets/Ask-Doubt.png b/assets/Ask-Doubt.png
new file mode 100644
index 000000000..355f1a5e0
Binary files /dev/null and b/assets/Ask-Doubt.png differ
diff --git a/assets/EDA-Worldcloud.png b/assets/EDA-Worldcloud.png
new file mode 100644
index 000000000..54ee71c75
Binary files /dev/null and b/assets/EDA-Worldcloud.png differ
diff --git a/assets/Intel-AI-Kit-Banner.png b/assets/Intel-AI-Kit-Banner.png
new file mode 100644
index 000000000..f261e1efb
Binary files /dev/null and b/assets/Intel-AI-Kit-Banner.png differ
diff --git a/assets/Intel-Optimization.png b/assets/Intel-Optimization.png
new file mode 100644
index 000000000..389b4427d
Binary files /dev/null and b/assets/Intel-Optimization.png differ
diff --git a/assets/Intel-Tech-Stack.png b/assets/Intel-Tech-Stack.png
new file mode 100644
index 000000000..f06076aa5
Binary files /dev/null and b/assets/Intel-Tech-Stack.png differ
diff --git a/assets/Process-Flow.png b/assets/Process-Flow.png
new file mode 100644
index 000000000..f8158207b
Binary files /dev/null and b/assets/Process-Flow.png differ
diff --git a/assets/Prototype-Tech-Stack.png b/assets/Prototype-Tech-Stack.png
new file mode 100644
index 000000000..cb6a4b6b7
Binary files /dev/null and b/assets/Prototype-Tech-Stack.png differ
diff --git a/benchmark/Emb-TFIDF/.DS_Store b/benchmark/Emb-TFIDF/.DS_Store
new file mode 100644
index 000000000..5008ddfcf
Binary files /dev/null and b/benchmark/Emb-TFIDF/.DS_Store differ
diff --git a/benchmark/Emb-TFIDF/Latency.png b/benchmark/Emb-TFIDF/Latency.png
new file mode 100644
index 000000000..e62c7f671
Binary files /dev/null and b/benchmark/Emb-TFIDF/Latency.png differ
diff --git a/benchmark/Emb-TFIDF/Throughput.png b/benchmark/Emb-TFIDF/Throughput.png
new file mode 100644
index 000000000..0688d7266
Binary files /dev/null and b/benchmark/Emb-TFIDF/Throughput.png differ
diff --git a/benchmark/Emb-TFIDF/Training-Time.png b/benchmark/Emb-TFIDF/Training-Time.png
new file mode 100644
index 000000000..fde562252
Binary files /dev/null and b/benchmark/Emb-TFIDF/Training-Time.png differ
diff --git a/benchmark/QA-ALBERT/.DS_Store b/benchmark/QA-ALBERT/.DS_Store
new file mode 100644
index 000000000..e705a463d
Binary files /dev/null and b/benchmark/QA-ALBERT/.DS_Store differ
diff --git a/benchmark/QA-ALBERT/F1-score.png b/benchmark/QA-ALBERT/F1-score.png
new file mode 100644
index 000000000..349f4eac8
Binary files /dev/null and b/benchmark/QA-ALBERT/F1-score.png differ
diff --git a/benchmark/QA-ALBERT/Latency.png b/benchmark/QA-ALBERT/Latency.png
new file mode 100644
index 000000000..4cca25474
Binary files /dev/null and b/benchmark/QA-ALBERT/Latency.png differ
diff --git a/benchmark/QA-ALBERT/Speed-Up.png b/benchmark/QA-ALBERT/Speed-Up.png
new file mode 100644
index 000000000..f945b088c
Binary files /dev/null and b/benchmark/QA-ALBERT/Speed-Up.png differ
diff --git a/benchmark/QA-ALBERT/Throughput.png b/benchmark/QA-ALBERT/Throughput.png
new file mode 100644
index 000000000..28c1f656c
Binary files /dev/null and b/benchmark/QA-ALBERT/Throughput.png differ
diff --git a/dataset/.DS_Store b/dataset/.DS_Store
new file mode 100644
index 000000000..bd1ac031c
Binary files /dev/null and b/dataset/.DS_Store differ
diff --git a/dataset/courses/.DS_Store b/dataset/courses/.DS_Store
new file mode 100644
index 000000000..ee318743e
Binary files /dev/null and b/dataset/courses/.DS_Store differ
diff --git a/dataset/courses/Algebra/.DS_Store b/dataset/courses/Algebra/.DS_Store
new file mode 100644
index 000000000..35d7e3213
Binary files /dev/null and b/dataset/courses/Algebra/.DS_Store differ
diff --git a/dataset/courses/Algebra/Study-Material/.DS_Store b/dataset/courses/Algebra/Study-Material/.DS_Store
new file mode 100644
index 000000000..55f0c23e7
Binary files /dev/null and b/dataset/courses/Algebra/Study-Material/.DS_Store differ
diff --git a/dataset/courses/Algebra/Study-Material/Week-1/.DS_Store b/dataset/courses/Algebra/Study-Material/Week-1/.DS_Store
new file mode 100644
index 000000000..9c1b213ab
Binary files /dev/null and b/dataset/courses/Algebra/Study-Material/Week-1/.DS_Store differ
diff --git a/dataset/courses/Algebra/Study-Material/Week-1/Algebra-Basics/.gitignore b/dataset/courses/Algebra/Study-Material/Week-1/Algebra-Basics/.gitignore
new file mode 100644
index 000000000..e69de29bb
diff --git a/dataset/courses/Algebra/Viva-Material/.DS_Store b/dataset/courses/Algebra/Viva-Material/.DS_Store
new file mode 100644
index 000000000..f3b1ba9a9
Binary files /dev/null and b/dataset/courses/Algebra/Viva-Material/.DS_Store differ
diff --git a/dataset/courses/Algebra/Viva-Material/context.txt b/dataset/courses/Algebra/Viva-Material/context.txt
new file mode 100644
index 000000000..8252a1ec6
--- /dev/null
+++ b/dataset/courses/Algebra/Viva-Material/context.txt
@@ -0,0 +1,54 @@
+* What is Algebra
+
+Algebra is a branch of mathematics that deals with symbols and letters representing numbers and quantities. It is a powerful tool for representing and solving mathematical problems and equations using variables and mathematical operations. Algebra enables us to study patterns, relationships, and structures in a more generalized and abstract way.
+
+In algebra, equations and expressions are written using variables (such as x, y, or z) to represent unknown quantities or values that can vary. These variables can be manipulated and solved for, allowing us to find solutions and understand the relationships between different quantities.
+
+
+* Some Algebra Rules
+
+Here are some important algebraic rules and concepts explained concisely:
+
+1. Order of Operations:
+ - The order of operations dictates the sequence in which operations should be performed in an expression.
+ - Use the acronym PEMDAS: Parentheses, Exponents, Multiplication and Division (from left to right), and Addition and Subtraction (from left to right).
+
+2. Commutative Property:
+ - The commutative property states that the order of addition or multiplication does not affect the result.
+ - Addition: a + b = b + a
+ - Multiplication: a × b = b × a
+
+3. Associative Property:
+ - The associative property states that the grouping of numbers being added or multiplied does not affect the result.
+ - Addition: (a + b) + c = a + (b + c)
+ - Multiplication: (a × b) × c = a × (b × c)
+
+4. Distributive Property:
+ - The distributive property allows us to multiply a number by a sum or difference.
+ - a × (b + c) = a × b + a × c
+
+5. Identity Property:
+ - The identity property states that there are special numbers (called identities) that, when combined with other numbers in a particular operation, leave the other number unchanged.
+ - Addition: a + 0 = a
+ - Multiplication: a × 1 = a
+
+6. Inverse Property:
+ - The inverse property states that there are special numbers (called inverses) that, when combined with other numbers in a particular operation, yield the identity element for that operation.
+ - Addition: a + (-a) = 0
+ - Multiplication: a × (1/a) = 1
+
+7. Zero Property:
+ - The zero property states that any number multiplied by zero is zero.
+ - a × 0 = 0
+
+8. Substitution Property:
+ - The substitution property allows us to replace a variable with an equivalent expression in an equation or expression.
+
+
+* Addition & Multiplication Properties
+
+In algebra, the Addition and Multiplication Properties are fundamental concepts that govern the manipulation and simplification of equations. These properties provide rules for performing operations on numbers and variables, allowing mathematicians to solve equations and express mathematical relationships. In this context, addition refers to the combining of quantities, while multiplication involves the repeated addition of quantities. Understanding these properties is crucial for solving equations, simplifying expressions, and exploring the relationships between numbers and variables.
+
+The Addition Property of Equality states that if two quantities are equal, then adding the same number to both sides of the equation will not change the equality. In other words, if a = b, then a + c = b + c, where c is any real number. This property allows us to manipulate equations by adding or subtracting terms to isolate variables or simplify expressions. For example, consider the equation 2x - 5 = 7. By applying the Addition Property of Equality, we can add 5 to both sides to obtain 2x = 12. This step allows us to isolate the variable x and solve for its value.
+
+Similarly, the Multiplication Property of Equality states that if two quantities are equal, then multiplying both sides of the equation by the same non-zero number will preserve the equality. In algebraic terms, if a = b, then ac = bc, where c ≠ 0. This property is essential for solving equations involving multiplication or division. For instance, let's consider the equation 3x = 15. By applying the Multiplication Property of Equality, we can multiply both sides by 1/3 to find x = 5. This operation helps us determine the value of the variable x.
\ No newline at end of file
diff --git a/dataset/courses/Algebra/algebra_banner.jpeg b/dataset/courses/Algebra/algebra_banner.jpeg
new file mode 100644
index 000000000..e945d0ed9
Binary files /dev/null and b/dataset/courses/Algebra/algebra_banner.jpeg differ
diff --git a/dataset/courses/Computer Vision/.DS_Store b/dataset/courses/Computer Vision/.DS_Store
new file mode 100644
index 000000000..38b28185d
Binary files /dev/null and b/dataset/courses/Computer Vision/.DS_Store differ
diff --git a/dataset/courses/Computer Vision/Study-Material/.DS_Store b/dataset/courses/Computer Vision/Study-Material/.DS_Store
new file mode 100644
index 000000000..76bda8c0b
Binary files /dev/null and b/dataset/courses/Computer Vision/Study-Material/.DS_Store differ
diff --git a/dataset/courses/Computer Vision/Study-Material/Week-1/.gitignore b/dataset/courses/Computer Vision/Study-Material/Week-1/.gitignore
new file mode 100644
index 000000000..e69de29bb
diff --git a/dataset/courses/Computer Vision/Viva-Material/.gitignore b/dataset/courses/Computer Vision/Viva-Material/.gitignore
new file mode 100644
index 000000000..e69de29bb
diff --git a/dataset/courses/Computer Vision/computer_vision_banner.jpeg b/dataset/courses/Computer Vision/computer_vision_banner.jpeg
new file mode 100644
index 000000000..3cc1ea947
Binary files /dev/null and b/dataset/courses/Computer Vision/computer_vision_banner.jpeg differ
diff --git a/dataset/courses/Deep Learning/.DS_Store b/dataset/courses/Deep Learning/.DS_Store
new file mode 100644
index 000000000..dc5ee06a4
Binary files /dev/null and b/dataset/courses/Deep Learning/.DS_Store differ
diff --git a/dataset/courses/Deep Learning/Study-Material/.DS_Store b/dataset/courses/Deep Learning/Study-Material/.DS_Store
new file mode 100644
index 000000000..713513636
Binary files /dev/null and b/dataset/courses/Deep Learning/Study-Material/.DS_Store differ
diff --git a/dataset/courses/Deep Learning/Study-Material/Week-1/.DS_Store b/dataset/courses/Deep Learning/Study-Material/Week-1/.DS_Store
new file mode 100644
index 000000000..8f59b5fa5
Binary files /dev/null and b/dataset/courses/Deep Learning/Study-Material/Week-1/.DS_Store differ
diff --git a/dataset/courses/Deep Learning/Study-Material/Week-1/Supervised-Learning-with-Neural-Networks/.DS_Store b/dataset/courses/Deep Learning/Study-Material/Week-1/Supervised-Learning-with-Neural-Networks/.DS_Store
new file mode 100644
index 000000000..49c8f5272
Binary files /dev/null and b/dataset/courses/Deep Learning/Study-Material/Week-1/Supervised-Learning-with-Neural-Networks/.DS_Store differ
diff --git a/dataset/courses/Deep Learning/Study-Material/Week-1/Supervised-Learning-with-Neural-Networks/doc.pdf b/dataset/courses/Deep Learning/Study-Material/Week-1/Supervised-Learning-with-Neural-Networks/doc.pdf
new file mode 100644
index 000000000..7c78c0c16
Binary files /dev/null and b/dataset/courses/Deep Learning/Study-Material/Week-1/Supervised-Learning-with-Neural-Networks/doc.pdf differ
diff --git a/dataset/courses/Deep Learning/Study-Material/Week-1/Supervised-Learning-with-Neural-Networks/subtitle-en.vtt b/dataset/courses/Deep Learning/Study-Material/Week-1/Supervised-Learning-with-Neural-Networks/subtitle-en.vtt
new file mode 100644
index 000000000..317ce8c95
--- /dev/null
+++ b/dataset/courses/Deep Learning/Study-Material/Week-1/Supervised-Learning-with-Neural-Networks/subtitle-en.vtt
@@ -0,0 +1,46 @@
+WEBVTT
+
+00:00:03.320 --> 00:00:34.890
+There's been a lot of hype about neural networks. And perhaps some of that hype is justified, given how well they're working. But it turns out that so far, almost all the economic value created by neural networks has been through one type of machine learning, called supervised learning. Let's see what that means, and let's go over some examples. In supervised learning, you have some input x, and you want to learn a function mapping to some output y. So for example, just now we saw the housing price prediction application where
+
+00:00:34.890 --> 00:01:10.700
+you input some features of a home and try to output or estimate the price y. Here are some other examples that neural networks have been applied to very effectively. Possibly the single most lucrative application of deep learning today is online advertising, maybe not the most inspiring, but certainly very lucrative, in which, by inputting information about an ad to the website it's thinking of showing you, and some information about the user, neural networks have gotten very good at predicting whether or not you click on an ad.
+
+00:01:10.700 --> 00:01:45.290
+And by showing you and showing users the ads that you are most likely to click on, this has been an incredibly lucrative application of neural networks at multiple companies. Because the ability to show you ads that you're more likely to click on has a direct impact on the bottom line of some of the very large online advertising companies. Computer vision has also made huge strides in the last several years, mostly due to deep learning. So you might input an image and want to output an index, say from 1 to 1,000 trying to tell you if this picture,
+
+00:01:45.290 --> 00:02:15.930
+it might be any one of, say a 1000 different images. So, you might us that for photo tagging. I think the recent progress in speech recognition has also been very exciting, where you can now input an audio clip to a neural network, and have it output a text transcript. Machine translation has also made huge strides thanks to deep learning where now you can have a neural network input an English sentence and directly output say, a Chinese sentence. And in autonomous driving, you might input an image, say a picture of what's in
+
+00:02:15.930 --> 00:02:48.660
+front of your car as well as some information from a radar, and based on that, maybe a neural network can be trained to tell you the position of the other cars on the road. So this becomes a key component in autonomous driving systems. So a lot of the value creation through neural networks has been through cleverly selecting what should be x and what should be y for your particular problem, and then fitting this supervised learning component into often a bigger system such as an autonomous vehicle.
+
+00:02:48.660 --> 00:03:27.840
+It turns out that slightly different types of neural networks are useful for different applications. For example, in the real estate application that we saw in the previous video, we use a universally standard neural network architecture, right? Maybe for real estate and online advertising might be a relatively standard neural network, like the one that we saw. For image applications we'll often use convolution on neural networks, often abbreviated CNN. And for sequence data. So for example, audio has a temporal component, right?
+
+00:03:27.840 --> 00:04:04.360
+Audio is played out over time, so audio is most naturally represented as a one-dimensional time series or as a one-dimensional temporal sequence. And so for sequence data, you often use an RNN, a recurrent neural network. Language, English and Chinese, the alphabets or the words come one at a time. So language is also most naturally represented as sequence data. And so more complex versions of RNNs are often used for these applications. And then, for more complex applications, like autonomous driving, where you have
+
+00:04:04.360 --> 00:04:41.830
+an image, that might suggest more of a CNN convolution neural network structure and radar info which is something quite different. You might end up with a more custom, or some more complex, hybrid neural network architecture. So, just to be a bit more concrete about what are the standard CNN and RNN architectures. So in the literature you might have seen pictures like this. So that's a standard neural net. You might have seen pictures like this. Well this is an example of a Convolutional Neural Network, and we'll see in
+
+00:04:41.830 --> 00:05:14.960
+a later course exactly what this picture means and how can you implement this. But convolutional networks are often use for image data. And you might also have seen pictures like this. And you'll learn how to implement this in a later course. Recurrent neural networks are very good for this type of one-dimensional sequence data that has maybe a temporal component. You might also have heard about applications of machine learning to both Structured Data and Unstructured Data. Here's what the terms mean.
+
+00:05:14.960 --> 00:05:49.740
+Structured Data means basically databases of data. So, for example, in housing price prediction, you might have a database or the column that tells you the size and the number of bedrooms. So, this is structured data, or in predicting whether or not a user will click on an ad, you might have information about the user, such as the age, some information about the ad, and then labels why that you're trying to predict. So that's structured data, meaning that each of the features, such as size of the house, the number of bedrooms, or
+
+00:05:49.740 --> 00:06:26.270
+the age of a user, has a very well defined meaning. In contrast, unstructured data refers to things like audio, raw audio, or images where you might want to recognize what's in the image or text. Here the features might be the pixel values in an image or the individual words in a piece of text. Historically, it has been much harder for computers to make sense of unstructured data compared to structured data. And the fact the human race has evolved to be very good at understanding audio cues as well as images.
+
+00:06:26.270 --> 00:06:55.220
+And then text was a more recent invention, but people are just really good at interpreting unstructured data. And so one of the most exciting things about the rise of neural networks is that, thanks to deep learning, thanks to neural networks, computers are now much better at interpreting unstructured data as well compared to just a few years ago. And this creates opportunities for many new exciting applications that use speech recognition, image recognition, natural language processing on text,
+
+00:06:56.230 --> 00:07:28.690
+much more than was possible even just two or three years ago. I think because people have a natural empathy to understanding unstructured data, you might hear about neural network successes on unstructured data more in the media because it's just cool when the neural network recognizes a cat. We all like that, and we all know what that means. But it turns out that a lot of short term economic value that neural networks are creating has also been on structured data, such as much better advertising systems, much better profit recommendations, and
+
+00:07:28.690 --> 00:08:01.360
+just a much better ability to process the giant databases that many companies have to make accurate predictions from them. So in this course, a lot of the techniques we'll go over will apply to both structured data and to unstructured data. For the purposes of explaining the algorithms, we will draw a little bit more on examples that use unstructured data. But as you think through applications of neural networks within your own team I hope you find both uses for them in both structured and unstructured data.
+
+00:08:02.590 --> 00:08:28.940
+So neural networks have transformed supervised learning and are creating tremendous economic value. It turns out though, that the basic technical ideas behind neural networks have mostly been around, sometimes for many decades. So why is it, then, that they're only just now taking off and working so well? In the next video, we'll talk about why it's only quite recently that neural networks have become this incredibly powerful tool that you can use.
\ No newline at end of file
diff --git a/dataset/courses/Deep Learning/Study-Material/Week-1/Supervised-Learning-with-Neural-Networks/video.mp4 b/dataset/courses/Deep Learning/Study-Material/Week-1/Supervised-Learning-with-Neural-Networks/video.mp4
new file mode 100644
index 000000000..5ce0c316e
Binary files /dev/null and b/dataset/courses/Deep Learning/Study-Material/Week-1/Supervised-Learning-with-Neural-Networks/video.mp4 differ
diff --git a/dataset/courses/Deep Learning/Study-Material/Week-1/What-is-Neural-Network/doc.pdf b/dataset/courses/Deep Learning/Study-Material/Week-1/What-is-Neural-Network/doc.pdf
new file mode 100644
index 000000000..d0c95fd66
Binary files /dev/null and b/dataset/courses/Deep Learning/Study-Material/Week-1/What-is-Neural-Network/doc.pdf differ
diff --git a/dataset/courses/Deep Learning/Study-Material/Week-1/What-is-Neural-Network/subtitle-en.vtt b/dataset/courses/Deep Learning/Study-Material/Week-1/What-is-Neural-Network/subtitle-en.vtt
new file mode 100644
index 000000000..051f7b7cd
--- /dev/null
+++ b/dataset/courses/Deep Learning/Study-Material/Week-1/What-is-Neural-Network/subtitle-en.vtt
@@ -0,0 +1,37 @@
+WEBVTT
+
+00:00:01.050 --> 00:00:28.501
+The term, Deep Learning, refers to training Neural Networks, sometimes very large Neural Networks. So what exactly is a Neural Network? In this video, let's try to give you some of the basic intuitions. Let's start to the Housing Price Prediction example. Let's say you have a data sets with six houses, so you know the size of the houses in square feet or square meters and you know the price of the house and you want to fit a function to predict the price of the houses, the function of the size.
+
+00:00:28.501 --> 00:01:03.310
+So if you are familiar with linear regression you might say, well let's put a straight line to these data so and we get a straight line like that. But to be Pathans you might say well we know that prices can never be negative, right. So instead of the straight line fit which eventually will become negative, let's bend the curve here. So it just ends up zero here. So this thick blue line ends up being your function for predicting the price of the house as a function of this size. Whereas zero here and then there's a straight line fit to the right.
+
+00:01:04.408 --> 00:01:48.940
+So you can think of this function that you've just fit the housing prices as a very simple neural network. It's almost as simple as possible neural network. Let me draw it here. We have as the input to the neural network the size of a house which one we call x. It goes into this node, this little circle and then it outputs the price which we call y. So this little circle, which is a single neuron in a neural network, implements this function that we drew on the left. And all the neuron does is it inputs the size, computes this linear function,
+
+00:01:48.940 --> 00:02:30.200
+takes a max of zero, and then outputs the estimated price. And by the way in the neural network literature, you see this function a lot. This function which goes to zero sometimes and then it'll takes of as a straight line. This function is called a ReLU function which stands for rectified linear units. So R-E-L-U. And rectify just means taking a max of 0 which is why you get a function shape like this. You don't need to worry about ReLU units for now but it's just something you see again later in this course.
+
+00:02:30.200 --> 00:03:08.164
+So if this is a single neuron, neural network, really a tiny little neural network, a larger neural network is then formed by taking many of the single neurons and stacking them together. So, if you think of this neuron that's being like a single Lego brick, you then get a bigger neural network by stacking together many of these Lego bricks. Let's see an example. Let’s say that instead of predicting the price of a house just from the size, you now have other features. You know other things about the host, such as the number of bedrooms,
+
+00:03:08.164 --> 00:03:48.820
+I should have wrote [INAUDIBLE] bedrooms, and you might think that one of the things that really affects the price of a house is family size, right? So can this house fit your family of three, or family of four, or family of five? And it's really based on the size in square feet or square meters, and the number of bedrooms that determines whether or not a house can fit your family's family size. And then maybe you know the zip codes, in different countries it's called a postal code of a house. And the zip code maybe as a future to tells you, walkability?
+
+00:03:48.820 --> 00:04:24.936
+So is this neighborhood highly walkable? Thing just walks the grocery store? Walk the school? Do you need to drive? And some people prefer highly walkable neighborhoods. And then the zip code as well as the wealth maybe tells you, right. Certainly in the United States but some other countries as well. Tells you how good is the school quality. So each of these little circles I'm drawing, can be one of those ReLU, rectified linear units or some other slightly non linear function. So that based on the size and number of bedrooms,
+
+00:04:24.936 --> 00:05:03.350
+you can estimate the family size, their zip code, based on walkability, based on zip code and wealth can estimate the school quality. And then finally you might think that well the way people decide how much they're will to pay for a house, is they look at the things that really matter to them. In this case family size, walkability, and school quality and that helps you predict the price. So in the example x is all of these four inputs. And y is the price you're trying to predict. And so by stacking together a few of the single neurons or the simple predictors
+
+00:05:03.350 --> 00:05:40.365
+we have from the previous slide, we now have a slightly larger neural network. How you manage neural network is that when you implement it, you need to give it just the input x and the output y for a number of examples in your training set and all this things in the middle, they will figure out by itself. So what you actually implement is this. Where, here, you have a neural network with four inputs. So the input features might be the size, number of bedrooms, the zip code or postal code, and the wealth of the neighborhood.
+
+00:05:40.365 --> 00:06:21.070
+And so given these input features, the job of the neural network will be to predict the price y. And notice also that each of these circle, these are called hidden units in the neural network, that each of them takes its inputs all four input features. So for example, rather than saying these first nodes represent family size and family size depends only on the features X1 and X2. Instead, we're going to say, well neural network, you decide whatever you want this known to be. And we'll give you all four of the features to complete whatever you want.
+
+00:06:21.070 --> 00:06:54.290
+So we say that layers that this is input layer and this layer in the middle of the neural network are density connected. Because every input feature is connected to every one of these circles in the middle. And the remarkable thing about neural networks is that, given enough data about x and y, given enough training examples with both x and y, neural networks are remarkably good at figuring out functions that accurately map from x to y. So, that's a basic neural network. In turns out that as you build out your own neural networks,
+
+00:06:54.290 --> 00:07:16.670
+you probably find them to be most useful, most powerful in supervised learning incentives, meaning that you're trying to take an input x and map it to some output y, like we just saw in the housing price prediction example. In the next video let's go over some more examples of supervised learning and some examples of where you might find your networks to be incredibly helpful for your applications as well.
\ No newline at end of file
diff --git a/dataset/courses/Deep Learning/Study-Material/Week-1/What-is-Neural-Network/video.mp4 b/dataset/courses/Deep Learning/Study-Material/Week-1/What-is-Neural-Network/video.mp4
new file mode 100755
index 000000000..b8a2d71bf
Binary files /dev/null and b/dataset/courses/Deep Learning/Study-Material/Week-1/What-is-Neural-Network/video.mp4 differ
diff --git a/dataset/courses/Deep Learning/Study-Material/Week-2/.DS_Store b/dataset/courses/Deep Learning/Study-Material/Week-2/.DS_Store
new file mode 100644
index 000000000..1137c1e0b
Binary files /dev/null and b/dataset/courses/Deep Learning/Study-Material/Week-2/.DS_Store differ
diff --git a/dataset/courses/Deep Learning/Study-Material/Week-2/Binary-Classification/.DS_Store b/dataset/courses/Deep Learning/Study-Material/Week-2/Binary-Classification/.DS_Store
new file mode 100644
index 000000000..6726216f7
Binary files /dev/null and b/dataset/courses/Deep Learning/Study-Material/Week-2/Binary-Classification/.DS_Store differ
diff --git a/dataset/courses/Deep Learning/Study-Material/Week-2/Binary-Classification/doc.pdf b/dataset/courses/Deep Learning/Study-Material/Week-2/Binary-Classification/doc.pdf
new file mode 100644
index 000000000..5ed81c651
Binary files /dev/null and b/dataset/courses/Deep Learning/Study-Material/Week-2/Binary-Classification/doc.pdf differ
diff --git a/dataset/courses/Deep Learning/Study-Material/Week-2/Binary-Classification/subtitle-en.vtt b/dataset/courses/Deep Learning/Study-Material/Week-2/Binary-Classification/subtitle-en.vtt
new file mode 100644
index 000000000..5c3640aac
--- /dev/null
+++ b/dataset/courses/Deep Learning/Study-Material/Week-2/Binary-Classification/subtitle-en.vtt
@@ -0,0 +1,563 @@
+WEBVTT
+
+1
+00:00:00.920 --> 00:00:02.860
+Hello, and welcome back.
+
+2
+00:00:02.860 --> 00:00:08.860
+In this week we're going to go over
+the basics of neural network programming.
+
+3
+00:00:08.860 --> 00:00:11.990
+It turns out that when you
+implement a neural network there
+
+4
+00:00:11.990 --> 00:00:16.260
+are some techniques that
+are going to be really important.
+
+5
+00:00:16.260 --> 00:00:21.150
+For example, if you have a training
+set of m training examples,
+
+6
+00:00:21.150 --> 00:00:25.110
+you might be used to processing
+the training set by having a four loop
+
+7
+00:00:25.110 --> 00:00:28.240
+step through your m training examples.
+
+8
+00:00:28.240 --> 00:00:31.260
+But it turns out that when you're
+implementing a neural network,
+
+9
+00:00:31.260 --> 00:00:34.540
+you usually want to process
+your entire training set
+
+10
+00:00:34.540 --> 00:00:39.040
+without using an explicit four loop to
+loop over your entire training set.
+
+11
+00:00:39.040 --> 00:00:42.940
+So, you'll see how to do that
+in this week's materials.
+
+12
+00:00:42.940 --> 00:00:47.700
+Another idea, when you organize
+the computation of, in your network,
+
+13
+00:00:47.700 --> 00:00:51.670
+usually you have what's called a forward
+pause or forward propagation step,
+
+14
+00:00:51.670 --> 00:00:56.100
+followed by a backward pause or
+what's called a backward propagation step.
+
+15
+00:00:56.100 --> 00:01:00.010
+And so in this week's materials,
+you also get an introduction about why
+
+16
+00:01:00.010 --> 00:01:04.830
+the computations, in learning an neural
+network can be organized in this for
+
+17
+00:01:04.830 --> 00:01:08.010
+propagation and
+a separate backward propagation.
+
+18
+00:01:09.100 --> 00:01:12.620
+For this week's materials I want
+to convey these ideas using
+
+19
+00:01:12.620 --> 00:01:16.170
+logistic regression in order to make
+the ideas easier to understand.
+
+20
+00:01:16.170 --> 00:01:19.970
+But even if you've seen logistic
+regression before, I think that there'll
+
+21
+00:01:19.970 --> 00:01:23.845
+be some new and interesting ideas for
+you to pick up in this week's materials.
+
+22
+00:01:23.845 --> 00:01:25.815
+So with that, let's get started.
+
+23
+00:01:25.815 --> 00:01:30.605
+Logistic regression is an algorithm for
+binary classification.
+
+24
+00:01:30.605 --> 00:01:33.145
+So let's start by setting up the problem.
+
+25
+00:01:33.145 --> 00:01:36.925
+Here's an example of a binary
+classification problem.
+
+26
+00:01:36.925 --> 00:01:41.545
+You might have an input of an image,
+like that, and
+
+27
+00:01:41.545 --> 00:01:47.260
+want to output a label to recognize
+this image as either being a cat,
+
+28
+00:01:47.260 --> 00:01:52.140
+in which case you output 1, or
+not-cat in which case you output 0,
+
+29
+00:01:52.140 --> 00:01:57.740
+and we're going to use y
+to denote the output label.
+
+30
+00:01:57.740 --> 00:02:01.550
+Let's look at how an image is
+represented in a computer.
+
+31
+00:02:01.550 --> 00:02:05.680
+To store an image your computer
+stores three separate matrices
+
+32
+00:02:05.680 --> 00:02:09.890
+corresponding to the red, green, and
+blue color channels of this image.
+
+33
+00:02:10.990 --> 00:02:15.900
+So if your input image is
+64 pixels by 64 pixels,
+
+34
+00:02:15.900 --> 00:02:21.700
+then you would have 3 64 by 64 matrices
+
+35
+00:02:21.700 --> 00:02:27.230
+corresponding to the red, green and blue
+pixel intensity values for your images.
+
+36
+00:02:27.230 --> 00:02:31.290
+Although to make this little slide I
+drew these as much smaller matrices, so
+
+37
+00:02:31.290 --> 00:02:35.320
+these are actually 5 by 4
+matrices rather than 64 by 64.
+
+38
+00:02:35.320 --> 00:02:41.640
+So to turn these pixel intensity values-
+Into a feature vector, what we're
+
+39
+00:02:41.640 --> 00:02:48.000
+going to do is unroll all of these pixel
+values into an input feature vector x.
+
+40
+00:02:48.000 --> 00:02:53.782
+So to unroll all these pixel intensity
+values into Feature vector, what we're
+
+41
+00:02:53.782 --> 00:02:59.580
+going to do is define a feature vector x
+corresponding to this image as follows.
+
+42
+00:02:59.580 --> 00:03:03.960
+We're just going to take all
+the pixel values 255, 231, and so on.
+
+43
+00:03:03.960 --> 00:03:10.827
+255, 231, and so
+on until we've listed all the red pixels.
+
+44
+00:03:10.827 --> 00:03:15.737
+And then eventually 255 134 255,
+134 and so
+
+45
+00:03:15.737 --> 00:03:20.952
+on until we get a long feature
+vector listing out all the red,
+
+46
+00:03:20.952 --> 00:03:25.570
+green and
+blue pixel intensity values of this image.
+
+47
+00:03:25.570 --> 00:03:31.043
+If this image is a 64 by 64 image,
+the total dimension
+
+48
+00:03:31.043 --> 00:03:36.401
+of this vector x will be 64
+by 64 by 3 because that's
+
+49
+00:03:36.401 --> 00:03:41.320
+the total numbers we have
+in all of these matrixes.
+
+50
+00:03:41.320 --> 00:03:44.097
+Which in this case,
+turns out to be 12,288,
+
+51
+00:03:44.097 --> 00:03:47.330
+that's what you get if you
+multiply all those numbers.
+
+52
+00:03:47.330 --> 00:03:51.870
+And so we're going to use nx=12288
+
+53
+00:03:51.870 --> 00:03:55.080
+to represent the dimension
+of the input features x.
+
+54
+00:03:55.080 --> 00:03:59.280
+And sometimes for brevity,
+I will also just use lowercase n
+
+55
+00:03:59.280 --> 00:04:02.720
+to represent the dimension of
+this input feature vector.
+
+56
+00:04:02.720 --> 00:04:07.510
+So in binary classification, our goal
+is to learn a classifier that can input
+
+57
+00:04:07.510 --> 00:04:10.760
+an image represented by
+this feature vector x.
+
+58
+00:04:10.760 --> 00:04:15.460
+And predict whether
+the corresponding label y is 1 or 0,
+
+59
+00:04:15.460 --> 00:04:19.000
+that is, whether this is a cat image or
+a non-cat image.
+
+60
+00:04:19.000 --> 00:04:21.560
+Let's now lay out some of
+the notation that we'll
+
+61
+00:04:21.560 --> 00:04:23.820
+use throughout the rest of this course.
+
+62
+00:04:23.820 --> 00:04:29.453
+A single training example
+is represented by a pair,
+
+63
+00:04:29.453 --> 00:04:34.446
+(x,y) where x is an x-dimensional feature
+
+64
+00:04:34.446 --> 00:04:39.320
+vector and y, the label, is either 0 or 1.
+
+65
+00:04:39.320 --> 00:04:44.550
+Your training sets will comprise
+lower-case m training examples.
+
+66
+00:04:44.550 --> 00:04:50.320
+And so your training sets will be
+written (x1, y1) which is the input and
+
+67
+00:04:50.320 --> 00:04:55.370
+output for your first training
+example (x(2), y(2)) for
+
+68
+00:04:55.370 --> 00:05:01.980
+the second training example up to 00:05:05.650
+And then that altogether is
+your entire training set.
+
+70
+00:05:05.650 --> 00:05:10.170
+So I'm going to use lowercase m to
+denote the number of training samples.
+
+71
+00:05:10.170 --> 00:05:14.418
+And sometimes to emphasize that this
+is the number of train examples,
+
+72
+00:05:14.418 --> 00:05:16.437
+I might write this as M = M train.
+
+73
+00:05:16.437 --> 00:05:18.692
+And when we talk about a test set,
+
+74
+00:05:18.692 --> 00:05:24.430
+we might sometimes use m subscript test
+to denote the number of test examples.
+
+75
+00:05:24.430 --> 00:05:27.430
+So that's the number of test examples.
+
+76
+00:05:27.430 --> 00:05:33.440
+Finally, to output all of the training
+examples into a more compact notation,
+
+77
+00:05:33.440 --> 00:05:36.840
+we're going to define a matrix, capital X.
+
+78
+00:05:36.840 --> 00:05:41.592
+As defined by taking you
+training set inputs x1, x2 and
+
+79
+00:05:41.592 --> 00:05:44.568
+so on and stacking them in columns.
+
+80
+00:05:44.568 --> 00:05:49.958
+So we take X1 and
+put that as a first column of this matrix,
+
+81
+00:05:49.958 --> 00:05:54.798
+X2, put that as a second column and
+so on down to Xm,
+
+82
+00:05:54.798 --> 00:05:58.000
+then this is the matrix capital X.
+
+83
+00:05:58.000 --> 00:06:03.005
+So this matrix X will have M columns,
+where M is the number of train
+
+84
+00:06:03.005 --> 00:06:08.665
+examples and the number of railroads,
+or the height of this matrix is NX.
+
+85
+00:06:08.665 --> 00:06:14.400
+Notice that in other causes,
+you might see the matrix capital
+
+86
+00:06:14.400 --> 00:06:19.390
+X defined by stacking up the train
+examples in rows like so,
+
+87
+00:06:19.390 --> 00:06:23.940
+X1 transpose down to Xm transpose.
+
+88
+00:06:23.940 --> 00:06:27.704
+It turns out that when you're
+implementing neural networks using
+
+89
+00:06:27.704 --> 00:06:32.218
+this convention I have on the left,
+will make the implementation much easier.
+
+90
+00:06:32.218 --> 00:06:37.171
+So just to recap,
+x is a nx by m dimensional matrix, and
+
+91
+00:06:37.171 --> 00:06:40.404
+when you implement this in Python,
+
+92
+00:06:40.404 --> 00:06:45.362
+you see that x.shape,
+that's the python command for
+
+93
+00:06:45.362 --> 00:06:50.325
+finding the shape of the matrix,
+that this an nx, m.
+
+94
+00:06:50.325 --> 00:06:53.255
+That just means it is an nx
+by m dimensional matrix.
+
+95
+00:06:53.255 --> 00:06:58.785
+So that's how you group the training
+examples, input x into matrix.
+
+96
+00:06:58.785 --> 00:07:01.315
+How about the output labels Y?
+
+97
+00:07:01.315 --> 00:07:04.815
+It turns out that to make your
+implementation of a neural network easier,
+
+98
+00:07:04.815 --> 00:07:10.030
+it would be convenient to
+also stack Y In columns.
+
+99
+00:07:10.030 --> 00:07:14.650
+So we're going to define capital
+Y to be equal to Y 1, Y 2,
+
+100
+00:07:14.650 --> 00:07:18.580
+up to Y m like so.
+
+101
+00:07:18.580 --> 00:07:24.980
+So Y here will be a 1 by
+m dimensional matrix.
+
+102
+00:07:24.980 --> 00:07:30.530
+And again, to use the notation
+without the shape of Y will be 1, m.
+
+103
+00:07:30.530 --> 00:07:34.810
+Which just means this is a 1 by m matrix.
+
+104
+00:07:34.810 --> 00:07:39.660
+And as you influence your new network,
+mtrain discourse, you find that a useful
+
+105
+00:07:39.660 --> 00:07:43.630
+convention would be to take the data
+associated with different training
+
+106
+00:07:43.630 --> 00:07:48.580
+examples, and by data I mean either x or
+y, or other quantities you see later.
+
+107
+00:07:48.580 --> 00:07:49.900
+But to take the stuff or
+
+108
+00:07:49.900 --> 00:07:52.990
+the data associated with
+different training examples and
+
+109
+00:07:52.990 --> 00:07:57.430
+to stack them in different columns,
+like we've done here for both x and y.
+
+110
+00:07:58.450 --> 00:08:01.380
+So, that's a notation we we'll use e for
+a regression and for
+
+111
+00:08:01.380 --> 00:08:04.060
+neural networks networks
+later in this course.
+
+112
+00:08:04.060 --> 00:08:07.430
+If you ever forget what a piece of
+notation means, like what is M or
+
+113
+00:08:07.430 --> 00:08:08.300
+what is N or
+
+114
+00:08:08.300 --> 00:08:12.630
+what is something else, we've also posted
+on the course website a notation guide
+
+115
+00:08:12.630 --> 00:08:17.430
+that you can use to quickly look up what
+any particular piece of notation means.
+
+116
+00:08:17.430 --> 00:08:20.890
+So with that, let's go on to the next
+video where we'll start to fetch out
+
+117
+00:08:20.890 --> 00:08:23.190
+logistic regression using this notation.
\ No newline at end of file
diff --git a/dataset/courses/Deep Learning/Study-Material/Week-2/Logistic-Regression/.DS_Store b/dataset/courses/Deep Learning/Study-Material/Week-2/Logistic-Regression/.DS_Store
new file mode 100644
index 000000000..91a0d92a8
Binary files /dev/null and b/dataset/courses/Deep Learning/Study-Material/Week-2/Logistic-Regression/.DS_Store differ
diff --git a/dataset/courses/Deep Learning/Study-Material/Week-2/Logistic-Regression/doc.pdf b/dataset/courses/Deep Learning/Study-Material/Week-2/Logistic-Regression/doc.pdf
new file mode 100644
index 000000000..e3f6676c3
Binary files /dev/null and b/dataset/courses/Deep Learning/Study-Material/Week-2/Logistic-Regression/doc.pdf differ
diff --git a/dataset/courses/Deep Learning/Study-Material/Week-2/Logistic-Regression/subtitle-en.vtt b/dataset/courses/Deep Learning/Study-Material/Week-2/Logistic-Regression/subtitle-en.vtt
new file mode 100644
index 000000000..8cc0b388e
--- /dev/null
+++ b/dataset/courses/Deep Learning/Study-Material/Week-2/Logistic-Regression/subtitle-en.vtt
@@ -0,0 +1,418 @@
+WEBVTT
+
+1
+00:00:00.000 --> 00:00:03.475
+In this video, we'll go over
+logistic regression.
+
+2
+00:00:03.475 --> 00:00:07.080
+This is a learning algorithm that you use
+when the output labels Y
+
+3
+00:00:07.080 --> 00:00:10.690
+in a supervised learning problem are all either zero or one,
+
+4
+00:00:10.690 --> 00:00:13.600
+so for binary classification problems.
+
+5
+00:00:13.600 --> 00:00:18.350
+Given an input feature vector X
+maybe corresponding to
+
+6
+00:00:18.350 --> 00:00:23.150
+an image that you want to recognize as
+either a cat picture or not a cat picture,
+
+7
+00:00:23.150 --> 00:00:26.525
+you want an algorithm that can
+output a prediction,
+
+8
+00:00:26.525 --> 00:00:28.254
+which we'll call Y hat,
+
+9
+00:00:28.254 --> 00:00:31.130
+which is your estimate of Y.
+
+10
+00:00:31.130 --> 00:00:35.896
+More formally, you want Y hat to be the
+probability of the chance that,
+
+11
+00:00:35.896 --> 00:00:40.630
+Y is equal to one given the input features X.
+
+12
+00:00:40.630 --> 00:00:43.880
+So in other words, if X is a picture,
+
+13
+00:00:43.880 --> 00:00:45.530
+as we saw in the last video,
+
+14
+00:00:45.530 --> 00:00:47.300
+you want Y hat to tell you,
+
+15
+00:00:47.300 --> 00:00:49.820
+what is the chance that this is a cat picture?
+
+16
+00:00:49.820 --> 00:00:53.420
+So X, as we said in the previous video,
+
+17
+00:00:53.420 --> 00:00:56.960
+is an X dimensional vector,
+
+18
+00:00:56.960 --> 00:01:02.000
+given that the parameters of
+logistic regression will
+
+19
+00:01:02.000 --> 00:01:07.745
+be W which is also an
+X dimensional vector,
+
+20
+00:01:07.745 --> 00:01:11.670
+together with b which is just a real number.
+
+21
+00:01:11.670 --> 00:01:16.055
+So given an input X and the
+parameters W and b,
+
+22
+00:01:16.055 --> 00:01:20.595
+how do we generate the output Y hat?
+
+23
+00:01:20.595 --> 00:01:22.970
+Well, one thing you could try,
+that doesn't work,
+
+24
+00:01:22.970 --> 00:01:27.590
+would be to have Y hat be
+w transpose X plus B,
+
+25
+00:01:27.590 --> 00:01:33.045
+kind of a linear function of the input X.
+
+26
+00:01:33.045 --> 00:01:37.145
+And in fact, this is what you use if
+you were doing linear regression.
+
+27
+00:01:37.145 --> 00:01:41.345
+But this isn't a very good algorithm
+for binary classification
+
+28
+00:01:41.345 --> 00:01:45.575
+because you want Y hat to be
+the chance that Y is equal to one.
+
+29
+00:01:45.575 --> 00:01:50.480
+So Y hat should really be
+between zero and one,
+
+30
+00:01:50.480 --> 00:01:54.697
+and it's difficult to enforce that
+because W transpose X
+
+31
+00:01:54.697 --> 00:01:58.475
+plus B can be much bigger than
+one or it can even be negative,
+
+32
+00:01:58.475 --> 00:02:00.905
+which doesn't make sense for probability.
+
+33
+00:02:00.905 --> 00:02:03.620
+That you want it to be between zero and one.
+
+34
+00:02:03.620 --> 00:02:07.670
+So in logistic regression, our output
+is instead going to be Y hat
+
+35
+00:02:07.670 --> 00:02:12.050
+equals the sigmoid function
+applied to this quantity.
+
+36
+00:02:12.050 --> 00:02:14.850
+This is what the sigmoid function looks like.
+
+37
+00:02:14.850 --> 00:02:24.000
+If on the horizontal axis I plot Z, then
+the function sigmoid of Z looks like this.
+
+38
+00:02:24.000 --> 00:02:28.050
+So it goes smoothly from zero up to one.
+
+39
+00:02:28.050 --> 00:02:30.120
+Let me label my axes here,
+
+40
+00:02:30.120 --> 00:02:34.915
+this is zero and it crosses the vertical axis as 0.5.
+
+41
+00:02:34.915 --> 00:02:41.305
+So this is what sigmoid of Z looks like. And
+we're going to use Z to denote this quantity,
+
+42
+00:02:41.305 --> 00:02:43.020
+W transpose X plus B.
+
+43
+00:02:43.020 --> 00:02:46.230
+Here's the formula for the sigmoid function.
+
+44
+00:02:46.230 --> 00:02:49.380
+Sigmoid of Z, where Z is a real number,
+
+45
+00:02:49.380 --> 00:02:52.510
+is one over one plus E to the negative Z.
+
+46
+00:02:52.510 --> 00:02:54.695
+So notice a couple of things.
+
+47
+00:02:54.695 --> 00:03:01.255
+If Z is very large, then E to the
+negative Z will be close to zero.
+
+48
+00:03:01.255 --> 00:03:03.420
+So then sigmoid of Z will be
+
+49
+00:03:03.420 --> 00:03:07.255
+approximately one over one plus
+something very close to zero,
+
+50
+00:03:07.255 --> 00:03:11.280
+because E to the negative of very
+large number will be close to zero.
+
+51
+00:03:11.280 --> 00:03:13.505
+So this is close to 1.
+
+52
+00:03:13.505 --> 00:03:16.255
+And indeed, if you look in the plot on the left,
+
+53
+00:03:16.255 --> 00:03:20.475
+if Z is very large the sigmoid of
+Z is very close to one.
+
+54
+00:03:20.475 --> 00:03:24.105
+Conversely, if Z is very small,
+
+55
+00:03:24.105 --> 00:03:28.970
+or it is a very large negative number,
+
+56
+00:03:29.180 --> 00:03:39.640
+then sigmoid of Z becomes one over
+one plus E to the negative Z,
+
+57
+00:03:39.640 --> 00:03:42.565
+and this becomes, it's a huge number.
+
+58
+00:03:42.565 --> 00:03:47.944
+So this becomes, think of it as one
+over one plus a number that is very,
+
+59
+00:03:47.944 --> 00:03:54.473
+very big, and so,
+
+60
+00:03:54.473 --> 00:03:56.570
+that's close to zero.
+
+61
+00:03:56.570 --> 00:04:00.325
+And indeed, you see that as Z becomes
+a very large negative number,
+
+62
+00:04:00.325 --> 00:04:03.505
+sigmoid of Z goes very close to zero.
+
+63
+00:04:03.505 --> 00:04:06.070
+So when you implement logistic regression,
+
+64
+00:04:06.070 --> 00:04:10.350
+your job is to try to learn
+parameters W and B so that
+
+65
+00:04:10.350 --> 00:04:15.220
+Y hat becomes a good estimate of
+the chance of Y being equal to one.
+
+66
+00:04:15.220 --> 00:04:18.955
+Before moving on, just another
+note on the notation.
+
+67
+00:04:18.955 --> 00:04:20.830
+When we programmed neural networks,
+
+68
+00:04:20.830 --> 00:04:26.855
+we'll usually keep the parameter W
+and parameter B separate,
+
+69
+00:04:26.855 --> 00:04:30.000
+where here, B corresponds to
+an inter-spectrum.
+
+70
+00:04:30.000 --> 00:04:31.295
+In some other courses,
+
+71
+00:04:31.295 --> 00:04:35.110
+you might have seen a notation
+that handles this differently.
+
+72
+00:04:35.110 --> 00:04:42.205
+In some conventions you define an extra feature
+called X0 and that equals a one.
+
+73
+00:04:42.205 --> 00:04:47.250
+So that now X is in R of NX plus one.
+
+74
+00:04:47.250 --> 00:04:53.865
+And then you define Y hat to be equal to
+sigma of theta transpose X.
+
+75
+00:04:53.865 --> 00:04:56.685
+In this alternative notational convention,
+
+76
+00:04:56.685 --> 00:05:00.510
+you have vector parameters theta,
+
+77
+00:05:00.510 --> 00:05:03.175
+theta zero, theta one, theta two,
+
+78
+00:05:03.175 --> 00:05:09.520
+down to theta NX And so,
+
+79
+00:05:09.520 --> 00:05:11.723
+theta zero, place a row a B,
+
+80
+00:05:11.723 --> 00:05:13.663
+that's just a real number,
+
+81
+00:05:13.663 --> 00:05:18.505
+and theta one down to theta NX
+play the role of W. It turns out,
+
+82
+00:05:18.505 --> 00:05:20.350
+when you implement your neural network,
+
+83
+00:05:20.350 --> 00:05:26.145
+it will be easier to just keep B and
+W as separate parameters.
+
+84
+00:05:26.145 --> 00:05:27.430
+And so, in this class,
+
+85
+00:05:27.430 --> 00:05:32.087
+we will not use any of this notational
+convention that I just wrote in red.
+
+86
+00:05:32.087 --> 00:05:36.330
+If you've not seen this notation before
+in other courses, don't worry about it.
+
+87
+00:05:36.330 --> 00:05:39.610
+It's just that for those of you that
+have seen this notation I wanted
+
+88
+00:05:39.610 --> 00:05:43.730
+to mention explicitly that we're not
+using that notation in this course.
+
+89
+00:05:43.730 --> 00:05:45.235
+But if you've not seen this before,
+
+90
+00:05:45.235 --> 00:05:48.430
+it's not important and you
+don't need to worry about it.
+
+91
+00:05:48.430 --> 00:05:52.465
+So you have now seen what the
+logistic regression model looks like.
+
+92
+00:05:52.465 --> 00:05:57.140
+Next to change the parameters W
+and B you need to define a cost function.
+
+93
+00:05:57.140 --> 00:05:58.830
+Let's do that in the next video.
\ No newline at end of file
diff --git a/dataset/courses/Deep Learning/Study-Material/Week-3/.DS_Store b/dataset/courses/Deep Learning/Study-Material/Week-3/.DS_Store
new file mode 100644
index 000000000..c51dae167
Binary files /dev/null and b/dataset/courses/Deep Learning/Study-Material/Week-3/.DS_Store differ
diff --git a/dataset/courses/Deep Learning/Study-Material/Week-3/Gradient-Decent/.DS_Store b/dataset/courses/Deep Learning/Study-Material/Week-3/Gradient-Decent/.DS_Store
new file mode 100644
index 000000000..143a933be
Binary files /dev/null and b/dataset/courses/Deep Learning/Study-Material/Week-3/Gradient-Decent/.DS_Store differ
diff --git a/dataset/courses/Deep Learning/Study-Material/Week-3/Gradient-Decent/doc.pdf b/dataset/courses/Deep Learning/Study-Material/Week-3/Gradient-Decent/doc.pdf
new file mode 100644
index 000000000..2eed539d6
Binary files /dev/null and b/dataset/courses/Deep Learning/Study-Material/Week-3/Gradient-Decent/doc.pdf differ
diff --git a/dataset/courses/Deep Learning/Study-Material/Week-3/Gradient-Decent/subtitle-en.vtt b/dataset/courses/Deep Learning/Study-Material/Week-3/Gradient-Decent/subtitle-en.vtt
new file mode 100644
index 000000000..edc1437a0
--- /dev/null
+++ b/dataset/courses/Deep Learning/Study-Material/Week-3/Gradient-Decent/subtitle-en.vtt
@@ -0,0 +1,808 @@
+WEBVTT
+
+1
+00:00:00.590 --> 00:00:03.210
+You've seen the logistic regression model.
+
+2
+00:00:03.210 --> 00:00:06.560
+You've seen the loss function that
+measures how well you're doing on
+
+3
+00:00:06.560 --> 00:00:08.780
+the single training example.
+
+4
+00:00:08.780 --> 00:00:13.530
+You've also seen the cost function that
+measures how well your parameters w and
+
+5
+00:00:13.530 --> 00:00:16.590
+b are doing on your entire training set.
+
+6
+00:00:16.590 --> 00:00:21.600
+Now let's talk about how you can use
+the gradient descent algorithm to train,
+
+7
+00:00:21.600 --> 00:00:25.730
+or to learn, the parameters w and
+b on your training set.
+
+8
+00:00:25.730 --> 00:00:30.030
+To recap, here is the familiar
+logistic regression algorithm.
+
+9
+00:00:31.130 --> 00:00:34.700
+And we have on the second
+line the cost function, J,
+
+10
+00:00:34.700 --> 00:00:37.879
+which is a function of
+your parameters w and b.
+
+11
+00:00:37.879 --> 00:00:39.960
+And that's defined as the average.
+
+12
+00:00:39.960 --> 00:00:44.140
+So it's 1 over m times the sum
+of this loss function.
+
+13
+00:00:44.140 --> 00:00:48.470
+And so the loss function
+measures how well your algorithms
+
+14
+00:00:48.470 --> 00:00:53.170
+outputs y-hat(i) on each of
+the training examples stacks up or
+
+15
+00:00:53.170 --> 00:00:58.000
+compares to the ground true label y(i)
+on each of the training examples.
+
+16
+00:00:58.000 --> 00:01:00.886
+And the full formula is
+expanded out on the right.
+
+17
+00:01:00.886 --> 00:01:04.130
+So the cost function measures
+how well your parameters w and
+
+18
+00:01:04.130 --> 00:01:06.760
+b are doing on the training set.
+
+19
+00:01:06.760 --> 00:01:11.510
+So in order to learn the set of parameters
+w and b it seems natural that we want to
+
+20
+00:01:11.510 --> 00:01:17.930
+find w and b that make the cost
+function J(w, b) as small as possible.
+
+21
+00:01:17.930 --> 00:01:21.320
+So here's an illustration
+of gradient descent.
+
+22
+00:01:21.320 --> 00:01:25.320
+In this diagram
+the horizontal axes represent
+
+23
+00:01:25.320 --> 00:01:28.510
+your spatial parameters, w and b.
+
+24
+00:01:28.510 --> 00:01:32.350
+In practice, w can be much higher
+dimensional, but for the purposes of
+
+25
+00:01:32.350 --> 00:01:38.190
+plotting, let's illustrate w as a single
+real number and b as a single real number.
+
+26
+00:01:38.190 --> 00:01:40.770
+The cost function J(w,b,) is,
+
+27
+00:01:40.770 --> 00:01:45.130
+then, some surface above these
+horizontal axes w and b.
+
+28
+00:01:45.130 --> 00:01:50.720
+So the height of the surface represents
+the value of J(w,b) at a certain point.
+
+29
+00:01:50.720 --> 00:01:55.070
+And what we want to do is really
+to find the value of w and
+
+30
+00:01:55.070 --> 00:01:59.730
+b that corresponds to the minimum
+of the cost function J.
+
+31
+00:02:00.830 --> 00:02:06.050
+It turns out that this cost
+function J is a convex function.
+
+32
+00:02:06.050 --> 00:02:10.327
+So it's just a single big bowl,
+so this is a convex function and
+
+33
+00:02:10.327 --> 00:02:13.717
+this is opposed to functions
+that look like this,
+
+34
+00:02:13.717 --> 00:02:18.120
+which are non-convex and
+has lots of different local.
+
+35
+00:02:18.120 --> 00:02:22.240
+So the fact that our cost
+function J(w,b) as defined
+
+36
+00:02:22.240 --> 00:02:27.020
+here is convex is one of the huge reasons
+why we use this particular cost function,
+
+37
+00:02:27.020 --> 00:02:29.610
+J, for logistic regression.
+
+38
+00:02:29.610 --> 00:02:33.810
+So to find a good value for
+the parameters,
+
+39
+00:02:33.810 --> 00:02:39.160
+what we'll do is initialize w and
+b to some initial value,
+
+40
+00:02:39.160 --> 00:02:43.360
+maybe denoted by that little red dot.
+
+41
+00:02:43.360 --> 00:02:47.562
+And for logistic regression almost
+any initialization method works,
+
+42
+00:02:47.562 --> 00:02:50.690
+usually you initialize the value to zero.
+
+43
+00:02:50.690 --> 00:02:52.910
+Random initialization also works, but
+
+44
+00:02:52.910 --> 00:02:55.630
+people don't usually do that for
+logistic regression.
+
+45
+00:02:55.630 --> 00:02:59.310
+But because this function is convex,
+no matter where you initialize,
+
+46
+00:02:59.310 --> 00:03:02.180
+you should get to the same point or
+roughly the same point.
+
+47
+00:03:02.180 --> 00:03:06.450
+And what gradient descent does is
+it starts at that initial point and
+
+48
+00:03:06.450 --> 00:03:10.310
+then takes a step in
+the steepest downhill direction.
+
+49
+00:03:10.310 --> 00:03:15.290
+So after one step of gradient descent
+you might end up there, because
+
+50
+00:03:15.290 --> 00:03:19.320
+it's trying to take a step downhill in
+the direction of steepest descent or
+
+51
+00:03:19.320 --> 00:03:21.250
+as quickly downhill as possible.
+
+52
+00:03:21.250 --> 00:03:23.600
+So that's one iteration
+of gradient descent.
+
+53
+00:03:23.600 --> 00:03:27.084
+And after two iterations of gradient
+descent you might step there,
+
+54
+00:03:27.084 --> 00:03:28.830
+three iterations and so on.
+
+55
+00:03:28.830 --> 00:03:32.640
+I guess this is now hidden by the back of
+the plot until eventually, hopefully you
+
+56
+00:03:32.640 --> 00:03:38.880
+converge to this global optimum or get to
+something close to the global optimum.
+
+57
+00:03:38.880 --> 00:03:42.300
+So this picture illustrates
+the gradient descent algorithm.
+
+58
+00:03:42.300 --> 00:03:44.310
+Let's write a bit more of the details.
+
+59
+00:03:44.310 --> 00:03:47.750
+For the purpose of illustration, let's
+say that there's some function, J(w),
+
+60
+00:03:47.750 --> 00:03:51.700
+that you want to minimize, and
+maybe that function looks like this.
+
+61
+00:03:51.700 --> 00:03:54.650
+To make this easier to draw,
+I'm going to ignore b for
+
+62
+00:03:54.650 --> 00:03:59.210
+now, just to make this a one-dimensional
+plot instead of a high-dimensional plot.
+
+63
+00:03:59.210 --> 00:04:01.240
+So gradient descent does this,
+
+64
+00:04:01.240 --> 00:04:06.740
+we're going to repeatedly carry
+out the following update.
+
+65
+00:04:06.740 --> 00:04:09.467
+Were going to take the value of w and
+update it,
+
+66
+00:04:09.467 --> 00:04:12.508
+going to use colon equals
+to represent updating w.
+
+67
+00:04:12.508 --> 00:04:17.426
+So set w to w minus alpha, times, and
+
+68
+00:04:17.426 --> 00:04:22.200
+this is a derivative dJ(w)/dw.
+
+69
+00:04:22.200 --> 00:04:26.230
+I will repeatedly do that
+until the algorithm converges.
+
+70
+00:04:26.230 --> 00:04:30.666
+So couple of points in the notation,
+alpha here, is the learning rate, and
+
+71
+00:04:30.666 --> 00:04:36.820
+controls how big a step we take on
+each iteration or gradient descent.
+
+72
+00:04:36.820 --> 00:04:41.200
+We'll talk later about some ways by
+choosing the learning rate alpha.
+
+73
+00:04:41.200 --> 00:04:44.490
+And second, this quantity here,
+this is a derivative.
+
+74
+00:04:44.490 --> 00:04:48.010
+This is basically the update or the change
+you want to make to the parameters w.
+
+75
+00:04:48.010 --> 00:04:52.700
+When we start to write code to
+implement gradient descent,
+
+76
+00:04:52.700 --> 00:04:57.380
+we're going to use the convention
+that the variable name in our code
+
+77
+00:04:58.620 --> 00:05:02.300
+dw will be used to represent
+this derivative term.
+
+78
+00:05:02.300 --> 00:05:06.551
+So when you write code
+you write something like
+
+79
+00:05:06.551 --> 00:05:10.046
+w colon equals w minus alpha times dw.
+
+80
+00:05:10.046 --> 00:05:14.750
+And so we use dw to be the variable
+name to represent this derivative term.
+
+81
+00:05:14.750 --> 00:05:19.330
+Now let's just make sure that this
+gradient descent update makes sense.
+
+82
+00:05:19.330 --> 00:05:21.880
+Let's say that w was over here.
+
+83
+00:05:21.880 --> 00:05:26.060
+So you're at this point on
+the cost function J(w).
+
+84
+00:05:26.060 --> 00:05:29.270
+Remember that the definition
+of a derivative
+
+85
+00:05:29.270 --> 00:05:31.420
+is the slope of a function at the point.
+
+86
+00:05:31.420 --> 00:05:36.190
+So the slope of the function is really
+the height divided by the width, right,
+
+87
+00:05:36.190 --> 00:05:40.290
+of a low triangle here at this
+tangent to J(w) at that point.
+
+88
+00:05:40.290 --> 00:05:43.900
+And so, here the derivative is positive.
+
+89
+00:05:43.900 --> 00:05:48.830
+W gets updated as w minus a learning
+rate times the derivative.
+
+90
+00:05:48.830 --> 00:05:53.310
+The derivative is positive and so
+you end up subtracting from w, so
+
+91
+00:05:53.310 --> 00:05:55.260
+you end up taking a step to the left.
+
+92
+00:05:55.260 --> 00:05:59.380
+And so gradient descent will
+make your algorithm slowly
+
+93
+00:05:59.380 --> 00:06:04.450
+decrease the parameter if you have
+started off with this large value of w.
+
+94
+00:06:04.450 --> 00:06:08.545
+As another example, if w was over here,
+
+95
+00:06:08.545 --> 00:06:15.050
+then at this point the slope here
+of dJ/dw will be negative and so
+
+96
+00:06:15.050 --> 00:06:22.771
+the gradient descent update would
+subtract alpha times a negative number.
+
+97
+00:06:22.771 --> 00:06:27.122
+And so end up slowly increasing w,
+so you end up making w bigger and
+
+98
+00:06:27.122 --> 00:06:31.530
+bigger with successive iterations and
+gradient descent.
+
+99
+00:06:31.530 --> 00:06:34.387
+So that hopefully whether you
+initialize on the left or
+
+100
+00:06:34.387 --> 00:06:39.000
+on the right gradient descent will move
+you towards this global minimum here.
+
+101
+00:06:39.000 --> 00:06:43.100
+If you're not familiar with derivates or
+with calculus and
+
+102
+00:06:43.100 --> 00:06:49.710
+what this term dJ(w)/dw means,
+don't worry too much about it.
+
+103
+00:06:49.710 --> 00:06:53.770
+We'll talk some more about
+derivatives in the next video.
+
+104
+00:06:53.770 --> 00:06:56.761
+If you have a deep knowledge of calculus,
+
+105
+00:06:56.761 --> 00:07:02.321
+you might be able to have a deeper
+intuitions about how neural networks work.
+
+106
+00:07:02.321 --> 00:07:05.471
+But even if you're not that
+familiar with calculus,
+
+107
+00:07:05.471 --> 00:07:10.091
+in the next few videos we'll give you
+enough intuitions about derivatives and
+
+108
+00:07:10.091 --> 00:07:14.980
+about calculus that you'll be able
+to effectively use neural networks.
+
+109
+00:07:14.980 --> 00:07:16.410
+But the overall intuition for
+
+110
+00:07:16.410 --> 00:07:21.520
+now is that this term represents
+the slope of the function, and
+
+111
+00:07:21.520 --> 00:07:26.760
+we want to know the slope of the function
+at the current setting of the parameters
+
+112
+00:07:26.760 --> 00:07:31.140
+so that we can take these steps of
+steepest descent, so that we know what
+
+113
+00:07:31.140 --> 00:07:35.450
+direction to step in in order to go
+downhill on the cost function J.
+
+114
+00:07:36.660 --> 00:07:42.520
+So we wrote our gradient descent for
+J(s) if only w was your parameter.
+
+115
+00:07:42.520 --> 00:07:47.150
+In logistic regression, your cost
+function is a function of both w and b.
+
+116
+00:07:47.150 --> 00:07:50.894
+So in that case, the inner loop of
+gradient descent, that is this thing here,
+
+117
+00:07:50.894 --> 00:07:53.302
+this thing you have to
+repeat becomes as follows.
+
+118
+00:07:53.302 --> 00:07:57.970
+You end up updating w as w
+minus the learning rate times
+
+119
+00:07:57.970 --> 00:08:02.030
+the derivative of J(w,b) respect to w.
+
+120
+00:08:02.030 --> 00:08:07.460
+And you update b as b minus
+the learning rate times
+
+121
+00:08:07.460 --> 00:08:12.270
+the derivative of the cost
+function in respect to b.
+
+122
+00:08:12.270 --> 00:08:17.300
+So these two equations at the bottom
+are the actual update you implement.
+
+123
+00:08:17.300 --> 00:08:22.320
+As an aside I just want to mention one
+notational convention in calculus that
+
+124
+00:08:22.320 --> 00:08:24.560
+is a bit confusing to some people.
+
+125
+00:08:24.560 --> 00:08:28.387
+I don't think it's super important
+that you understand calculus, but
+
+126
+00:08:28.387 --> 00:08:32.411
+in case you see this I want to make sure
+that you don't think too much of this.
+
+127
+00:08:32.411 --> 00:08:35.519
+Which is that in calculus, this term here,
+
+128
+00:08:35.519 --> 00:08:40.730
+we actually write as fallows,
+of that funny squiggle symbol.
+
+129
+00:08:40.730 --> 00:08:46.160
+So this symbol,
+this is actually just a lower case d
+
+130
+00:08:46.160 --> 00:08:51.070
+in a fancy font, in a stylized font for
+when you see this expression all this
+
+131
+00:08:51.070 --> 00:08:56.145
+means is this isn't [INAUDIBLE] J(w,b) or
+really the slope of the function
+
+132
+00:08:56.145 --> 00:09:01.580
+J(w,b), how much that function
+slopes in the w direction.
+
+133
+00:09:01.580 --> 00:09:06.640
+And the rule of the notation in calculus,
+which I think isn't totally logical,
+
+134
+00:09:06.640 --> 00:09:11.780
+but the rule in the notation for calculus,
+which I think just makes things much
+
+135
+00:09:11.780 --> 00:09:16.940
+more complicated than you need to be
+is that if J is a function of two or
+
+136
+00:09:16.940 --> 00:09:21.550
+more variables, then instead of using
+lowercase d you use this funny symbol.
+
+137
+00:09:21.550 --> 00:09:24.380
+This is called a partial
+derivative symbol.
+
+138
+00:09:24.380 --> 00:09:26.120
+But don't worry about this,
+
+139
+00:09:26.120 --> 00:09:31.090
+and if J is a function of only one
+variable, then you use lowercase d.
+
+140
+00:09:31.090 --> 00:09:33.960
+So the only difference between
+whether you use this funny
+
+141
+00:09:33.960 --> 00:09:38.040
+partial derivative symbol or
+lowercase d as we did on top,
+
+142
+00:09:38.040 --> 00:09:41.570
+is whether J is a function of two or
+more variables.
+
+143
+00:09:41.570 --> 00:09:45.900
+In which case, you use this symbol,
+the partial derivative symbol, or
+
+144
+00:09:45.900 --> 00:09:51.480
+if J is only a function of one
+variable then you use lower case d.
+
+145
+00:09:51.480 --> 00:09:55.410
+This is one of those funny rules
+of notation in calculus that
+
+146
+00:09:55.410 --> 00:09:58.540
+I think just make things more
+complicated than they need to be.
+
+147
+00:09:58.540 --> 00:10:03.300
+But if you see this partial derivative
+symbol all it means is you're measure
+
+148
+00:10:03.300 --> 00:10:07.290
+the slope of the function,
+with respect to one of the variables.
+
+149
+00:10:07.290 --> 00:10:12.530
+And similarly to adhere to
+the formerly correct mathematical
+
+150
+00:10:12.530 --> 00:10:18.070
+notation in calculus, because here
+J has two inputs not just one.
+
+151
+00:10:18.070 --> 00:10:22.540
+This thing at the bottom should be written
+with this partial derivative simple.
+
+152
+00:10:22.540 --> 00:10:28.290
+But it really means the same thing as,
+almost the same thing as lower case d.
+
+153
+00:10:28.290 --> 00:10:31.360
+Finally, when you implement this in code,
+
+154
+00:10:31.360 --> 00:10:36.220
+we're going to use the convention that
+this quantity, really the amount by which
+
+155
+00:10:36.220 --> 00:10:41.980
+you update w, will denote as
+the variable dw in your code.
+
+156
+00:10:41.980 --> 00:10:44.220
+And this quantity, right?
+
+157
+00:10:44.220 --> 00:10:47.230
+The amount by which you want to update b
+
+158
+00:10:47.230 --> 00:10:50.740
+will denote by the variable
+db in your code.
+
+159
+00:10:50.740 --> 00:10:55.580
+All right, so, that's how you
+can implement gradient descent.
+
+160
+00:10:55.580 --> 00:10:59.830
+Now if you haven't seen calculus for a few
+years, I know that that might seem like
+
+161
+00:10:59.830 --> 00:11:03.770
+a lot more derivatives in calculus than
+you might be comfortable with so far.
+
+162
+00:11:03.770 --> 00:11:06.330
+But if you're feeling that way,
+don't worry about it.
+
+163
+00:11:06.330 --> 00:11:10.150
+In the next video, we'll give you
+better intuition about derivatives.
+
+164
+00:11:10.150 --> 00:11:13.560
+And even without the deep mathematical
+understanding of calculus,
+
+165
+00:11:13.560 --> 00:11:16.310
+with just an intuitive
+understanding of calculus
+
+166
+00:11:16.310 --> 00:11:19.130
+you will be able to make neural
+networks work effectively.
+
+167
+00:11:19.130 --> 00:11:22.743
+So that, let's go onto the next video
+where we'll talk a little bit more about
+
+168
+00:11:22.743 --> 00:11:23.470
+derivatives.
\ No newline at end of file
diff --git a/dataset/courses/Deep Learning/Study-Material/Week-3/Parameter-vs-HyperParameter/.DS_Store b/dataset/courses/Deep Learning/Study-Material/Week-3/Parameter-vs-HyperParameter/.DS_Store
new file mode 100644
index 000000000..23ed95574
Binary files /dev/null and b/dataset/courses/Deep Learning/Study-Material/Week-3/Parameter-vs-HyperParameter/.DS_Store differ
diff --git a/dataset/courses/Deep Learning/Study-Material/Week-3/Parameter-vs-HyperParameter/doc.pdf b/dataset/courses/Deep Learning/Study-Material/Week-3/Parameter-vs-HyperParameter/doc.pdf
new file mode 100644
index 000000000..56663640a
Binary files /dev/null and b/dataset/courses/Deep Learning/Study-Material/Week-3/Parameter-vs-HyperParameter/doc.pdf differ
diff --git a/dataset/courses/Deep Learning/Study-Material/Week-3/Parameter-vs-HyperParameter/subtitle-en.vtt b/dataset/courses/Deep Learning/Study-Material/Week-3/Parameter-vs-HyperParameter/subtitle-en.vtt
new file mode 100644
index 000000000..5aecc7700
--- /dev/null
+++ b/dataset/courses/Deep Learning/Study-Material/Week-3/Parameter-vs-HyperParameter/subtitle-en.vtt
@@ -0,0 +1,737 @@
+WEBVTT
+
+1
+00:00:00.060 --> 00:00:02.669
+being effective in developing your deep
+
+2
+00:00:02.669 --> 00:00:04.380
+neural Nets requires that you not only
+
+3
+00:00:04.380 --> 00:00:06.870
+organize your parameters well but also
+
+4
+00:00:06.870 --> 00:00:09.269
+your hyper parameters so what are hyper
+
+5
+00:00:09.269 --> 00:00:11.759
+parameters let's take a look so the
+
+6
+00:00:11.759 --> 00:00:15.170
+parameters your model are W and B and
+
+7
+00:00:15.170 --> 00:00:17.820
+there are other things you need to tell
+
+8
+00:00:17.820 --> 00:00:21.720
+your learning algorithm such as the
+
+9
+00:00:21.720 --> 00:00:26.220
+learning rate alpha because on we need
+
+10
+00:00:26.220 --> 00:00:28.920
+to set alpha and that in turn will
+
+11
+00:00:28.920 --> 00:00:32.329
+determine how your parameters evolve or
+
+12
+00:00:32.329 --> 00:00:34.890
+maybe the number of iterations of
+
+13
+00:00:34.890 --> 00:00:38.190
+gradient descent you carry out your
+
+14
+00:00:38.190 --> 00:00:40.170
+learning algorithm has other you know
+
+15
+00:00:40.170 --> 00:00:42.629
+numbers that you need to set such as the
+
+16
+00:00:42.629 --> 00:00:47.340
+number of hidden layers so we call that
+
+17
+00:00:47.340 --> 00:00:50.629
+capital L or the number of hidden units
+
+18
+00:00:50.629 --> 00:00:56.039
+right such as zero and one and two and
+
+19
+00:00:56.039 --> 00:00:59.670
+so on and then you also have the choice
+
+20
+00:00:59.670 --> 00:01:03.329
+of activation function do you want to
+
+21
+00:01:03.329 --> 00:01:05.610
+use a rel you or ten age or a sigma
+
+22
+00:01:05.610 --> 00:01:06.869
+little something especially in the
+
+23
+00:01:06.869 --> 00:01:11.760
+hidden layers and so all of these things
+
+24
+00:01:11.760 --> 00:01:13.590
+are things that you need to tell your
+
+25
+00:01:13.590 --> 00:01:15.990
+learning algorithm and so these are
+
+26
+00:01:15.990 --> 00:01:19.640
+parameters that control the ultimate
+
+27
+00:01:19.640 --> 00:01:22.200
+parameters W and B and so we call all of
+
+28
+00:01:22.200 --> 00:01:25.640
+these things below hyper parameters
+
+29
+00:01:25.640 --> 00:01:29.340
+because these things like alpha the
+
+30
+00:01:29.340 --> 00:01:30.750
+learning rate the number of iterations
+
+31
+00:01:30.750 --> 00:01:32.369
+number of hidden layers and so on these
+
+32
+00:01:32.369 --> 00:01:36.000
+are all parameters that control W and B
+
+33
+00:01:36.000 --> 00:01:39.290
+so we call these things hyper parameters
+
+34
+00:01:39.290 --> 00:01:41.970
+because it is the hyper parameters that
+
+35
+00:01:41.970 --> 00:01:44.250
+you know somehow determine the final
+
+36
+00:01:44.250 --> 00:01:46.950
+value of the parameters W and B that you
+
+37
+00:01:46.950 --> 00:01:50.100
+end up with in fact deep learning has a
+
+38
+00:01:50.100 --> 00:01:53.520
+lot of different hyper parameters later
+
+39
+00:01:53.520 --> 00:01:55.470
+in the later course we'll see other
+
+40
+00:01:55.470 --> 00:01:57.899
+hyper parameters as well such as the
+
+41
+00:01:57.899 --> 00:02:05.150
+momentum term the mini batch size
+
+42
+00:02:05.150 --> 00:02:07.220
+various forms of regularization
+
+43
+00:02:07.220 --> 00:02:13.020
+parameters and so on and if none of
+
+44
+00:02:13.020 --> 00:02:14.700
+these terms at the bottom make sense yet
+
+45
+00:02:14.700 --> 00:02:16.020
+don't worry about it we'll talk about
+
+46
+00:02:16.020 --> 00:02:18.810
+them in the second course because deep
+
+47
+00:02:18.810 --> 00:02:21.870
+learning has so many hyper parameters in
+
+48
+00:02:21.870 --> 00:02:24.120
+contrast to earlier errors of machine
+
+49
+00:02:24.120 --> 00:02:26.370
+learning I'm going to try to be very
+
+50
+00:02:26.370 --> 00:02:28.890
+consistent in calling the learning rate
+
+51
+00:02:28.890 --> 00:02:31.050
+alpha a hyper parameter rather than
+
+52
+00:02:31.050 --> 00:02:33.480
+calling the parameter I think in earlier
+
+53
+00:02:33.480 --> 00:02:35.250
+eras of machine learning when we didn't
+
+54
+00:02:35.250 --> 00:02:37.920
+have so many hyper parameters most of us
+
+55
+00:02:37.920 --> 00:02:39.600
+used to be a bit slow up here and just
+
+56
+00:02:39.600 --> 00:02:42.120
+call alpha a parameter and technically
+
+57
+00:02:42.120 --> 00:02:44.610
+alpha is a parameter but is a parameter
+
+58
+00:02:44.610 --> 00:02:47.580
+that determines the real parameters our
+
+59
+00:02:47.580 --> 00:02:50.280
+childhood consistent in calling these
+
+60
+00:02:50.280 --> 00:02:51.570
+things like alpha the number of
+
+61
+00:02:51.570 --> 00:02:54.180
+iterations and so on hyper parameters so
+
+62
+00:02:54.180 --> 00:02:55.769
+when you're training a deep net for your
+
+63
+00:02:55.769 --> 00:02:57.810
+own application you find that there may
+
+64
+00:02:57.810 --> 00:02:59.940
+be a lot of possible settings for the
+
+65
+00:02:59.940 --> 00:03:01.560
+hyper parameters that you need to just
+
+66
+00:03:01.560 --> 00:03:04.440
+try out so apply deep learning today is
+
+67
+00:03:04.440 --> 00:03:07.230
+a very imperiled process where often you
+
+68
+00:03:07.230 --> 00:03:09.840
+might have an idea for example you might
+
+69
+00:03:09.840 --> 00:03:12.150
+have an idea for the best value for the
+
+70
+00:03:12.150 --> 00:03:13.549
+learning rate you might say well maybe
+
+71
+00:03:13.549 --> 00:03:16.739
+alpha equals 0.01 I want to try that
+
+72
+00:03:16.739 --> 00:03:20.670
+then you implemented try it out and then
+
+73
+00:03:20.670 --> 00:03:22.530
+see how that works and then based on
+
+74
+00:03:22.530 --> 00:03:23.910
+that outcome you might say you know what
+
+75
+00:03:23.910 --> 00:03:25.890
+I've changed online I want to increase
+
+76
+00:03:25.890 --> 00:03:28.620
+the learning rate to 0.05 and so if
+
+77
+00:03:28.620 --> 00:03:30.930
+you're not sure what's the best value
+
+78
+00:03:30.930 --> 00:03:32.970
+for the learning ready-to-use you might
+
+79
+00:03:32.970 --> 00:03:35.010
+try one value of the learning rate alpha
+
+80
+00:03:35.010 --> 00:03:37.680
+and see their cost function j go down
+
+81
+00:03:37.680 --> 00:03:39.690
+like this then you might try a larger
+
+82
+00:03:39.690 --> 00:03:41.820
+value for the learning rate alpha and
+
+83
+00:03:41.820 --> 00:03:43.650
+see the cost function blow up and
+
+84
+00:03:43.650 --> 00:03:45.060
+diverge then you might try another
+
+85
+00:03:45.060 --> 00:03:47.250
+version and see it go down really fast
+
+86
+00:03:47.250 --> 00:03:49.709
+it's inverse to higher value you might
+
+87
+00:03:49.709 --> 00:03:51.780
+try another version and see it you know
+
+88
+00:03:51.780 --> 00:03:53.670
+see the cost function J do that then
+
+89
+00:03:53.670 --> 00:03:55.530
+I'll be China so the values you might
+
+90
+00:03:55.530 --> 00:03:57.840
+say okay looks like this the value of
+
+91
+00:03:57.840 --> 00:04:00.870
+alpha gives me a pretty fast learning
+
+92
+00:04:00.870 --> 00:04:02.790
+and allows me to converge to a lower
+
+93
+00:04:02.790 --> 00:04:04.290
+cost function jennice I'm going to use
+
+94
+00:04:04.290 --> 00:04:06.720
+this value of alpha you saw in a
+
+95
+00:04:06.720 --> 00:04:08.040
+previous slide that there are a lot of
+
+96
+00:04:08.040 --> 00:04:10.170
+different hybrid parameters and it turns
+
+97
+00:04:10.170 --> 00:04:11.489
+out that when you're starting on the new
+
+98
+00:04:11.489 --> 00:04:13.830
+application I should find it very
+
+99
+00:04:13.830 --> 00:04:15.450
+difficult to know in advance exactly
+
+100
+00:04:15.450 --> 00:04:17.940
+what's the best value of the hyper
+
+101
+00:04:17.940 --> 00:04:20.580
+parameters so what often happen is you
+
+102
+00:04:20.580 --> 00:04:22.169
+just have to try out many different
+
+103
+00:04:22.169 --> 00:04:24.570
+values and go around this cycle your
+
+104
+00:04:24.570 --> 00:04:26.970
+trial some value really try five hidden
+
+105
+00:04:26.970 --> 00:04:28.440
+layers with this many number of hidden
+
+106
+00:04:28.440 --> 00:04:31.140
+units implement that see if it works and
+
+107
+00:04:31.140 --> 00:04:34.140
+then iterate so the title of this slide
+
+108
+00:04:34.140 --> 00:04:36.180
+is that apply deep learning is very
+
+109
+00:04:36.180 --> 00:04:38.340
+empirical process and empirical process
+
+110
+00:04:38.340 --> 00:04:40.740
+is maybe a fancy way of saying you just
+
+111
+00:04:40.740 --> 00:04:42.419
+have to try a lot of things and see what
+
+112
+00:04:42.419 --> 00:04:45.330
+works another effect I've seen is that
+
+113
+00:04:45.330 --> 00:04:47.190
+deep learning today is applied to so
+
+114
+00:04:47.190 --> 00:04:48.810
+many problems ranging from computer
+
+115
+00:04:48.810 --> 00:04:51.990
+vision to speech recognition to natural
+
+116
+00:04:51.990 --> 00:04:53.789
+language processing to a lot of
+
+117
+00:04:53.789 --> 00:04:55.500
+structured data applications such as
+
+118
+00:04:55.500 --> 00:04:59.250
+maybe a online advertising or web search
+
+119
+00:04:59.250 --> 00:05:02.430
+or product recommendations and so on and
+
+120
+00:05:02.430 --> 00:05:05.640
+what I've seen is that first I've seen
+
+121
+00:05:05.640 --> 00:05:08.190
+researchers from one discipline any one
+
+122
+00:05:08.190 --> 00:05:10.080
+of these try to go to a different one
+
+123
+00:05:10.080 --> 00:05:12.060
+and sometimes the intuitions about hyper
+
+124
+00:05:12.060 --> 00:05:14.400
+parameters carries over and sometimes it
+
+125
+00:05:14.400 --> 00:05:16.590
+doesn't so I often advise people
+
+126
+00:05:16.590 --> 00:05:17.849
+especially when starting on a new
+
+127
+00:05:17.849 --> 00:05:20.970
+problem to just try out a range of
+
+128
+00:05:20.970 --> 00:05:23.550
+values and see what works and then mix
+
+129
+00:05:23.550 --> 00:05:25.500
+course we'll see a systematic way we'll
+
+130
+00:05:25.500 --> 00:05:27.930
+see some systematic ways for trying out
+
+131
+00:05:27.930 --> 00:05:30.780
+a range of values all right and second
+
+132
+00:05:30.780 --> 00:05:32.070
+even if you're working on one
+
+133
+00:05:32.070 --> 00:05:33.570
+application for a long time you know
+
+134
+00:05:33.570 --> 00:05:35.220
+maybe you're working on online
+
+135
+00:05:35.220 --> 00:05:37.979
+advertising as you make progress on the
+
+136
+00:05:37.979 --> 00:05:39.930
+problem is quite possible there the best
+
+137
+00:05:39.930 --> 00:05:41.580
+value for the learning rate a number of
+
+138
+00:05:41.580 --> 00:05:43.830
+hidden units and so on might change so
+
+139
+00:05:43.830 --> 00:05:46.440
+even if you tune your system to the best
+
+140
+00:05:46.440 --> 00:05:49.229
+value of hyper parameters to daily as
+
+141
+00:05:49.229 --> 00:05:51.750
+possible you find that the best value
+
+142
+00:05:51.750 --> 00:05:53.430
+might change a year from now maybe
+
+143
+00:05:53.430 --> 00:05:55.650
+because the computer infrastructure I'd
+
+144
+00:05:55.650 --> 00:05:57.840
+be it you know CPUs or the type of GPU
+
+145
+00:05:57.840 --> 00:05:59.789
+running on or something has changed but
+
+146
+00:05:59.789 --> 00:06:01.560
+so maybe one rule of thumb is you know
+
+147
+00:06:01.560 --> 00:06:03.659
+every now and then maybe every few
+
+148
+00:06:03.659 --> 00:06:05.070
+months if you're working on a problem
+
+149
+00:06:05.070 --> 00:06:06.659
+for an extended period of time for many
+
+150
+00:06:06.659 --> 00:06:09.030
+years just try a few values for the
+
+151
+00:06:09.030 --> 00:06:10.800
+hyper parameters and double check if
+
+152
+00:06:10.800 --> 00:06:12.570
+there's a better value for the hyper
+
+153
+00:06:12.570 --> 00:06:15.150
+parameters and as you do so you slowly
+
+154
+00:06:15.150 --> 00:06:17.280
+gain intuition as well about the hyper
+
+155
+00:06:17.280 --> 00:06:18.779
+parameters that work best for your
+
+156
+00:06:18.779 --> 00:06:19.870
+problems
+
+157
+00:06:19.870 --> 00:06:21.820
+and I know that this might seem like an
+
+158
+00:06:21.820 --> 00:06:24.010
+unsatisfying part of deep learning that
+
+159
+00:06:24.010 --> 00:06:25.510
+you just have to try on all the values
+
+160
+00:06:25.510 --> 00:06:27.940
+for these hyper parameters but maybe
+
+161
+00:06:27.940 --> 00:06:30.160
+this is one area where deep learning
+
+162
+00:06:30.160 --> 00:06:32.200
+research is still advancing and maybe
+
+163
+00:06:32.200 --> 00:06:33.850
+over time we'll be able to give better
+
+164
+00:06:33.850 --> 00:06:36.190
+guidance for the best hyper parameters
+
+165
+00:06:36.190 --> 00:06:38.350
+to use but it's also possible that
+
+166
+00:06:38.350 --> 00:06:41.260
+because CPUs and GPUs and networks and
+
+167
+00:06:41.260 --> 00:06:43.630
+data says are all changing and it is
+
+168
+00:06:43.630 --> 00:06:45.910
+possible that the guidance won't to
+
+169
+00:06:45.910 --> 00:06:47.680
+converge for some time and you just need
+
+170
+00:06:47.680 --> 00:06:49.360
+to keep trying out different values and
+
+171
+00:06:49.360 --> 00:06:50.860
+evaluate them on a hold on
+
+172
+00:06:50.860 --> 00:06:52.479
+cross-validation set or something and
+
+173
+00:06:52.479 --> 00:06:54.100
+pick the value that works for your
+
+174
+00:06:54.100 --> 00:06:56.350
+problems so that was a brief discussion
+
+175
+00:06:56.350 --> 00:06:58.870
+of hyper parameters in the second course
+
+176
+00:06:58.870 --> 00:07:01.030
+we'll also give some suggestions for how
+
+177
+00:07:01.030 --> 00:07:03.280
+to systematically explore the space of
+
+178
+00:07:03.280 --> 00:07:06.040
+hyper parameters but by now you actually
+
+179
+00:07:06.040 --> 00:07:07.570
+have pretty much all the tools you need
+
+180
+00:07:07.570 --> 00:07:09.430
+to do their programming exercise before
+
+181
+00:07:09.430 --> 00:07:11.470
+you do that adjust or share view one
+
+182
+00:07:11.470 --> 00:07:14.050
+more set of ideas which is I often ask
+
+183
+00:07:14.050 --> 00:07:16.150
+what does deep learning have to do the
+
+184
+00:07:16.150 --> 00:07:18.660
+human brain
\ No newline at end of file
diff --git a/dataset/courses/Deep Learning/Study-Material/Week-4/.DS_Store b/dataset/courses/Deep Learning/Study-Material/Week-4/.DS_Store
new file mode 100644
index 000000000..9ef45a10a
Binary files /dev/null and b/dataset/courses/Deep Learning/Study-Material/Week-4/.DS_Store differ
diff --git a/dataset/courses/Deep Learning/Study-Material/Week-4/Why-is-Deep-Learning-taking-off/.DS_Store b/dataset/courses/Deep Learning/Study-Material/Week-4/Why-is-Deep-Learning-taking-off/.DS_Store
new file mode 100644
index 000000000..bc0d4a909
Binary files /dev/null and b/dataset/courses/Deep Learning/Study-Material/Week-4/Why-is-Deep-Learning-taking-off/.DS_Store differ
diff --git a/dataset/courses/Deep Learning/Study-Material/Week-4/Why-is-Deep-Learning-taking-off/doc.pdf b/dataset/courses/Deep Learning/Study-Material/Week-4/Why-is-Deep-Learning-taking-off/doc.pdf
new file mode 100644
index 000000000..38a7d70c2
Binary files /dev/null and b/dataset/courses/Deep Learning/Study-Material/Week-4/Why-is-Deep-Learning-taking-off/doc.pdf differ
diff --git a/dataset/courses/Deep Learning/Study-Material/Week-4/Why-is-Deep-Learning-taking-off/subtitle-en.vtt b/dataset/courses/Deep Learning/Study-Material/Week-4/Why-is-Deep-Learning-taking-off/subtitle-en.vtt
new file mode 100644
index 000000000..210adbf20
--- /dev/null
+++ b/dataset/courses/Deep Learning/Study-Material/Week-4/Why-is-Deep-Learning-taking-off/subtitle-en.vtt
@@ -0,0 +1,58 @@
+WEBVTT
+
+00:00:00.329 --> 00:00:28.949
+if the basic technical idea is behind deep learning behind your networks have been around for decades why are they only just now taking off in this video let's go over some of the main drivers behind the rise of deep learning because I think this will help you that the spot the best opportunities within your own organization to apply these to over the last few years a lot of people have asked me Andrew why is deep learning certainly working so well and when a marsan question this is usually the
+
+00:00:28.949 --> 00:01:02.460
+picture I draw for them let's say we plot a figure where on the horizontal axis we plot the amount of data we have for a task and let's say on the vertical axis we plot the performance on above learning algorithms such as the accuracy of our spam classifier or our ad click predictor or the accuracy of our neural net for figuring out the position of other calls for our self-driving car it turns out if you plot the performance of a traditional learning algorithm like support vector machine or logistic
+
+00:01:02.460 --> 00:01:34.820
+regression as a function of the amount of data you have you might get a curve that looks like this where the performance improves for a while as you add more data but after a while the performance you know pretty much plateaus right suppose your horizontal lines enjoy that very well you know was it they didn't know what to do with huge amounts of data and what happened in our society over the last 10 years maybe is that for a lot of problems we went from having a relatively small amount of data
+
+00:01:34.820 --> 00:02:11.129
+to having you know often a fairly large amount of data and all of this was thanks to the digitization of a society where so much human activity is now in the digital realm we spend so much time on the computers on websites on mobile apps and activities on digital devices creates data and thanks to the rise of inexpensive cameras built into our cell phones accelerometers all sorts of sensors in the Internet of Things we also just have been collecting one more and more data so over the last 20 years
+
+00:02:11.129 --> 00:02:44.580
+for a lot of applications we just accumulate a lot more data more than traditional learning algorithms were able to effectively take advantage of and what new network lead turns out that if you train a small neural net then this performance maybe looks like that if you train a somewhat larger Internet that's called as a medium-sized internet to fall in something a little bit better and if you train a very large neural net then it's the form and often just keeps getting better and better so couple
+
+00:02:44.580 --> 00:03:17.069
+observations one is if you want to hit this very high level of performance then you need two things first often you need to be able to train a big enough neural network in order to take advantage of the huge amount of data and second you need to be out here on the x axes you do need a lot of data so we often say that scale has been driving deep learning progress and by scale I mean both the size of the neural network we need just a new network a lot of hidden units a lot of parameters a lot of connections
+
+00:03:17.069 --> 00:03:49.920
+as well as scale of the data in fact today one of the most reliable ways to get better performance in the neural network is often to either train a bigger network or throw more data at it and that only works up to a point because eventually you run out of data or eventually then your network is so big that it takes too long to train but just improving scale has actually taken us a long way in the world of learning in order to make this diagram a bit more technically precise and just add a few more things I wrote the amount of data
+
+00:03:49.920 --> 00:04:29.700
+on the x-axis technically this is amount of labeled data where by label data I mean training examples we have both the input X and the label Y I went to introduce a little bit of notation that we'll use later in this course we're going to use lowercase alphabet to denote the size of my training sets or the number of training examples this lowercase M so that's the horizontal axis couple other details to this Tigger in this regime of smaller training sets the relative ordering of the algorithms is actually not very well defined so if
+
+00:04:29.700 --> 00:05:01.919
+you don't have a lot of training data is often up to your skill at hand engineering features that determines the foreman so it's quite possible that if someone training an SVM is more motivated to hand engineer features and someone training even large their own that may be in this small training set regime the SEM could do better so you know in this region to the left of the figure the relative ordering between gene algorithms is not that well defined and performance depends much more on your skill at engine features
+
+00:05:01.919 --> 00:05:34.919
+and other mobile details of the algorithms and there's only in this some big data regime very large training sets very large M regime in the right that we more consistently see largely Ronettes dominating the other approaches and so if any of your friends ask you why are known as you know taking off I would encourage you to draw this picture for them as well so I will say that in the early days in their modern rise of deep learning it was scaled data and scale of computation just our ability to Train
+
+00:05:34.919 --> 00:06:12.330
+very large dinner networks either on a CPU or GPU that enabled us to make a lot of progress but increasingly especially in the last several years we've seen tremendous algorithmic innovation as well so I also don't want to understate that interestingly many of the algorithmic innovations have been about trying to make neural networks run much faster so as a concrete example one of the huge breakthroughs in your networks has been switching from a sigmoid function which looks like this to a railer function
+
+00:06:12.330 --> 00:06:41.470
+which we talked about briefly in an early video that looks like this if you don't understand the details of one about the state don't worry about it but it turns out that one of the problems of using sigmoid functions and machine learning is that there these regions here where the slope of the function would gradient is nearly zero and so learning becomes really slow because when you implement gradient descent and gradient is zero the parameters just change very slowly and so learning is very slow
+
+00:06:41.470 --> 00:07:16.960
+whereas by changing the what's called the activation function the neural network to use this function called the value function of the rectified linear unit our elu the gradient is equal to one for all positive values of input right and so the gradient is much less likely to gradually shrink to zero and the gradient here the slope of this line is zero on the left but it turns out that just by switching to the sigmoid function to the rayleigh function has made an algorithm called gradient descent work much faster and so this is
+
+00:07:16.960 --> 00:07:51.070
+an example of maybe relatively simple algorithm in Bayesian but ultimately the impact of this algorithmic innovation was it really hope computation so the regimen quite a lot of examples like this of where we change the algorithm because it allows that code to run much faster and this allows us to train bigger neural networks or to do so the reason or multi-client even when we have a large network roam all the data the other reason that fast computation is important is that it turns out the process of training your network this is
+
+00:07:51.070 --> 00:08:24.039
+very intuitive often you have an idea for a neural network architecture and so you implement your idea and code implementing your idea then lets you run an experiment which tells you how well your neural network does and then by looking at it you go back to change the details of your new network and then you go around this circle over and over and when your new network takes a long time to Train it just takes a long time to go around this cycle and there's a huge difference in your productivity building
+
+00:08:24.039 --> 00:08:59.730
+effective neural networks when you can have an idea and try it and see the work in ten minutes or maybe ammos a day versus if you've to train your neural network for a month which sometimes does happened because you get a result back you know in ten minutes or maybe in a day you should just try a lot more ideas and be much more likely to discover in your network and it works well for your application and so faster computation has really helped in terms of speeding up the rate at which you can get an
+
+00:08:59.730 --> 00:09:36.000
+experimental result back and this has really helped both practitioners of neuro networks as well as researchers working and deep learning iterate much faster and improve your ideas much faster and so all this has also been a huge boon to the entire deep learning research community which has been incredible with just you know inventing new algorithms and making nonstop progress on that front so these are some of the forces powering the rise of deep learning but the good news is that these forces are still working powerfully to
+
+00:09:36.000 --> 00:10:09.839
+make deep learning even better Tech Data society is still throwing up one more digital data or take computation with the rise of specialized hardware like GPUs and faster networking many types of hardware I'm actually quite confident that our ability to do very large neural networks or should a computation point of view will keep on getting better and take algorithms relative learning research communities though continuously phenomenal at innovating on the algorithms front so because of this I think that we can be optimistic answer
+
+00:10:09.839 --> 00:10:22.610
+the optimistic the deep learning will keep on getting better for many years to come so that let's go on to the last video of the section where we'll talk a little bit more about what you learn from this course
\ No newline at end of file
diff --git a/dataset/courses/Deep Learning/Viva-Material/context.txt b/dataset/courses/Deep Learning/Viva-Material/context.txt
new file mode 100644
index 000000000..018e23a46
--- /dev/null
+++ b/dataset/courses/Deep Learning/Viva-Material/context.txt
@@ -0,0 +1,21 @@
+* What is Deep Learning
+
+Deep learning is a subfield of machine learning and artificial intelligence that focuses on the development of algorithms and models inspired by the structure and function of the human brain's neural networks. It aims to enable computers to learn and make intelligent decisions by simulating the way the human brain processes information.
+
+The fundamental building block of deep learning is the artificial neural network (ANN), which is a computational model composed of interconnected nodes, or artificial neurons. These neurons are organized into layers, with each layer receiving input from the previous layer and producing output for the next layer. The layers are typically divided into an input layer, one or more hidden layers, and an output layer.
+
+What sets deep learning apart from traditional machine learning approaches is the use of deep neural networks, which are neural networks with multiple hidden layers. Deep neural networks can learn complex representations and hierarchies of data, enabling them to automatically extract high-level features from raw input, such as images, text, or audio. This ability to learn hierarchical representations is one of the reasons why deep learning has achieved remarkable success in various fields.
+
+To train a deep neural network, a large dataset is typically required. The network learns by adjusting its weights and biases through a process called backpropagation, where the error between the predicted output and the actual output is propagated backward through the network, updating the parameters in a way that minimizes the error. The optimization is usually performed using gradient descent or its variants.
+
+* Logistic Regression
+
+Used for classification tasks, In logistic regression, the input variables, also known as features or predictors, are linearly combined using weights. The linear combination is then passed through the logistic function, which maps the resulting value to a probability between 0 and 1. This probability represents the likelihood of the binary outcome being one of the classes. By choosing a threshold (typically 0.5), the predicted probability can be converted into a discrete class prediction.
+
+The logistic function, also called the sigmoid function, has an S-shaped curve that allows logistic regression to model non-linear relationships between the input variables and the binary outcome. This flexibility makes logistic regression suitable for a wide range of classification problems.
+
+* Gradient Descent
+
+Gradient descent is an optimization algorithm commonly used in machine learning and mathematical optimization. Its purpose is to minimize or maximize a given function by iteratively adjusting the parameters or weights of a model in the direction of steepest descent or ascent, respectively.
+
+The name "gradient descent" stems from the fact that it relies on the gradient of the function being optimized. The gradient is a vector that points in the direction of the greatest increase of the function. By taking steps in the opposite direction of the gradient, the algorithm moves towards the minimum (or maximum) of the function.
\ No newline at end of file
diff --git a/dataset/courses/Deep Learning/deep_learning_banner.jpeg b/dataset/courses/Deep Learning/deep_learning_banner.jpeg
new file mode 100644
index 000000000..501840a01
Binary files /dev/null and b/dataset/courses/Deep Learning/deep_learning_banner.jpeg differ
diff --git a/dataset/courses/Machine Learning/.DS_Store b/dataset/courses/Machine Learning/.DS_Store
new file mode 100644
index 000000000..8435191e7
Binary files /dev/null and b/dataset/courses/Machine Learning/.DS_Store differ
diff --git a/dataset/courses/Machine Learning/Study-Material/.DS_Store b/dataset/courses/Machine Learning/Study-Material/.DS_Store
new file mode 100644
index 000000000..5233fc361
Binary files /dev/null and b/dataset/courses/Machine Learning/Study-Material/.DS_Store differ
diff --git a/dataset/courses/Machine Learning/Study-Material/Week-1/.gitignore b/dataset/courses/Machine Learning/Study-Material/Week-1/.gitignore
new file mode 100644
index 000000000..e69de29bb
diff --git a/dataset/courses/Machine Learning/Viva-Material/.gitignore b/dataset/courses/Machine Learning/Viva-Material/.gitignore
new file mode 100644
index 000000000..e69de29bb
diff --git a/dataset/courses/Machine Learning/machine_learning_banner.jpeg b/dataset/courses/Machine Learning/machine_learning_banner.jpeg
new file mode 100644
index 000000000..cf30d71b9
Binary files /dev/null and b/dataset/courses/Machine Learning/machine_learning_banner.jpeg differ
diff --git a/docker-compose.yml b/docker-compose.yml
new file mode 100644
index 000000000..772538745
--- /dev/null
+++ b/docker-compose.yml
@@ -0,0 +1,20 @@
+version: '3.4'
+services:
+ leap-api:
+ restart: always
+ build:
+ context: "./api/."
+ dockerfile: ./Dockerfile
+ container_name: leap-api
+ ports:
+ - "8500:8500"
+ leap-webapp:
+ restart: always
+ build:
+ context: "./webapp/."
+ dockerfile: ./Dockerfile
+ container_name: leap-webapp
+ ports:
+ - "8502:8502"
+ depends_on:
+ - leap-api
\ No newline at end of file
diff --git a/nlp/.DS_Store b/nlp/.DS_Store
new file mode 100644
index 000000000..63eaee108
Binary files /dev/null and b/nlp/.DS_Store differ
diff --git a/nlp/README.md b/nlp/README.md
new file mode 100644
index 000000000..0f83ed5b3
--- /dev/null
+++ b/nlp/README.md
@@ -0,0 +1,22 @@
+
+## Preprocess course dataset (To maintain minimum text length of each subtitle)
+
+- Run the `preprocess_subtitle.py` script
+
+```python
+ $ cd utils
+
+ $ python preprocess_subtitle.py --course_dir=../../dataset/courses --min_text_len=500
+```
+
+## Perform Basic EDA on course dataset
+
+- Run the `run_eda.py` script
+
+```python
+ $ cd utils
+
+ $ python run_eda.py --course_dir=../../dataset/courses
+```
+
+
diff --git a/nlp/__init__.py b/nlp/__init__.py
new file mode 100644
index 000000000..e69de29bb
diff --git a/nlp/feature_extractor/run_benchmark_tfidf.py b/nlp/feature_extractor/run_benchmark_tfidf.py
new file mode 100644
index 000000000..79d828e56
--- /dev/null
+++ b/nlp/feature_extractor/run_benchmark_tfidf.py
@@ -0,0 +1,161 @@
+#!/usr/bin/env python
+# coding=utf-8
+# Copyright 2023 C5ailabs Team (Authors: Rohit Sroch) All rights reserved.
+#
+# Licensed under the Apache License, Version 2.0 (the "License");
+# you may not use this file except in compliance with the License.
+# You may obtain a copy of the License at
+#
+# http://www.apache.org/licenses/LICENSE-2.0
+#
+# Unless required by applicable law or agreed to in writing, software
+# distributed under the License is distributed on an "AS IS" BASIS,
+# WITHOUT WARRANTIES OR CONDITIONS OF ANY KIND, either express or implied.
+# See the License for the specific language governing permissions and
+# limitations under the License.
+"""
+Run a retriever benchmark (TFIDFEmbeddings) Intel® Extension for Scikit-Learn vs Vanilla Scikit-Learn
+"""
+import argparse
+import os
+import timeit
+import numpy as np
+import webvtt
+from glob import glob
+
+from tfidf import TFIDFEmbeddings
+
+def get_size(path):
+ """A simple function gets the size from a path"""
+ return str(round(os.path.getsize(path)/(1024*1024), 3))
+
+def str_np(iterable):
+ """A simple function results printable value"""
+ return str(np.median(iterable).round(5)), str(np.std(iterable).round(5))
+
+def get_subtitles_as_docs(course_dir):
+ # format of courses folder structure is courses/{topic_name}/Study-Material/{week_name}/{subtopic_name}/subtitle-en.vtt
+ path = os.path.join(course_dir, "*/Study-Material/*/*/*.vtt")
+ subtitle_fpaths = glob(path)
+
+ docs = []
+ for subtitle_fpath in subtitle_fpaths:
+ subtitles = webvtt.read(subtitle_fpath)
+ for index, subtitle in enumerate(subtitles):
+ docs.append(subtitle.text)
+
+ return docs
+
+def train_tfidf_emb_model(tfidf_emb_model, texts):
+ """using .fit_transform"""
+ def warmup(inputs):
+ for _ in range(100): tfidf_emb_model.embed_documents(
+ inputs, is_preprocess=args.is_preprocess)
+ # perform warmup before running inference
+ warmup([texts[0]])
+
+ def run_train():
+ tfidf_emb_model.embed_documents(
+ texts, is_preprocess=args.is_preprocess)
+
+ output = str_np(timeit.repeat(
+ stmt="""run_train()""", number=1, repeat=50, globals=locals()))
+
+ benchmark = {"avg_time(sec)": output[0], "std_time(sec)": output[1]}
+
+ return benchmark
+
+def infer_tfidf_emb_model(tfidf_emb_model, texts, batch_size=8):
+ """using .transform"""
+
+ tfidf_emb_model.embed_documents(texts, is_preprocess=args.is_preprocess)
+ num_batches = int(len(texts) / batch_size)
+ def warmup(inputs):
+ for _ in range(100): tfidf_emb_model.embed_queries(
+ inputs, is_preprocess=args.is_preprocess)
+ # perform warmup before running inference
+ warmup([texts[0]])
+
+ def run_infer():
+ for index in range(num_batches):
+ batch_texts = texts[index * batch_size: (index + 1) * batch_size]
+ #if len(batch_texts) > 0:
+ tfidf_emb_model.embed_queries(batch_texts, is_preprocess=args.is_preprocess)
+
+ output = str_np(timeit.repeat(
+ stmt="""run_infer()""", number=1, repeat=50, globals=locals()))
+
+ benchmark = {"avg_time(sec)": output[0], "std_time(sec)": output[1]}
+
+ return benchmark
+
+
+def main(args):
+
+ print("*********Enable Intel® Extension for Scikit-Learn: {}*********".format(
+ args.intel_scikit_learn_enabled
+ ))
+ # get the contexts as docs with metadata
+ docs = get_subtitles_as_docs(args.course_dir)
+ docs = docs[: args.max_samples]
+
+ # load tfidf emb model
+ tfidf_emb_model = TFIDFEmbeddings(
+ intel_scikit_learn_enabled=args.intel_scikit_learn_enabled
+ )
+
+ print("*"*100)
+ print("*********Training TFIDFVectorizer Benchmark (.fit_transform func)*********")
+ benchmark = train_tfidf_emb_model(tfidf_emb_model, docs)
+ trainTime = float(benchmark["avg_time(sec)"])
+ print("Train time: %.3f ms" % (trainTime * 1000))
+
+ print("*"*100)
+ print("*********Inference TFIDFVectorizer Benchmark (.transform func)*********")
+ benchmark = infer_tfidf_emb_model(tfidf_emb_model, docs, args.batch_size)
+
+ eval_samples = min(args.max_samples, len(docs))
+ samples = eval_samples - (eval_samples % args.batch_size)
+ evalTime = float(benchmark["avg_time(sec)"])
+ print('Batch size = %d' % args.batch_size)
+ print("Latency: %.3f ms" % (evalTime / samples * 1000))
+ print("Throughput: {} samples/sec".format(samples / evalTime))
+
+
+
+if __name__ == "__main__":
+
+ parser = argparse.ArgumentParser(description="Run a retriever benchmark (TFIDFEmbeddings)")
+
+ parser.add_argument(
+ "--course_dir",
+ type=str,
+ help="Base directory containing courses",
+ required=True
+ )
+ parser.add_argument(
+ "--intel_scikit_learn_enabled",
+ action="store_true",
+ help="Whether to use intel extension for scikit learn in case of emb_model_type as syntactic",
+ )
+ parser.add_argument(
+ "--max_samples",
+ type=int,
+ help="Maximum samples considered for benchmark",
+ default=300
+ )
+ parser.add_argument(
+ "--batch_size",
+ type=int,
+ help="Batch size for benchmark",
+ default=8
+ )
+ parser.add_argument(
+ "--is_preprocess",
+ action="store_true",
+ help="Whether to preprocess text",
+ )
+
+ args = parser.parse_args()
+
+ main(args)
diff --git a/nlp/feature_extractor/tfidf.py b/nlp/feature_extractor/tfidf.py
new file mode 100644
index 000000000..520d9349b
--- /dev/null
+++ b/nlp/feature_extractor/tfidf.py
@@ -0,0 +1,166 @@
+"""Wrapper around sklearn TF-IDF vectorizer"""
+from __future__ import annotations
+
+import os
+import pickle
+from collections import defaultdict
+from abc import ABC, abstractmethod
+from typing import List
+
+from typing import Any, Dict, List, Optional
+from pydantic import BaseModel, Field
+
+try:
+ from nltk.tokenize import word_tokenize
+ from nltk import pos_tag
+ from nltk.corpus import stopwords
+ from nltk.stem import WordNetLemmatizer
+ from nltk.corpus import wordnet as wn
+except ImportError as exc:
+ raise ImportError(
+ "Could not import nltk python package. "
+ "Please install it with `pip install nltk`."
+ ) from exc
+
+class Embeddings(ABC):
+ """Interface for embedding models."""
+
+ @abstractmethod
+ def embed_documents(self, texts: List[str]) -> List[List[float]]:
+ """Embed search docs."""
+
+ @abstractmethod
+ def embed_query(self, text: str) -> List[float]:
+ """Embed query text."""
+
+class TFIDFEmbeddings(BaseModel, Embeddings):
+ """Wrapper around sklearn TFIDF vectorizer."""
+
+ vectorizer: Any #: :meta private:
+
+ intel_scikit_learn_enabled: Optional[bool] = True
+ """Key word arguments to pass to the model."""
+ tfidf_kwargs: Dict[str, Any] = Field(default_factory=dict)
+
+ def __init__(self, **kwargs: Any):
+ """Initialize the sentence_transformer."""
+ super().__init__(**kwargs)
+ try:
+ if self.intel_scikit_learn_enabled:
+ # Turn on scikit-learn optimizations with these 2 simple lines:
+ from sklearnex import patch_sklearn
+ patch_sklearn()
+ else:
+ # Turn off scikit-learn optimizations with these 2 simple lines:
+ from sklearnex import unpatch_sklearn
+ unpatch_sklearn()
+
+ from sklearn.feature_extraction.text import TfidfVectorizer
+ except ImportError as exc:
+ raise ImportError(
+ "Could not import scikit-learn and scikit-learn-intelex python package. "
+ "Please install it with `pip install scikit-learn scikit-learn-intelex`."
+ ) from exc
+
+ if self.tfidf_kwargs.get("tfidf_vocab_path", None) is not None:
+ print("******Loading tfidf_vocab.pkl ********")
+ path = os.path.join(self.tfidf_kwargs.get("tfidf_vocab_path"), "tfidf_vocab.pkl")
+
+ with open(path, "rb") as fp:
+ tfidf_vocab = pickle.load(fp)
+ self.tfidf_kwargs["vocabulary"] = tfidf_vocab
+ self.tfidf_kwargs.pop("tfidf_vocab_path")
+
+ self.vectorizer = TfidfVectorizer(**self.tfidf_kwargs)
+
+ class Config:
+ """Configuration for this pydantic object."""
+
+ arbitrary_types_allowed = True
+
+ def save_tfidf_vocab(self, tfidf_vocab, save_path):
+ """save the tfidf vectorizer object"""
+
+ path = os.path.join(save_path, "tfidf_vocab.pkl")
+ with open(path, "wb") as f:
+ pickle.dump(tfidf_vocab, f)
+
+ def embed_documents(self, texts: List[str], is_preprocess: bool=False) -> List[List[float]]:
+ """Compute doc embeddings.
+
+ Args:
+ texts: The list of texts to embed.
+
+ Returns:
+ List of embeddings, one for each text.
+ """
+ if is_preprocess:
+ texts = list(map(lambda x: self._preprocess_query(x.replace("\n", " ")), texts))
+ else:
+ texts = list(map(lambda x: x.replace("\n", " "), texts))
+ embeddings = self.vectorizer.fit_transform(texts) # .fit_transform
+ embeddings = [emb.toarray().astype("float32")[0].tolist() for emb in embeddings]
+
+ return embeddings
+
+ def embed_queries(self, texts: List[str], is_preprocess: bool=False) -> List[List[float]]:
+ """Compute queries embeddings.
+
+ Args:
+ texts: The list of texts to embed.
+
+ Returns:
+ List of embeddings, one for each text.
+ """
+ if is_preprocess:
+ texts = list(map(lambda x: self._preprocess_query(x.replace("\n", " ")), texts))
+ else:
+ texts = list(map(lambda x: x.replace("\n", " "), texts))
+ embeddings = self.vectorizer.transform(texts) # .transform
+ embeddings = [emb.toarray().astype("float32")[0].tolist() for emb in embeddings]
+
+ return embeddings
+
+ def embed_query(self, text: str, is_preprocess: bool=False) -> List[float]:
+ """Compute query embedding.
+
+ Args:
+ text: The text to embed.
+
+ Returns:
+ Embeddings for the text.
+ """
+ if is_preprocess:
+ text = self._preprocess_query(text.replace("\n", " "))
+ else:
+ text = text.replace("\n", " ")
+ embedding = self.vectorizer.transform([text]).toarray().astype("float32")[0] # .transform
+
+ return embedding.tolist()
+
+ def _preprocess_query(self, query):
+ """preprocess the query"""
+
+ # Next change is lower case using apply and lambda function
+ query_transformed = word_tokenize(query)
+ # Now to remove stopwords, lemmatisation and stemming
+ # We need p.o.s (part of speech) tags to understand if its a noun or a verb
+ tag_map = defaultdict(lambda: wn.NOUN)
+ tag_map['J'] = wn.ADJ
+ tag_map['V'] = wn.VERB
+ tag_map['R'] = wn.ADV
+
+ _stopwords = stopwords.words('english')
+ _query = ""
+ # Instantiate the lemmatizer
+ word_lem = WordNetLemmatizer()
+ for word, tag in pos_tag(query_transformed):
+ # Loop over the entry in the text column.
+ # If the word is not in the stopword
+ if word not in _stopwords and (word.isalpha() or word.isalnum() or word.isnumeric()):
+ # Run our lemmatizer on the word.
+ word = str(word_lem.lemmatize(word, tag_map[tag[0]]))
+ # Now add to final words
+ _query += word + " "
+
+ return _query.strip()
\ No newline at end of file
diff --git a/nlp/question_answering/__init__.py b/nlp/question_answering/__init__.py
new file mode 100644
index 000000000..e69de29bb
diff --git a/nlp/question_answering/artifacts/.gitignore b/nlp/question_answering/artifacts/.gitignore
new file mode 100644
index 000000000..e69de29bb
diff --git a/nlp/question_answering/finetune_qa.sh b/nlp/question_answering/finetune_qa.sh
new file mode 100644
index 000000000..70d2685c9
--- /dev/null
+++ b/nlp/question_answering/finetune_qa.sh
@@ -0,0 +1,73 @@
+#!/bin/bash
+HOME_DIR=`pwd`
+
+##############################################################################################
+######################### Finetune Transformer Model for QA ###############################
+##############################################################################################
+
+export MODEL_NAME_OR_PATH=ai4bharat/indic-bert
+export BACKBONE_NAME=indic-mALBERT-uncased
+export DATASET_NAME=squad # squad, squad_v2 (pass --version_2_with_negative)
+export TASK_NAME=qa
+
+# hyperparameters
+export SEED=42
+export BATCH_SIZE=32
+export MAX_SEQ_LENGTH=384
+export NUM_TRAIN_EPOCHS=5
+export WEIGHT_DECAY=0.0
+export LEARNING_RATE=5e-5
+export LR_SCHEDULER_TYPE=linear
+export WARMUP_RATIO=0.0
+export NUM_WARMUP_STEPS= 365
+export DOC_STRIDE=128
+export OPTIMIZER=adamw_hf # adamw_hf, adamw_torch, adamw_torch_fused, adamw_torch_xla, adamw_apex_fused, adafactor, adamw_anyprecision, sgd, adagrad
+export LOGGING_STRATEGY=epoch # no, steps, epoch
+export EVALUATION_STRATEGY=epoch # no, steps, epoch
+export SAVE_STRATEGY=epoch # no, steps, epoch
+export SAVE_TOTAL_LIMIT=1
+export KEEP_ACCENTS=True
+export DO_LOWER_CASE=True
+
+# other parameters
+export RUN_NAME=finetuning
+export REPORT_TO=mlflow
+
+export ENABLE_FP16=True
+export ENABLE_BF16=False
+export ENABLE_IPEX=False
+
+export OUTPUT_DIR=artifacts/$TASK_NAME/$DATASET_NAME/$BACKBONE_NAME
+export RUN_NAME=$BACKBONE_NAME-$DATASET_NAME-$TASK_NAME-$SEED
+
+python run_qa_finetune.py \
+ --do_train \
+ --do_eval \
+ --overwrite_output_dir \
+ --model_name_or_path $MODEL_NAME_OR_PATH \
+ --dataset_name $DATASET_NAME \
+ --logging_strategy $LOGGING_STRATEGY \
+ --evaluation_strategy $EVALUATION_STRATEGY \
+ --save_strategy $SAVE_STRATEGY \
+ --save_total_limit $SAVE_TOTAL_LIMIT \
+ --keep_accents $KEEP_ACCENTS \
+ --do_lower_case $DO_LOWER_CASE \
+ --optim $OPTIMIZER \
+ --weight_decay $WEIGHT_DECAY \
+ --per_device_train_batch_size $BATCH_SIZE \
+ --per_device_eval_batch_size $BATCH_SIZE \
+ --gradient_accumulation_steps 1 \
+ --learning_rate $LEARNING_RATE \
+ --lr_scheduler_type $LR_SCHEDULER_TYPE \
+ --warmup_ratio $WARMUP_RATIO \
+ --warmup_steps $NUM_WARMUP_STEPS \
+ --num_train_epochs $NUM_TRAIN_EPOCHS \
+ --max_seq_length $MAX_SEQ_LENGTH \
+ --doc_stride $DOC_STRIDE \
+ --seed $SEED \
+ --output_dir $OUTPUT_DIR \
+ --run_name $RUN_NAME \
+ --report_to $REPORT_TO \
+ --fp16 $ENABLE_FP16 \
+ --bf16 $ENABLE_BF16 \
+ --use_ipex $ENABLE_IPEX
\ No newline at end of file
diff --git a/nlp/question_answering/pot_benchmark_qa.sh b/nlp/question_answering/pot_benchmark_qa.sh
new file mode 100644
index 000000000..0fb099b24
--- /dev/null
+++ b/nlp/question_answering/pot_benchmark_qa.sh
@@ -0,0 +1,86 @@
+#!/bin/bash
+HOME_DIR=`pwd`
+
+##############################################################################################
+######## Post Training Optimization (POT) and Benchmark Transformer Model for QA ##########
+##############################################################################################
+
+export MODEL_NAME_OR_PATH=artifacts/qa/squad/indic-mALBERT
+export BACKBONE_NAME=indic-mALBERT-uncased
+export DATASET_NAME=squad # squad, squad_v2 (pass --version_2_with_negative)
+export TASK_NAME=qa
+export USE_OPTIMUM=True # whether to use hugging face wrapper optimum around intel neural compressor
+
+# other parameters
+export BATCH_SIZE=8
+export MAX_SEQ_LENGTH=384
+export DOC_STRIDE=128
+export KEEP_ACCENTS=True
+export DO_LOWER_CASE=True
+export MAX_EVAL_SAMPLES=200
+
+export TUNE=True
+export PTQ_METHOD="static_int8" # "dynamic_int8", "static_int8", "static_smooth_int8"
+export BACKEND="default" # default, ipex
+export ITERS=100
+
+export INT8=False
+if [[ ${TUNE} == "True" ]]; then
+ export INT8=True # if tune is True then int8 must be true
+fi
+
+export PRECISION=fp32
+if [[ ${INT8} == "True" ]]; then
+ export PRECISION=int8
+fi
+
+if [[ ${PRECISION} == "fp32" ]]; then
+ if [[ ${BACKEND} == "ipex" ]]; then
+ export OUTPUT_DIR=tuned/$TASK_NAME/$DATASET_NAME/$BACKBONE_NAME/$BACKEND/$PRECISION
+ echo Perform Optimization using IPEX: PRECISION=$PRECISION
+ else
+ export OUTPUT_DIR=tuned/$TASK_NAME/$DATASET_NAME/$BACKBONE_NAME/$BACKEND/$PRECISION
+ echo Base Pytorch with no Optimization: PRECISION=$PRECISION
+ fi
+else
+ export OUTPUT_DIR=tuned/$TASK_NAME/$DATASET_NAME/$BACKBONE_NAME/$BACKEND/$PTQ_METHOD/$PRECISION
+ echo Perform Quantization using Neural Compressor with: Tune=$TUNE, PTQ_METHOD=$PTQ_METHOD, BACKEND=$BACKEND, INT8=$INT8, PRECISION=$PRECISION
+fi
+
+if [[ ${USE_OPTIMUM} == "True" ]]; then
+ python -u run_qa_pot_optimum.py \
+ --model_name_or_path $MODEL_NAME_OR_PATH \
+ --dataset_name $DATASET_NAME \
+ --max_seq_length $MAX_SEQ_LENGTH \
+ --per_device_eval_batch_size $BATCH_SIZE \
+ --keep_accents $KEEP_ACCENTS \
+ --do_lower_case $DO_LOWER_CASE \
+ --max_eval_samples $MAX_EVAL_SAMPLES \
+ --doc_stride $DOC_STRIDE \
+ --tune $TUNE \
+ --ptq_method $PTQ_METHOD \
+ --int8 $INT8 \
+ --backend $BACKEND \
+ --iters $ITERS \
+ --benchmark \
+ --no_cuda \
+ --output_dir $OUTPUT_DIR
+else
+ python -u run_qa_pot.py \
+ --model_name_or_path $MODEL_NAME_OR_PATH \
+ --dataset_name $DATASET_NAME \
+ --max_seq_length $MAX_SEQ_LENGTH \
+ --per_device_eval_batch_size $BATCH_SIZE \
+ --keep_accents $KEEP_ACCENTS \
+ --do_lower_case $DO_LOWER_CASE \
+ --max_eval_samples $MAX_EVAL_SAMPLES \
+ --doc_stride $DOC_STRIDE \
+ --tune $TUNE \
+ --ptq_method $PTQ_METHOD \
+ --int8 $INT8 \
+ --backend $BACKEND \
+ --iters $ITERS \
+ --benchmark \
+ --no_cuda \
+ --output_dir $OUTPUT_DIR
+fi
\ No newline at end of file
diff --git a/nlp/question_answering/run_qa_finetune.py b/nlp/question_answering/run_qa_finetune.py
new file mode 100644
index 000000000..b89acb7e1
--- /dev/null
+++ b/nlp/question_answering/run_qa_finetune.py
@@ -0,0 +1,703 @@
+#!/usr/bin/env python
+# coding=utf-8
+# Copyright 2020 The HuggingFace Team All rights reserved.
+#
+# Licensed under the Apache License, Version 2.0 (the "License");
+# you may not use this file except in compliance with the License.
+# You may obtain a copy of the License at
+#
+# http://www.apache.org/licenses/LICENSE-2.0
+#
+# Unless required by applicable law or agreed to in writing, software
+# distributed under the License is distributed on an "AS IS" BASIS,
+# WITHOUT WARRANTIES OR CONDITIONS OF ANY KIND, either express or implied.
+# See the License for the specific language governing permissions and
+# limitations under the License.
+"""
+Fine-tuning a 🤗 Transformers model for question answering using 💻 IPEX (Intel® Extension for PyTorch) and 🤖 MLFlow.
+"""
+# You can also adapt this script on your own question answering task. Pointers for this are left as comments.
+
+import logging
+import os
+import timeit
+import sys
+from dataclasses import dataclass, field
+from typing import Optional
+
+import datasets
+import evaluate
+from datasets import load_dataset
+from trainer_qa import QuestionAnsweringTrainer
+from utils_qa import postprocess_qa_predictions
+
+import transformers
+from transformers import (
+ AutoConfig,
+ AutoModelForQuestionAnswering,
+ AutoTokenizer,
+ DataCollatorWithPadding,
+ EvalPrediction,
+ HfArgumentParser,
+ PreTrainedTokenizerFast,
+ TrainingArguments,
+ default_data_collator,
+ set_seed,
+)
+from transformers.trainer_utils import get_last_checkpoint
+from transformers.utils import check_min_version
+from transformers.utils.versions import require_version
+
+os.environ["TOKENIZERS_PARALLELISM"] = "false"
+# Will error if the minimal version of Transformers is not installed. Remove at your own risks.
+check_min_version("4.29.0")
+
+require_version("datasets>=1.8.0", "To fix: pip install datasets")
+
+logger = logging.getLogger(__name__)
+
+
+@dataclass
+class ModelArguments:
+ """
+ Arguments pertaining to which model/config/tokenizer we are going to fine-tune from.
+ """
+
+ model_name_or_path: str = field(
+ metadata={"help": "Path to pretrained model or model identifier from huggingface.co/models"}
+ )
+ config_name: Optional[str] = field(
+ default=None, metadata={"help": "Pretrained config name or path if not the same as model_name"}
+ )
+ tokenizer_name: Optional[str] = field(
+ default=None, metadata={"help": "Pretrained tokenizer name or path if not the same as model_name"}
+ )
+ cache_dir: Optional[str] = field(
+ default=".cache",
+ metadata={"help": "Path to directory to store the pretrained models downloaded from huggingface.co"},
+ )
+ model_revision: str = field(
+ default="main",
+ metadata={"help": "The specific model version to use (can be a branch name, tag name or commit id)."},
+ )
+ use_auth_token: bool = field(
+ default=False,
+ metadata={
+ "help": (
+ "Will use the token generated when running `huggingface-cli login` (necessary to use this script "
+ "with private models)."
+ )
+ },
+ )
+ keep_accents: bool = field(
+ default=False,
+ metadata={
+ "help": "To preserve accents (vowel matras / diacritics) while tokenization"
+
+ },
+ )
+ do_lower_case: bool = field(
+ default=False,
+ metadata={
+ "help": "Whether to lower case while tokenization"
+
+ },
+ )
+
+@dataclass
+class DataTrainingArguments:
+ """
+ Arguments pertaining to what data we are going to input our model for training and eval.
+ """
+
+ dataset_name: Optional[str] = field(
+ default=None, metadata={"help": "The name of the dataset to use (via the datasets library)."}
+ )
+ dataset_config_name: Optional[str] = field(
+ default=None, metadata={"help": "The configuration name of the dataset to use (via the datasets library)."}
+ )
+ train_file: Optional[str] = field(default=None, metadata={"help": "The input training data file (a text file)."})
+ validation_file: Optional[str] = field(
+ default=None,
+ metadata={"help": "An optional input evaluation data file to evaluate the perplexity on (a text file)."},
+ )
+ test_file: Optional[str] = field(
+ default=None,
+ metadata={"help": "An optional input test data file to evaluate the perplexity on (a text file)."},
+ )
+ overwrite_cache: bool = field(
+ default=False, metadata={"help": "Overwrite the cached training and evaluation sets"}
+ )
+ preprocessing_num_workers: Optional[int] = field(
+ default=None,
+ metadata={"help": "The number of processes to use for the preprocessing."},
+ )
+ max_seq_length: int = field(
+ default=384,
+ metadata={
+ "help": (
+ "The maximum total input sequence length after tokenization. Sequences longer "
+ "than this will be truncated, sequences shorter will be padded."
+ )
+ },
+ )
+ pad_to_max_length: bool = field(
+ default=True,
+ metadata={
+ "help": (
+ "Whether to pad all samples to `max_seq_length`. If False, will pad the samples dynamically when"
+ " batching to the maximum length in the batch (which can be faster on GPU but will be slower on TPU)."
+ )
+ },
+ )
+ max_train_samples: Optional[int] = field(
+ default=None,
+ metadata={
+ "help": (
+ "For debugging purposes or quicker training, truncate the number of training examples to this "
+ "value if set."
+ )
+ },
+ )
+ max_eval_samples: Optional[int] = field(
+ default=None,
+ metadata={
+ "help": (
+ "For debugging purposes or quicker training, truncate the number of evaluation examples to this "
+ "value if set."
+ )
+ },
+ )
+ max_predict_samples: Optional[int] = field(
+ default=None,
+ metadata={
+ "help": (
+ "For debugging purposes or quicker training, truncate the number of prediction examples to this "
+ "value if set."
+ )
+ },
+ )
+ version_2_with_negative: bool = field(
+ default=False, metadata={"help": "If true, some of the examples do not have an answer."}
+ )
+ null_score_diff_threshold: float = field(
+ default=0.0,
+ metadata={
+ "help": (
+ "The threshold used to select the null answer: if the best answer has a score that is less than "
+ "the score of the null answer minus this threshold, the null answer is selected for this example. "
+ "Only useful when `version_2_with_negative=True`."
+ )
+ },
+ )
+ doc_stride: int = field(
+ default=128,
+ metadata={"help": "When splitting up a long document into chunks, how much stride to take between chunks."},
+ )
+ n_best_size: int = field(
+ default=20,
+ metadata={"help": "The total number of n-best predictions to generate when looking for an answer."},
+ )
+ max_answer_length: int = field(
+ default=30,
+ metadata={
+ "help": (
+ "The maximum length of an answer that can be generated. This is needed because the start "
+ "and end predictions are not conditioned on one another."
+ )
+ },
+ )
+
+ def __post_init__(self):
+ if (
+ self.dataset_name is None
+ and self.train_file is None
+ and self.validation_file is None
+ and self.test_file is None
+ ):
+ raise ValueError("Need either a dataset name or a training/validation file/test_file.")
+ else:
+ if self.train_file is not None:
+ extension = self.train_file.split(".")[-1]
+ assert extension in ["csv", "json"], "`train_file` should be a csv or a json file."
+ if self.validation_file is not None:
+ extension = self.validation_file.split(".")[-1]
+ assert extension in ["csv", "json"], "`validation_file` should be a csv or a json file."
+ if self.test_file is not None:
+ extension = self.test_file.split(".")[-1]
+ assert extension in ["csv", "json"], "`test_file` should be a csv or a json file."
+
+
+def main():
+ # See all possible arguments in src/transformers/training_args.py
+ # or by passing the --help flag to this script.
+ # We now keep distinct sets of args, for a cleaner separation of concerns.
+
+ parser = HfArgumentParser((ModelArguments, DataTrainingArguments, TrainingArguments))
+ if len(sys.argv) == 2 and sys.argv[1].endswith(".json"):
+ # If we pass only one argument to the script and it's the path to a json file,
+ # let's parse it to get our arguments.
+ model_args, data_args, training_args = parser.parse_json_file(json_file=os.path.abspath(sys.argv[1]))
+ else:
+ model_args, data_args, training_args = parser.parse_args_into_dataclasses()
+
+ # Setup logging
+ logging.basicConfig(
+ format="%(asctime)s - %(levelname)s - %(name)s - %(message)s",
+ datefmt="%m/%d/%Y %H:%M:%S",
+ level=logging.INFO,
+ )
+
+ if training_args.should_log:
+ # The default of training_args.log_level is passive, so we set log level at info here to have that default.
+ transformers.utils.logging.set_verbosity_info()
+
+ log_level = training_args.get_process_log_level()
+ logger.setLevel(log_level)
+ datasets.utils.logging.set_verbosity(log_level)
+ transformers.utils.logging.set_verbosity(log_level)
+ transformers.utils.logging.enable_default_handler()
+ transformers.utils.logging.enable_explicit_format()
+
+ # Log on each process the small summary:
+ logger.warning(
+ f"Process rank: {training_args.local_rank}, device: {training_args.device}, n_gpu: {training_args.n_gpu} "
+ + f"distributed training: {bool(training_args.local_rank != -1)}, 16-bits training: {training_args.fp16}"
+ )
+ # logger.info(f"Training/evaluation parameters {training_args}")
+
+ # Detecting last checkpoint.
+ last_checkpoint = None
+ if os.path.isdir(training_args.output_dir) and training_args.do_train and not training_args.overwrite_output_dir:
+ last_checkpoint = get_last_checkpoint(training_args.output_dir)
+ if last_checkpoint is None and len(os.listdir(training_args.output_dir)) > 0:
+ raise ValueError(
+ f"Output directory ({training_args.output_dir}) already exists and is not empty. "
+ "Use --overwrite_output_dir to overcome."
+ )
+ elif last_checkpoint is not None and training_args.resume_from_checkpoint is None:
+ logger.info(
+ f"Checkpoint detected, resuming training at {last_checkpoint}. To avoid this behavior, change "
+ "the `--output_dir` or add `--overwrite_output_dir` to train from scratch."
+ )
+
+ # Set seed before initializing model.
+ set_seed(training_args.seed)
+
+ # Get the datasets: you can either provide your own CSV/JSON/TXT training and evaluation files (see below)
+ # or just provide the name of one of the public datasets available on the hub at https://huggingface.co/datasets/
+ # (the dataset will be downloaded automatically from the datasets Hub).
+ #
+ # For CSV/JSON files, this script will use the column called 'text' or the first column if no column called
+ # 'text' is found. You can easily tweak this behavior (see below).
+ #
+ # In distributed training, the load_dataset function guarantee that only one local process can concurrently
+ # download the dataset.
+ if data_args.dataset_name is not None:
+ # Downloading and loading a dataset from the hub.
+ raw_datasets = load_dataset(
+ data_args.dataset_name,
+ data_args.dataset_config_name,
+ cache_dir=model_args.cache_dir,
+ use_auth_token=True if model_args.use_auth_token else None,
+ )
+ else:
+ data_files = {}
+ if data_args.train_file is not None:
+ data_files["train"] = data_args.train_file
+ extension = data_args.train_file.split(".")[-1]
+
+ if data_args.validation_file is not None:
+ data_files["validation"] = data_args.validation_file
+ extension = data_args.validation_file.split(".")[-1]
+ if data_args.test_file is not None:
+ data_files["test"] = data_args.test_file
+ extension = data_args.test_file.split(".")[-1]
+ raw_datasets = load_dataset(
+ extension,
+ data_files=data_files,
+ field="data",
+ cache_dir=model_args.cache_dir,
+ use_auth_token=True if model_args.use_auth_token else None,
+ )
+ # See more about loading any type of standard or custom dataset (from files, python dict, pandas DataFrame, etc) at
+ # https://huggingface.co/docs/datasets/loading_datasets.html.
+
+ # Load pretrained model and tokenizer
+ #
+ # Distributed training:
+ # The .from_pretrained methods guarantee that only one local process can concurrently
+ # download model & vocab.
+ config = AutoConfig.from_pretrained(
+ model_args.config_name if model_args.config_name else model_args.model_name_or_path,
+ cache_dir=model_args.cache_dir,
+ revision=model_args.model_revision,
+ use_auth_token=True if model_args.use_auth_token else None,
+ )
+ tokenizer = AutoTokenizer.from_pretrained(
+ model_args.tokenizer_name if model_args.tokenizer_name else model_args.model_name_or_path,
+ cache_dir=model_args.cache_dir,
+ use_fast=True,
+ revision=model_args.model_revision,
+ use_auth_token=True if model_args.use_auth_token else None,
+ keep_accents=model_args.keep_accents,
+ do_lower_case=model_args.do_lower_case
+ )
+ model = AutoModelForQuestionAnswering.from_pretrained(
+ model_args.model_name_or_path,
+ from_tf=bool(".ckpt" in model_args.model_name_or_path),
+ config=config,
+ cache_dir=model_args.cache_dir,
+ revision=model_args.model_revision,
+ use_auth_token=True if model_args.use_auth_token else None,
+ )
+
+ # Tokenizer check: this script requires a fast tokenizer.
+ if not isinstance(tokenizer, PreTrainedTokenizerFast):
+ raise ValueError(
+ "This example script only works for models that have a fast tokenizer. Checkout the big table of models at"
+ " https://huggingface.co/transformers/index.html#supported-frameworks to find the model types that meet"
+ " this requirement"
+ )
+
+ # Preprocessing the datasets.
+ # Preprocessing is slighlty different for training and evaluation.
+ if training_args.do_train:
+ column_names = raw_datasets["train"].column_names
+ elif training_args.do_eval:
+ column_names = raw_datasets["validation"].column_names
+ else:
+ column_names = raw_datasets["test"].column_names
+ question_column_name = "question" if "question" in column_names else column_names[0]
+ context_column_name = "context" if "context" in column_names else column_names[1]
+ answer_column_name = "answers" if "answers" in column_names else column_names[2]
+
+ # Padding side determines if we do (question|context) or (context|question).
+ pad_on_right = tokenizer.padding_side == "right"
+
+ if data_args.max_seq_length > tokenizer.model_max_length:
+ logger.warning(
+ f"The max_seq_length passed ({data_args.max_seq_length}) is larger than the maximum length for the"
+ f"model ({tokenizer.model_max_length}). Using max_seq_length={tokenizer.model_max_length}."
+ )
+ max_seq_length = min(data_args.max_seq_length, tokenizer.model_max_length)
+
+ # Training preprocessing
+ def prepare_train_features(examples):
+ # Some of the questions have lots of whitespace on the left, which is not useful and will make the
+ # truncation of the context fail (the tokenized question will take a lots of space). So we remove that
+ # left whitespace
+ examples[question_column_name] = [q.lstrip() for q in examples[question_column_name]]
+
+ # Tokenize our examples with truncation and maybe padding, but keep the overflows using a stride. This results
+ # in one example possible giving several features when a context is long, each of those features having a
+ # context that overlaps a bit the context of the previous feature.
+ tokenized_examples = tokenizer(
+ examples[question_column_name if pad_on_right else context_column_name],
+ examples[context_column_name if pad_on_right else question_column_name],
+ truncation="only_second" if pad_on_right else "only_first",
+ max_length=max_seq_length,
+ stride=data_args.doc_stride,
+ return_overflowing_tokens=True,
+ return_offsets_mapping=True,
+ padding="max_length" if data_args.pad_to_max_length else False,
+ )
+
+ # Since one example might give us several features if it has a long context, we need a map from a feature to
+ # its corresponding example. This key gives us just that.
+ sample_mapping = tokenized_examples.pop("overflow_to_sample_mapping")
+ # The offset mappings will give us a map from token to character position in the original context. This will
+ # help us compute the start_positions and end_positions.
+ offset_mapping = tokenized_examples.pop("offset_mapping")
+
+ # Let's label those examples!
+ tokenized_examples["start_positions"] = []
+ tokenized_examples["end_positions"] = []
+
+ for i, offsets in enumerate(offset_mapping):
+ # We will label impossible answers with the index of the CLS token.
+ input_ids = tokenized_examples["input_ids"][i]
+ cls_index = input_ids.index(tokenizer.cls_token_id)
+
+ # Grab the sequence corresponding to that example (to know what is the context and what is the question).
+ sequence_ids = tokenized_examples.sequence_ids(i)
+
+ # One example can give several spans, this is the index of the example containing this span of text.
+ sample_index = sample_mapping[i]
+ answers = examples[answer_column_name][sample_index]
+ # If no answers are given, set the cls_index as answer.
+ if len(answers["answer_start"]) == 0:
+ tokenized_examples["start_positions"].append(cls_index)
+ tokenized_examples["end_positions"].append(cls_index)
+ else:
+ # Start/end character index of the answer in the text.
+ start_char = answers["answer_start"][0]
+ end_char = start_char + len(answers["text"][0])
+
+ # Start token index of the current span in the text.
+ token_start_index = 0
+ while sequence_ids[token_start_index] != (1 if pad_on_right else 0):
+ token_start_index += 1
+
+ # End token index of the current span in the text.
+ token_end_index = len(input_ids) - 1
+ while sequence_ids[token_end_index] != (1 if pad_on_right else 0):
+ token_end_index -= 1
+
+ # Detect if the answer is out of the span (in which case this feature is labeled with the CLS index).
+ if not (offsets[token_start_index][0] <= start_char and offsets[token_end_index][1] >= end_char):
+ tokenized_examples["start_positions"].append(cls_index)
+ tokenized_examples["end_positions"].append(cls_index)
+ else:
+ # Otherwise move the token_start_index and token_end_index to the two ends of the answer.
+ # Note: we could go after the last offset if the answer is the last word (edge case).
+ while token_start_index < len(offsets) and offsets[token_start_index][0] <= start_char:
+ token_start_index += 1
+ tokenized_examples["start_positions"].append(token_start_index - 1)
+ while offsets[token_end_index][1] >= end_char:
+ token_end_index -= 1
+ tokenized_examples["end_positions"].append(token_end_index + 1)
+
+ return tokenized_examples
+
+ if training_args.do_train:
+ if "train" not in raw_datasets:
+ raise ValueError("--do_train requires a train dataset")
+ train_dataset = raw_datasets["train"]
+ if data_args.max_train_samples is not None:
+ # We will select sample from whole data if argument is specified
+ max_train_samples = min(len(train_dataset), data_args.max_train_samples)
+ train_dataset = train_dataset.select(range(max_train_samples))
+ # Create train feature from dataset
+ with training_args.main_process_first(desc="train dataset map pre-processing"):
+ train_dataset = train_dataset.map(
+ prepare_train_features,
+ batched=True,
+ num_proc=data_args.preprocessing_num_workers,
+ remove_columns=column_names,
+ load_from_cache_file=not data_args.overwrite_cache,
+ desc="Running tokenizer on train dataset",
+ )
+ if data_args.max_train_samples is not None:
+ # Number of samples might increase during Feature Creation, We select only specified max samples
+ max_train_samples = min(len(train_dataset), data_args.max_train_samples)
+ train_dataset = train_dataset.select(range(max_train_samples))
+
+ # Validation preprocessing
+ def prepare_validation_features(examples):
+ # Some of the questions have lots of whitespace on the left, which is not useful and will make the
+ # truncation of the context fail (the tokenized question will take a lots of space). So we remove that
+ # left whitespace
+ examples[question_column_name] = [q.lstrip() for q in examples[question_column_name]]
+
+ # Tokenize our examples with truncation and maybe padding, but keep the overflows using a stride. This results
+ # in one example possible giving several features when a context is long, each of those features having a
+ # context that overlaps a bit the context of the previous feature.
+ tokenized_examples = tokenizer(
+ examples[question_column_name if pad_on_right else context_column_name],
+ examples[context_column_name if pad_on_right else question_column_name],
+ truncation="only_second" if pad_on_right else "only_first",
+ max_length=max_seq_length,
+ stride=data_args.doc_stride,
+ return_overflowing_tokens=True,
+ return_offsets_mapping=True,
+ padding="max_length" if data_args.pad_to_max_length else False,
+ )
+
+ # Since one example might give us several features if it has a long context, we need a map from a feature to
+ # its corresponding example. This key gives us just that.
+ sample_mapping = tokenized_examples.pop("overflow_to_sample_mapping")
+
+ # For evaluation, we will need to convert our predictions to substrings of the context, so we keep the
+ # corresponding example_id and we will store the offset mappings.
+ tokenized_examples["example_id"] = []
+
+ for i in range(len(tokenized_examples["input_ids"])):
+ # Grab the sequence corresponding to that example (to know what is the context and what is the question).
+ sequence_ids = tokenized_examples.sequence_ids(i)
+ context_index = 1 if pad_on_right else 0
+
+ # One example can give several spans, this is the index of the example containing this span of text.
+ sample_index = sample_mapping[i]
+ tokenized_examples["example_id"].append(examples["id"][sample_index])
+
+ # Set to None the offset_mapping that are not part of the context so it's easy to determine if a token
+ # position is part of the context or not.
+ tokenized_examples["offset_mapping"][i] = [
+ (o if sequence_ids[k] == context_index else None)
+ for k, o in enumerate(tokenized_examples["offset_mapping"][i])
+ ]
+
+ return tokenized_examples
+
+ if training_args.do_eval:
+ if "validation" not in raw_datasets:
+ raise ValueError("--do_eval requires a validation dataset")
+ eval_examples = raw_datasets["validation"]
+ if data_args.max_eval_samples is not None:
+ # We will select sample from whole data
+ max_eval_samples = min(len(eval_examples), data_args.max_eval_samples)
+ eval_examples = eval_examples.select(range(max_eval_samples))
+ # Validation Feature Creation
+ with training_args.main_process_first(desc="validation dataset map pre-processing"):
+ eval_dataset = eval_examples.map(
+ prepare_validation_features,
+ batched=True,
+ num_proc=data_args.preprocessing_num_workers,
+ remove_columns=column_names,
+ load_from_cache_file=not data_args.overwrite_cache,
+ desc="Running tokenizer on validation dataset",
+ )
+ if data_args.max_eval_samples is not None:
+ # During Feature creation dataset samples might increase, we will select required samples again
+ max_eval_samples = min(len(eval_dataset), data_args.max_eval_samples)
+ eval_dataset = eval_dataset.select(range(max_eval_samples))
+
+ if training_args.do_predict:
+ if "test" not in raw_datasets:
+ raise ValueError("--do_predict requires a test dataset")
+ predict_examples = raw_datasets["test"]
+ if data_args.max_predict_samples is not None:
+ # We will select sample from whole data
+ predict_examples = predict_examples.select(range(data_args.max_predict_samples))
+ # Predict Feature Creation
+ with training_args.main_process_first(desc="prediction dataset map pre-processing"):
+ predict_dataset = predict_examples.map(
+ prepare_validation_features,
+ batched=True,
+ num_proc=data_args.preprocessing_num_workers,
+ remove_columns=column_names,
+ load_from_cache_file=not data_args.overwrite_cache,
+ desc="Running tokenizer on prediction dataset",
+ )
+ if data_args.max_predict_samples is not None:
+ # During Feature creation dataset samples might increase, we will select required samples again
+ max_predict_samples = min(len(predict_dataset), data_args.max_predict_samples)
+ predict_dataset = predict_dataset.select(range(max_predict_samples))
+
+ # Data collator
+ # We have already padded to max length if the corresponding flag is True, otherwise we need to pad in the data
+ # collator.
+ data_collator = (
+ default_data_collator
+ if data_args.pad_to_max_length
+ else DataCollatorWithPadding(tokenizer, pad_to_multiple_of=8 if training_args.fp16 else None)
+ )
+
+ # Post-processing:
+ def post_processing_function(examples, features, predictions, stage="eval"):
+ # Post-processing: we match the start logits and end logits to answers in the original context.
+ predictions = postprocess_qa_predictions(
+ examples=examples,
+ features=features,
+ predictions=predictions,
+ version_2_with_negative=data_args.version_2_with_negative,
+ n_best_size=data_args.n_best_size,
+ max_answer_length=data_args.max_answer_length,
+ null_score_diff_threshold=data_args.null_score_diff_threshold,
+ output_dir=training_args.output_dir,
+ log_level=log_level,
+ prefix=stage,
+ )
+ # Format the result to the format the metric expects.
+ if data_args.version_2_with_negative:
+ formatted_predictions = [
+ {"id": str(k), "prediction_text": v, "no_answer_probability": 0.0} for k, v in predictions.items()
+ ]
+ else:
+ formatted_predictions = [{"id": str(k), "prediction_text": v} for k, v in predictions.items()]
+
+ references = [{"id": str(ex["id"]), "answers": ex[answer_column_name]} for ex in examples]
+ return EvalPrediction(predictions=formatted_predictions, label_ids=references)
+
+ metric = evaluate.load("squad_v2" if data_args.version_2_with_negative else "squad")
+
+ def compute_metrics(p: EvalPrediction):
+ return metric.compute(predictions=p.predictions, references=p.label_ids)
+
+ # Initialize our Trainer
+ trainer = QuestionAnsweringTrainer(
+ model=model,
+ args=training_args,
+ train_dataset=train_dataset if training_args.do_train else None,
+ eval_dataset=eval_dataset if training_args.do_eval else None,
+ eval_examples=eval_examples if training_args.do_eval else None,
+ tokenizer=tokenizer,
+ data_collator=data_collator,
+ post_process_function=post_processing_function,
+ compute_metrics=compute_metrics,
+ )
+
+ # Training
+ if training_args.do_train:
+ checkpoint = None
+ if training_args.resume_from_checkpoint is not None:
+ checkpoint = training_args.resume_from_checkpoint
+ elif last_checkpoint is not None:
+ checkpoint = last_checkpoint
+ start_time = timeit.default_timer()
+ train_result = trainer.train(resume_from_checkpoint=checkpoint)
+ end_time = timeit.default_timer()
+ total_train_time = round((end_time-start_time)/60, 3)
+
+ trainer.save_model() # Saves the tokenizer too for easy upload
+
+ metrics = train_result.metrics
+ max_train_samples = (
+ data_args.max_train_samples if data_args.max_train_samples is not None else len(train_dataset)
+ )
+ metrics["train_samples"] = min(max_train_samples, len(train_dataset))
+ metrics["total_train_time(min)"] = total_train_time
+
+ # final time save state
+ trainer.log_metrics("train", metrics)
+ trainer.save_metrics("train", metrics)
+ trainer.save_state()
+
+ # Evaluation
+ if training_args.do_eval:
+ logger.info("*** Evaluate ***")
+ metrics = trainer.evaluate()
+
+ max_eval_samples = data_args.max_eval_samples if data_args.max_eval_samples is not None else len(eval_dataset)
+ metrics["eval_samples"] = min(max_eval_samples, len(eval_dataset))
+
+ trainer.log_metrics("eval", metrics)
+ trainer.save_metrics("eval", metrics)
+
+ # Prediction
+ if training_args.do_predict:
+ logger.info("*** Predict ***")
+ results = trainer.predict(predict_dataset, predict_examples)
+ metrics = results.metrics
+
+ max_predict_samples = (
+ data_args.max_predict_samples if data_args.max_predict_samples is not None else len(predict_dataset)
+ )
+ metrics["predict_samples"] = min(max_predict_samples, len(predict_dataset))
+
+ trainer.log_metrics("predict", metrics)
+ trainer.save_metrics("predict", metrics)
+
+ kwargs = {"finetuned_from": model_args.model_name_or_path, "tasks": "question-answering"}
+ if data_args.dataset_name is not None:
+ kwargs["dataset_tags"] = data_args.dataset_name
+ if data_args.dataset_config_name is not None:
+ kwargs["dataset_args"] = data_args.dataset_config_name
+ kwargs["dataset"] = f"{data_args.dataset_name} {data_args.dataset_config_name}"
+ else:
+ kwargs["dataset"] = data_args.dataset_name
+
+ if training_args.push_to_hub:
+ trainer.push_to_hub(**kwargs)
+ else:
+ trainer.create_model_card(**kwargs)
+
+
+def _mp_fn(index):
+ # For xla_spawn (TPUs)
+ main()
+
+
+if __name__ == "__main__":
+ main()
\ No newline at end of file
diff --git a/nlp/question_answering/run_qa_inference.py b/nlp/question_answering/run_qa_inference.py
new file mode 100644
index 000000000..7ffb484dd
--- /dev/null
+++ b/nlp/question_answering/run_qa_inference.py
@@ -0,0 +1,216 @@
+#!/usr/bin/env python
+# coding=utf-8
+# Copyright 2023 C5ailabs Team (Authors: Rohit Sroch) All rights reserved.
+# Copyright 2020 The HuggingFace Team All rights reserved.
+#
+# Licensed under the Apache License, Version 2.0 (the "License");
+# you may not use this file except in compliance with the License.
+# You may obtain a copy of the License at
+#
+# http://www.apache.org/licenses/LICENSE-2.0
+#
+# Unless required by applicable law or agreed to in writing, software
+# distributed under the License is distributed on an "AS IS" BASIS,
+# WITHOUT WARRANTIES OR CONDITIONS OF ANY KIND, either express or implied.
+# See the License for the specific language governing permissions and
+# limitations under the License.
+"""
+Inference of a 🤗 Transformers model for question answering.
+"""
+
+import argparse
+import os
+
+from transformers import (
+ AutoConfig,
+ AutoModelForQuestionAnswering,
+ AutoTokenizer,
+ pipeline
+)
+
+# optimum-intel
+from optimum.intel import INCModelForQuestionAnswering
+
+from transformers.utils import check_min_version
+
+os.environ["TOKENIZERS_PARALLELISM"] = "false"
+# Will error if the minimal version of Transformers is not installed. Remove at your own risks.
+check_min_version("4.29.0")
+
+
+def parse_args():
+ parser = argparse.ArgumentParser(description="Inference transformers model on a Question Answering task")
+
+ ####################################
+ # Model Arguments
+ ####################################
+ parser.add_argument(
+ "--model_name_or_path",
+ type=str,
+ help="Path to pretrained model or model identifier from huggingface.co/models.",
+ required=True,
+ )
+ parser.add_argument(
+ "--max_seq_length",
+ type=int,
+ default=384,
+ help=(
+ "The maximum total input sequence length after tokenization. Sequences longer than this will be truncated,"
+ " sequences shorter will be padded if `--pad_to_max_lengh` is passed."
+ ),
+ )
+ parser.add_argument(
+ "--config_name",
+ type=str,
+ default=None,
+ help="Pretrained config name or path if not the same as model_name",
+ )
+ parser.add_argument(
+ "--tokenizer_name",
+ type=str,
+ default=None,
+ help="Pretrained tokenizer name or path if not the same as model_name",
+ )
+ parser.add_argument(
+ "--keep_accents",
+ action="store_true",
+ help="To preserve accents (vowel matras / diacritics) while tokenization",
+ )
+ parser.add_argument(
+ "--do_lower_case",
+ action="store_true",
+ help="Whether to lower case while tokenization",
+ )
+ parser.add_argument(
+ "--cache_dir",
+ type=str,
+ help="Where do you want to store the pretrained models downloaded from huggingface.co",
+ default='.cache'
+ )
+ parser.add_argument(
+ "--model_revision",
+ type=str,
+ help="The specific model version to use (can be a branch name, tag name or commit id).",
+ default='main'
+ )
+ parser.add_argument(
+ "--model_type",
+ type=str,
+ help="Type of the model, Whether its quantized or vanilla",
+ default='vanilla_fp32',
+ choices=["vanilla_fp32", "quantized_int8"]
+ )
+ parser.add_argument(
+ "--ipex_enabled",
+ action="store_true",
+ help="Whether to enable IPEX (Intel Extention for Pytorch)"
+ )
+ ####################################
+ # Other Arguments
+ ####################################
+ parser.add_argument(
+ "--doc_stride",
+ type=int,
+ default=128,
+ help="When splitting up a long document into chunks how much stride to take between chunks.",
+ )
+ parser.add_argument(
+ "--top_k",
+ type=int,
+ default=1,
+ help="The number of answers to return (will be chosen by order of likelihood).",
+ )
+ parser.add_argument(
+ "--max_answer_length",
+ type=int,
+ default=30,
+ help=(
+ "The maximum length of an answer that can be generated. This is needed because the start "
+ "and end predictions are not conditioned on one another."
+ ),
+ )
+
+ args = parser.parse_args()
+
+ return args
+
+
+def load_qa_pipeline(args):
+ """load the QA pipeline"""
+ qa_pipeline = None
+
+ tokenizer = AutoTokenizer.from_pretrained(
+ args.tokenizer_name if args.tokenizer_name else args.model_name_or_path,
+ cache_dir=args.cache_dir,
+ use_fast=True,
+ revision=args.model_revision,
+ keep_accents=args.keep_accents,
+ do_lower_case=args.do_lower_case
+ )
+ if args.model_type == "vanilla_fp32":
+ config = AutoConfig.from_pretrained(
+ args.config_name if args.config_name else args.model_name_or_path,
+ cache_dir=args.cache_dir,
+ revision=args.model_revision
+ )
+ model = AutoModelForQuestionAnswering.from_pretrained(
+ args.model_name_or_path,
+ from_tf=bool(".ckpt" in args.model_name_or_path),
+ config=config,
+ cache_dir=args.cache_dir,
+ revision=args.model_revision
+ )
+ if args.ipex_enabled:
+ try:
+ import intel_extension_for_pytorch as ipex
+ except:
+ assert False, "transformers 4.29.0 requests IPEX version higher or equal to 1.12"
+ model = ipex.optimize(model)
+
+ qa_pipeline = pipeline(
+ task="question-answering",
+ model=model,
+ tokenizer=tokenizer
+ )
+ elif args.model_type == "quantized_int8":
+
+ model = INCModelForQuestionAnswering.from_pretrained(
+ args.model_name_or_path,
+ cache_dir=args.cache_dir,
+ revision=args.model_revision
+ )
+ qa_pipeline = pipeline(
+ task="question-answering",
+ model=model,
+ tokenizer=tokenizer
+ )
+
+ return qa_pipeline
+
+
+
+def main():
+
+ args = parse_args()
+
+ qa_pipeline = load_qa_pipeline(args)
+
+ print("*"*100)
+ context = input("Type Context >>>")
+ question = input("Type Question >>>")
+ print("*"*100)
+ preds = qa_pipeline(
+ question=question,
+ context=context,
+ doc_stride=args.doc_stride,
+ max_answer_len=args.max_answer_length,
+ max_seq_len=args.max_seq_length,
+ top_k=args.top_k
+ )
+ print(
+ f"score: {round(preds['score'], 4)}, start: {preds['start']}, end: {preds['end']}, answer: {preds['answer']}"
+ )
+
+
+if __name__ == "__main__":
+ main()
\ No newline at end of file
diff --git a/nlp/question_answering/run_qa_pot.py b/nlp/question_answering/run_qa_pot.py
new file mode 100644
index 000000000..0ab191ae2
--- /dev/null
+++ b/nlp/question_answering/run_qa_pot.py
@@ -0,0 +1,603 @@
+#!/usr/bin/env python
+# coding=utf-8
+# Copyright 2023 C5ailabs Team (Authors: Rohit Sroch) All rights reserved.
+# Copyright 2020 The HuggingFace Team All rights reserved.
+#
+# Licensed under the Apache License, Version 2.0 (the "License");
+# you may not use this file except in compliance with the License.
+# You may obtain a copy of the License at
+#
+# http://www.apache.org/licenses/LICENSE-2.0
+#
+# Unless required by applicable law or agreed to in writing, software
+# distributed under the License is distributed on an "AS IS" BASIS,
+# WITHOUT WARRANTIES OR CONDITIONS OF ANY KIND, either express or implied.
+# See the License for the specific language governing permissions and
+# limitations under the License.
+"""
+Post Training Optimization/Quantization of a 🤗 Transformers model for question answering using 💻 IPEX (Intel® Extension for PyTorch) and 🤖 Neural Compressor.
+"""
+# You can also adapt this script on your own question answering task. Pointers for this are left as comments.
+
+import logging
+import os
+import json
+import timeit
+import sys
+import psutil
+from dataclasses import dataclass, field
+from typing import Optional
+
+import torch
+import datasets
+import evaluate
+from datasets import load_dataset
+from trainer_qa import QuestionAnsweringTrainer
+from utils_qa import postprocess_qa_predictions
+
+import transformers
+from transformers import (
+ AutoConfig,
+ AutoModelForQuestionAnswering,
+ AutoTokenizer,
+ DataCollatorWithPadding,
+ EvalPrediction,
+ HfArgumentParser,
+ PreTrainedTokenizerFast,
+ TrainingArguments,
+ default_data_collator
+)
+
+from transformers.utils import check_min_version
+from transformers.utils.versions import require_version
+
+# neural compressor
+from neural_compressor.config import PostTrainingQuantConfig
+from neural_compressor import quantization
+
+from neural_compressor import benchmark
+from neural_compressor.config import BenchmarkConfig
+from neural_compressor.utils.pytorch import load
+from neural_compressor.adaptor.pytorch import get_example_inputs
+
+try:
+ import intel_extension_for_pytorch as ipex
+except:
+ assert False, "transformers 4.29.0 requests IPEX version higher or equal to 1.12"
+
+os.environ["TOKENIZERS_PARALLELISM"] = "false"
+# Will error if the minimal version of Transformers is not installed. Remove at your own risks.
+check_min_version("4.29.0")
+
+require_version("datasets>=1.8.0", "To fix: pip install datasets")
+
+logger = logging.getLogger(__name__)
+
+
+@dataclass
+class ModelArguments:
+ """
+ Arguments pertaining to which model/config/tokenizer we are going to fine-tune from.
+ """
+
+ model_name_or_path: str = field(
+ metadata={"help": "Path to pretrained model or model identifier from huggingface.co/models"}
+ )
+ config_name: Optional[str] = field(
+ default=None, metadata={"help": "Pretrained config name or path if not the same as model_name"}
+ )
+ tokenizer_name: Optional[str] = field(
+ default=None, metadata={"help": "Pretrained tokenizer name or path if not the same as model_name"}
+ )
+ cache_dir: Optional[str] = field(
+ default=".cache",
+ metadata={"help": "Path to directory to store the pretrained models downloaded from huggingface.co"},
+ )
+ model_revision: str = field(
+ default="main",
+ metadata={"help": "The specific model version to use (can be a branch name, tag name or commit id)."},
+ )
+ use_auth_token: bool = field(
+ default=False,
+ metadata={
+ "help": (
+ "Will use the token generated when running `huggingface-cli login` (necessary to use this script "
+ "with private models)."
+ )
+ },
+ )
+ keep_accents: bool = field(
+ default=False,
+ metadata={
+ "help": "To preserve accents (vowel matras / diacritics) while tokenization "
+
+ },
+ )
+ do_lower_case: bool = field(
+ default=False,
+ metadata={
+ "help": "Whether to lower case while tokenization"
+
+ },
+ )
+
+ #########################################
+ # Neural Compressor Arguments and IPEX
+ #########################################
+ tune: bool = field(
+ default=False,
+ metadata={"help": "Whether or not to apply quantization."},
+ )
+ ptq_method: str = field(
+ default="dynamic_qat",
+ metadata={"help": "Post Training Quantization method with choices as dynamic_int8, static_int8, static_smooth_int8"},
+ )
+ int8: bool = field(
+ default=False, metadata={"help": "use int8 model to get accuracy or benchmark"}
+ )
+ backend: str = field(
+ default="default",
+ metadata={"help": "Post Training Quantization backend with choices as default, ipex"},
+ )
+ benchmark: bool = field(
+ default=False, metadata={"help": "get benchmark instead of accuracy"}
+ )
+ accuracy_only: bool = field(
+ default=False, metadata={"help": "get accuracy"}
+ )
+ iters: int = field(
+ default=100,
+ metadata={
+ "help": "The inference iterations to run for benchmark."
+ },
+ )
+
+
+@dataclass
+class DataTrainingArguments:
+ """
+ Arguments pertaining to what data we are going to input our model for training and eval.
+ """
+
+ dataset_name: Optional[str] = field(
+ default=None, metadata={"help": "The name of the dataset to use (via the datasets library)."}
+ )
+ dataset_config_name: Optional[str] = field(
+ default=None, metadata={"help": "The configuration name of the dataset to use (via the datasets library)."}
+ )
+ validation_file: Optional[str] = field(
+ default=None,
+ metadata={"help": "An optional input evaluation data file to evaluate the perplexity on (a text file)."},
+ )
+ overwrite_cache: bool = field(
+ default=False, metadata={"help": "Overwrite the cached training and evaluation sets"}
+ )
+ preprocessing_num_workers: Optional[int] = field(
+ default=None,
+ metadata={"help": "The number of processes to use for the preprocessing."},
+ )
+ max_seq_length: int = field(
+ default=384,
+ metadata={
+ "help": (
+ "The maximum total input sequence length after tokenization. Sequences longer "
+ "than this will be truncated, sequences shorter will be padded."
+ )
+ },
+ )
+ pad_to_max_length: bool = field(
+ default=True,
+ metadata={
+ "help": (
+ "Whether to pad all samples to `max_seq_length`. If False, will pad the samples dynamically when"
+ " batching to the maximum length in the batch (which can be faster on GPU but will be slower on TPU)."
+ )
+ },
+ )
+ max_eval_samples: Optional[int] = field(
+ default=50,
+ metadata={
+ "help": (
+ "For debugging purposes or quicker training, truncate the number of evaluation examples to this "
+ "value if set."
+ )
+ },
+ )
+ version_2_with_negative: bool = field(
+ default=False, metadata={"help": "If true, some of the examples do not have an answer."}
+ )
+ null_score_diff_threshold: float = field(
+ default=0.0,
+ metadata={
+ "help": (
+ "The threshold used to select the null answer: if the best answer has a score that is less than "
+ "the score of the null answer minus this threshold, the null answer is selected for this example. "
+ "Only useful when `version_2_with_negative=True`."
+ )
+ },
+ )
+ doc_stride: int = field(
+ default=128,
+ metadata={"help": "When splitting up a long document into chunks, how much stride to take between chunks."},
+ )
+ n_best_size: int = field(
+ default=20,
+ metadata={"help": "The total number of n-best predictions to generate when looking for an answer."},
+ )
+ max_answer_length: int = field(
+ default=30,
+ metadata={
+ "help": (
+ "The maximum length of an answer that can be generated. This is needed because the start "
+ "and end predictions are not conditioned on one another."
+ )
+ },
+ )
+
+ def __post_init__(self):
+ if (
+ self.dataset_name is None
+ and self.validation_file is None
+ ):
+ raise ValueError("Need either a dataset name or a training/validation file/test_file.")
+ else:
+ if self.validation_file is not None:
+ extension = self.validation_file.split(".")[-1]
+ assert extension in ["csv", "json"], "`validation_file` should be a csv or a json file."
+
+
+def main():
+ # See all possible arguments in src/transformers/training_args.py
+ # or by passing the --help flag to this script.
+ # We now keep distinct sets of args, for a cleaner separation of concerns.
+
+ parser = HfArgumentParser((ModelArguments, DataTrainingArguments, TrainingArguments))
+ if len(sys.argv) == 2 and sys.argv[1].endswith(".json"):
+ # If we pass only one argument to the script and it's the path to a json file,
+ # let's parse it to get our arguments.
+ model_args, data_args, training_args = parser.parse_json_file(json_file=os.path.abspath(sys.argv[1]))
+ else:
+ model_args, data_args, training_args = parser.parse_args_into_dataclasses()
+
+ # Setup logging
+ logging.basicConfig(
+ format="%(asctime)s - %(levelname)s - %(name)s - %(message)s",
+ datefmt="%m/%d/%Y %H:%M:%S",
+ level=logging.INFO,
+ )
+
+ if training_args.should_log:
+ # The default of training_args.log_level is passive, so we set log level at info here to have that default.
+ transformers.utils.logging.set_verbosity_info()
+
+ log_level = training_args.get_process_log_level()
+ logger.setLevel(log_level)
+ datasets.utils.logging.set_verbosity(log_level)
+ transformers.utils.logging.set_verbosity(log_level)
+ transformers.utils.logging.enable_default_handler()
+ transformers.utils.logging.enable_explicit_format()
+
+ if data_args.dataset_name is not None:
+ # Downloading and loading a dataset from the hub.
+ raw_datasets = load_dataset(
+ data_args.dataset_name,
+ data_args.dataset_config_name,
+ cache_dir=model_args.cache_dir,
+ use_auth_token=True if model_args.use_auth_token else None,
+ )
+ else:
+ data_files = {}
+ if data_args.validation_file is not None:
+ data_files["validation"] = data_args.validation_file
+ extension = data_args.validation_file.split(".")[-1]
+
+ raw_datasets = load_dataset(
+ extension,
+ data_files=data_files,
+ field="data",
+ cache_dir=model_args.cache_dir,
+ use_auth_token=True if model_args.use_auth_token else None,
+ )
+ # See more about loading any type of standard or custom dataset (from files, python dict, pandas DataFrame, etc) at
+ # https://huggingface.co/docs/datasets/loading_datasets.html.
+
+ # Load pretrained model and tokenizer
+ #
+ # Distributed training:
+ # The .from_pretrained methods guarantee that only one local process can concurrently
+ # download model & vocab.
+ config = AutoConfig.from_pretrained(
+ model_args.config_name if model_args.config_name else model_args.model_name_or_path,
+ cache_dir=model_args.cache_dir,
+ revision=model_args.model_revision,
+ use_auth_token=True if model_args.use_auth_token else None,
+ )
+ tokenizer = AutoTokenizer.from_pretrained(
+ model_args.tokenizer_name if model_args.tokenizer_name else model_args.model_name_or_path,
+ cache_dir=model_args.cache_dir,
+ use_fast=True,
+ revision=model_args.model_revision,
+ use_auth_token=True if model_args.use_auth_token else None,
+ keep_accents=model_args.keep_accents,
+ do_lower_case=model_args.do_lower_case
+ )
+ model = AutoModelForQuestionAnswering.from_pretrained(
+ model_args.model_name_or_path,
+ from_tf=bool(".ckpt" in model_args.model_name_or_path),
+ config=config,
+ cache_dir=model_args.cache_dir,
+ revision=model_args.model_revision,
+ use_auth_token=True if model_args.use_auth_token else None,
+ )
+
+ # Tokenizer check: this script requires a fast tokenizer.
+ if not isinstance(tokenizer, PreTrainedTokenizerFast):
+ raise ValueError(
+ "This example script only works for models that have a fast tokenizer. Checkout the big table of models at"
+ " https://huggingface.co/transformers/index.html#supported-frameworks to find the model types that meet"
+ " this requirement"
+ )
+
+ # Preprocessing the datasets.
+ # Preprocessing is slighlty different for training and evaluation.
+ column_names = raw_datasets["validation"].column_names
+
+ question_column_name = "question" if "question" in column_names else column_names[0]
+ context_column_name = "context" if "context" in column_names else column_names[1]
+ answer_column_name = "answers" if "answers" in column_names else column_names[2]
+
+ # Padding side determines if we do (question|context) or (context|question).
+ pad_on_right = tokenizer.padding_side == "right"
+
+ if data_args.max_seq_length > tokenizer.model_max_length:
+ logger.warning(
+ f"The max_seq_length passed ({data_args.max_seq_length}) is larger than the maximum length for the"
+ f"model ({tokenizer.model_max_length}). Using max_seq_length={tokenizer.model_max_length}."
+ )
+ max_seq_length = min(data_args.max_seq_length, tokenizer.model_max_length)
+
+ # Validation preprocessing
+ def prepare_validation_features(examples):
+ # Some of the questions have lots of whitespace on the left, which is not useful and will make the
+ # truncation of the context fail (the tokenized question will take a lots of space). So we remove that
+ # left whitespace
+ examples[question_column_name] = [q.lstrip() for q in examples[question_column_name]]
+
+ # Tokenize our examples with truncation and maybe padding, but keep the overflows using a stride. This results
+ # in one example possible giving several features when a context is long, each of those features having a
+ # context that overlaps a bit the context of the previous feature.
+ tokenized_examples = tokenizer(
+ examples[question_column_name if pad_on_right else context_column_name],
+ examples[context_column_name if pad_on_right else question_column_name],
+ truncation="only_second" if pad_on_right else "only_first",
+ max_length=max_seq_length,
+ stride=data_args.doc_stride,
+ return_overflowing_tokens=True,
+ return_offsets_mapping=True,
+ padding="max_length" if data_args.pad_to_max_length else False,
+ )
+
+ # Since one example might give us several features if it has a long context, we need a map from a feature to
+ # its corresponding example. This key gives us just that.
+ sample_mapping = tokenized_examples.pop("overflow_to_sample_mapping")
+
+ # For evaluation, we will need to convert our predictions to substrings of the context, so we keep the
+ # corresponding example_id and we will store the offset mappings.
+ tokenized_examples["example_id"] = []
+
+ for i in range(len(tokenized_examples["input_ids"])):
+ # Grab the sequence corresponding to that example (to know what is the context and what is the question).
+ sequence_ids = tokenized_examples.sequence_ids(i)
+ context_index = 1 if pad_on_right else 0
+
+ # One example can give several spans, this is the index of the example containing this span of text.
+ sample_index = sample_mapping[i]
+ tokenized_examples["example_id"].append(examples["id"][sample_index])
+
+ # Set to None the offset_mapping that are not part of the context so it's easy to determine if a token
+ # position is part of the context or not.
+ tokenized_examples["offset_mapping"][i] = [
+ (o if sequence_ids[k] == context_index else None)
+ for k, o in enumerate(tokenized_examples["offset_mapping"][i])
+ ]
+
+ return tokenized_examples
+
+ eval_examples = raw_datasets["validation"]
+ if data_args.max_eval_samples is not None:
+ # We will select sample from whole data
+ max_eval_samples = min(len(eval_examples), data_args.max_eval_samples)
+ eval_examples = eval_examples.select(range(max_eval_samples))
+ # Validation Feature Creation
+ with training_args.main_process_first(desc="validation dataset map pre-processing"):
+ eval_dataset = eval_examples.map(
+ prepare_validation_features,
+ batched=True,
+ num_proc=data_args.preprocessing_num_workers,
+ remove_columns=column_names,
+ load_from_cache_file=not data_args.overwrite_cache,
+ desc="Running tokenizer on validation dataset",
+ )
+ if data_args.max_eval_samples is not None:
+ # During Feature creation dataset samples might increase, we will select required samples again
+ max_eval_samples = min(len(eval_dataset), data_args.max_eval_samples)
+ eval_dataset = eval_dataset.select(range(max_eval_samples))
+
+
+ # Data collator
+ # We have already padded to max length if the corresponding flag is True, otherwise we need to pad in the data
+ # collator.
+ data_collator = (
+ default_data_collator
+ if data_args.pad_to_max_length
+ else DataCollatorWithPadding(tokenizer, pad_to_multiple_of=None)
+ )
+
+ # Post-processing:
+ def post_processing_function(examples, features, predictions, stage="eval"):
+ # Post-processing: we match the start logits and end logits to answers in the original context.
+ predictions = postprocess_qa_predictions(
+ examples=examples,
+ features=features,
+ predictions=predictions,
+ version_2_with_negative=data_args.version_2_with_negative,
+ n_best_size=data_args.n_best_size,
+ max_answer_length=data_args.max_answer_length,
+ null_score_diff_threshold=data_args.null_score_diff_threshold,
+ output_dir=training_args.output_dir,
+ log_level=log_level,
+ prefix=stage,
+ )
+ # Format the result to the format the metric expects.
+ if data_args.version_2_with_negative:
+ formatted_predictions = [
+ {"id": str(k), "prediction_text": v, "no_answer_probability": 0.0} for k, v in predictions.items()
+ ]
+ else:
+ formatted_predictions = [{"id": str(k), "prediction_text": v} for k, v in predictions.items()]
+
+ references = [{"id": str(ex["id"]), "answers": ex[answer_column_name]} for ex in examples]
+ return EvalPrediction(predictions=formatted_predictions, label_ids=references)
+
+ metric = evaluate.load("squad_v2" if data_args.version_2_with_negative else "squad")
+
+ def compute_metrics(p: EvalPrediction):
+ return metric.compute(predictions=p.predictions, references=p.label_ids)
+
+ # Initialize our Trainer
+ trainer = QuestionAnsweringTrainer(
+ model=model,
+ args=training_args,
+ train_dataset=None,
+ eval_dataset=eval_dataset,
+ eval_examples=eval_examples,
+ tokenizer=tokenizer,
+ data_collator=data_collator,
+ post_process_function=post_processing_function,
+ compute_metrics=compute_metrics,
+ )
+
+ ############################################################################################
+ logger.info("*"*100)
+ logger.info("\n\n")
+ eval_dataloader = trainer.get_eval_dataloader()
+ batch_size = eval_dataloader.batch_size
+ metric_name = "eval_f1"
+
+ def take_eval_steps(model, trainer, metric_name, save_metrics=False):
+ trainer.model = model
+ start_time = timeit.default_timer()
+ metrics = trainer.evaluate()
+ evalTime = timeit.default_timer() - start_time
+ max_eval_samples = data_args.max_eval_samples \
+ if data_args.max_eval_samples is not None else len(eval_dataset)
+ eval_samples = min(max_eval_samples, len(eval_dataset))
+ samples = eval_samples - (eval_samples % batch_size) \
+ if training_args.dataloader_drop_last else eval_samples
+ if save_metrics:
+ trainer.save_metrics("eval", metrics)
+
+ print('Batch size = %d' % batch_size)
+ print("Finally Eval {} Accuracy: {}".format(metric_name, metrics.get(metric_name)))
+ print("Latency: %.3f ms" % (evalTime / samples * 1000))
+ print("Throughput: {} samples/sec".format(samples / evalTime))
+ summary = {
+ "batch_size": batch_size,
+ "final_{}".format(metric_name): metrics.get(metric_name),
+ "latency (ms)": (evalTime / samples * 1000),
+ "throughput (samples/sec)": (samples / evalTime)
+ }
+ save_path = os.path.join(training_args.output_dir, "summary.json")
+ with open(save_path, "w") as fp:
+ json.dump(summary, fp)
+
+ return metrics.get(metric_name)
+
+ def eval_func(model):
+ return take_eval_steps(model, trainer, metric_name)
+
+ if model_args.tune and os.path.exists(os.path.join(training_args.output_dir, "pytorch_model.bin")) == False:
+ logger.info("************Perform INT8 Quantization using Intel® Neural Compressor using PTQ_METHOD={}, Backend={}************".format(
+ model_args.ptq_method,
+ model_args.backend
+ ))
+ if model_args.backend == "ipex":
+ ipex.nn.utils._model_convert.replace_dropout_with_identity(model)
+
+ recipes = {}
+ approach = "dynamic"
+ if model_args.ptq_method == "dynamic_int8":
+ approach = "dynamic"
+ elif model_args.ptq_method == "static_int8":
+ approach = "static"
+ elif model_args.ptq_method == "static_smooth_int8":
+ recipes={"smooth_quant": True, "smooth_quant_args": {"alpha": 0.5, "folding": True}}
+ approach = "static"
+
+ config = PostTrainingQuantConfig(
+ approach=approach,
+ backend=model_args.backend,
+ recipes=recipes,
+ calibration_sampling_size=data_args.max_eval_samples
+ )
+ q_model = quantization.fit(model,
+ config,
+ calib_dataloader=eval_dataloader,
+ eval_func=eval_func)
+ q_model.save(training_args.output_dir)
+ else:
+ if model_args.int8:
+ logger.info("************Already INT8 Quantizated model exists at {}. Delete it to Re-Tune!************".format(
+ training_args.output_dir
+ ))
+
+ if model_args.int8:
+ logger.info("************Loading INT8 Quantized Model using PTQ_METHOD={}, Backend={}************".format(
+ model_args.ptq_method,
+ model_args.backend
+ ))
+ model = load(training_args.output_dir, model, dataloader=eval_dataloader)
+ else:
+ model.eval()
+ if model_args.backend == "ipex":
+ logger.info("************Optimize FP32 Model using Backend={} i.e `ipex.optimize`************".format(
+ model_args.backend
+ ))
+ example_inputs = get_example_inputs(model, eval_dataloader)
+ model = ipex.optimize(model)
+ with torch.no_grad():
+ model = torch.jit.trace(model, example_inputs, strict=False)
+ model = torch.jit.freeze(model)
+
+ if model_args.benchmark or model_args.accuracy_only:
+ if model_args.int8:
+ logger.info("************Benchmark INT8 Pytorch Model using Backend={}************".format(
+ model_args.backend
+ ))
+ else:
+ logger.info("************Benchmark FP32 Pytorch Model using Backend={}************".format(
+ model_args.backend
+ ))
+ if model_args.benchmark:
+ try:
+ cpu_counts = psutil.cpu_count(logical=False)
+ b_conf = BenchmarkConfig(backend=model_args.backend,
+ warmup=5,
+ iteration=model_args.iters,
+ cores_per_instance=cpu_counts,
+ num_of_instance=1)
+ benchmark.fit(model, b_conf, b_dataloader=eval_dataloader)
+ except Exception:
+ pass
+ else:
+ eval_func(model)
+ ############################################################################################
+
+
+def _mp_fn(index):
+ # For xla_spawn (TPUs)
+ main()
+
+
+if __name__ == "__main__":
+ main()
\ No newline at end of file
diff --git a/nlp/question_answering/run_qa_pot_optimum.py b/nlp/question_answering/run_qa_pot_optimum.py
new file mode 100644
index 000000000..637bee307
--- /dev/null
+++ b/nlp/question_answering/run_qa_pot_optimum.py
@@ -0,0 +1,612 @@
+#!/usr/bin/env python
+# coding=utf-8
+# Copyright 2023 C5ailabs Team (Authors: Rohit Sroch) All rights reserved.
+# Copyright 2020 The HuggingFace Team All rights reserved.
+#
+# Licensed under the Apache License, Version 2.0 (the "License");
+# you may not use this file except in compliance with the License.
+# You may obtain a copy of the License at
+#
+# http://www.apache.org/licenses/LICENSE-2.0
+#
+# Unless required by applicable law or agreed to in writing, software
+# distributed under the License is distributed on an "AS IS" BASIS,
+# WITHOUT WARRANTIES OR CONDITIONS OF ANY KIND, either express or implied.
+# See the License for the specific language governing permissions and
+# limitations under the License.
+"""
+Post Training Optimization/Quantization of a 🤗 Transformers model for question answering using 💻 IPEX (Intel® Extension for PyTorch) and 🤖 Neural Compressor (Optimum-Intel).
+"""
+# You can also adapt this script on your own question answering task. Pointers for this are left as comments.
+
+import logging
+import os
+import json
+import timeit
+import sys
+import psutil
+from dataclasses import dataclass, field
+from typing import Optional
+
+import torch
+import datasets
+import evaluate
+from datasets import load_dataset
+from trainer_qa import QuestionAnsweringTrainer
+from utils_qa import postprocess_qa_predictions
+
+import transformers
+from transformers import (
+ AutoConfig,
+ AutoModelForQuestionAnswering,
+ AutoTokenizer,
+ DataCollatorWithPadding,
+ EvalPrediction,
+ HfArgumentParser,
+ PreTrainedTokenizerFast,
+ TrainingArguments,
+ default_data_collator
+)
+
+from transformers.utils import check_min_version
+from transformers.utils.versions import require_version
+
+# optimum-intel
+from optimum.intel import INCQuantizer
+from optimum.intel import INCModelForQuestionAnswering
+
+# neural compressor
+from neural_compressor.config import PostTrainingQuantConfig
+from neural_compressor import quantization
+
+from neural_compressor import benchmark
+from neural_compressor.config import BenchmarkConfig
+from neural_compressor.utils.pytorch import load
+from neural_compressor.adaptor.pytorch import get_example_inputs
+
+try:
+ import intel_extension_for_pytorch as ipex
+except:
+ assert False, "transformers 4.29.0 requests IPEX version higher or equal to 1.12"
+
+os.environ["TOKENIZERS_PARALLELISM"] = "false"
+# Will error if the minimal version of Transformers is not installed. Remove at your own risks.
+check_min_version("4.29.0")
+
+require_version("datasets>=1.8.0", "To fix: pip install datasets")
+
+logger = logging.getLogger(__name__)
+
+
+@dataclass
+class ModelArguments:
+ """
+ Arguments pertaining to which model/config/tokenizer we are going to fine-tune from.
+ """
+
+ model_name_or_path: str = field(
+ metadata={"help": "Path to pretrained model or model identifier from huggingface.co/models"}
+ )
+ config_name: Optional[str] = field(
+ default=None, metadata={"help": "Pretrained config name or path if not the same as model_name"}
+ )
+ tokenizer_name: Optional[str] = field(
+ default=None, metadata={"help": "Pretrained tokenizer name or path if not the same as model_name"}
+ )
+ cache_dir: Optional[str] = field(
+ default=".cache",
+ metadata={"help": "Path to directory to store the pretrained models downloaded from huggingface.co"},
+ )
+ model_revision: str = field(
+ default="main",
+ metadata={"help": "The specific model version to use (can be a branch name, tag name or commit id)."},
+ )
+ use_auth_token: bool = field(
+ default=False,
+ metadata={
+ "help": (
+ "Will use the token generated when running `huggingface-cli login` (necessary to use this script "
+ "with private models)."
+ )
+ },
+ )
+ keep_accents: bool = field(
+ default=False,
+ metadata={
+ "help": "To preserve accents (vowel matras / diacritics) while tokenization "
+
+ },
+ )
+ do_lower_case: bool = field(
+ default=False,
+ metadata={
+ "help": "Whether to lower case while tokenization"
+
+ },
+ )
+
+ #########################################
+ # Neural Compressor Arguments and IPEX
+ #########################################
+ tune: bool = field(
+ default=False,
+ metadata={"help": "Whether or not to apply quantization."},
+ )
+ ptq_method: str = field(
+ default="dynamic_qat",
+ metadata={"help": "Post Training Quantization method with choices as dynamic_int8, static_int8, static_smooth_int8"},
+ )
+ int8: bool = field(
+ default=False, metadata={"help": "use int8 model to get accuracy or benchmark"}
+ )
+ backend: str = field(
+ default="default",
+ metadata={"help": "Post Training Quantization backend with choices as default, ipex"},
+ )
+ benchmark: bool = field(
+ default=False, metadata={"help": "get benchmark instead of accuracy"}
+ )
+ accuracy_only: bool = field(
+ default=False, metadata={"help": "get accuracy"}
+ )
+ iters: int = field(
+ default=100,
+ metadata={
+ "help": "The inference iterations to run for benchmark."
+ },
+ )
+
+
+@dataclass
+class DataTrainingArguments:
+ """
+ Arguments pertaining to what data we are going to input our model for training and eval.
+ """
+
+ dataset_name: Optional[str] = field(
+ default=None, metadata={"help": "The name of the dataset to use (via the datasets library)."}
+ )
+ dataset_config_name: Optional[str] = field(
+ default=None, metadata={"help": "The configuration name of the dataset to use (via the datasets library)."}
+ )
+ validation_file: Optional[str] = field(
+ default=None,
+ metadata={"help": "An optional input evaluation data file to evaluate the perplexity on (a text file)."},
+ )
+ overwrite_cache: bool = field(
+ default=False, metadata={"help": "Overwrite the cached training and evaluation sets"}
+ )
+ preprocessing_num_workers: Optional[int] = field(
+ default=None,
+ metadata={"help": "The number of processes to use for the preprocessing."},
+ )
+ max_seq_length: int = field(
+ default=384,
+ metadata={
+ "help": (
+ "The maximum total input sequence length after tokenization. Sequences longer "
+ "than this will be truncated, sequences shorter will be padded."
+ )
+ },
+ )
+ pad_to_max_length: bool = field(
+ default=True,
+ metadata={
+ "help": (
+ "Whether to pad all samples to `max_seq_length`. If False, will pad the samples dynamically when"
+ " batching to the maximum length in the batch (which can be faster on GPU but will be slower on TPU)."
+ )
+ },
+ )
+ max_eval_samples: Optional[int] = field(
+ default=50,
+ metadata={
+ "help": (
+ "For debugging purposes or quicker training, truncate the number of evaluation examples to this "
+ "value if set."
+ )
+ },
+ )
+ version_2_with_negative: bool = field(
+ default=False, metadata={"help": "If true, some of the examples do not have an answer."}
+ )
+ null_score_diff_threshold: float = field(
+ default=0.0,
+ metadata={
+ "help": (
+ "The threshold used to select the null answer: if the best answer has a score that is less than "
+ "the score of the null answer minus this threshold, the null answer is selected for this example. "
+ "Only useful when `version_2_with_negative=True`."
+ )
+ },
+ )
+ doc_stride: int = field(
+ default=128,
+ metadata={"help": "When splitting up a long document into chunks, how much stride to take between chunks."},
+ )
+ n_best_size: int = field(
+ default=20,
+ metadata={"help": "The total number of n-best predictions to generate when looking for an answer."},
+ )
+ max_answer_length: int = field(
+ default=30,
+ metadata={
+ "help": (
+ "The maximum length of an answer that can be generated. This is needed because the start "
+ "and end predictions are not conditioned on one another."
+ )
+ },
+ )
+
+ def __post_init__(self):
+ if (
+ self.dataset_name is None
+ and self.validation_file is None
+ ):
+ raise ValueError("Need either a dataset name or a training/validation file/test_file.")
+ else:
+ if self.validation_file is not None:
+ extension = self.validation_file.split(".")[-1]
+ assert extension in ["csv", "json"], "`validation_file` should be a csv or a json file."
+
+
+def main():
+ # See all possible arguments in src/transformers/training_args.py
+ # or by passing the --help flag to this script.
+ # We now keep distinct sets of args, for a cleaner separation of concerns.
+
+ parser = HfArgumentParser((ModelArguments, DataTrainingArguments, TrainingArguments))
+ if len(sys.argv) == 2 and sys.argv[1].endswith(".json"):
+ # If we pass only one argument to the script and it's the path to a json file,
+ # let's parse it to get our arguments.
+ model_args, data_args, training_args = parser.parse_json_file(json_file=os.path.abspath(sys.argv[1]))
+ else:
+ model_args, data_args, training_args = parser.parse_args_into_dataclasses()
+
+ # Setup logging
+ logging.basicConfig(
+ format="%(asctime)s - %(levelname)s - %(name)s - %(message)s",
+ datefmt="%m/%d/%Y %H:%M:%S",
+ level=logging.INFO,
+ )
+
+ if training_args.should_log:
+ # The default of training_args.log_level is passive, so we set log level at info here to have that default.
+ transformers.utils.logging.set_verbosity_info()
+
+ log_level = training_args.get_process_log_level()
+ logger.setLevel(log_level)
+ datasets.utils.logging.set_verbosity(log_level)
+ transformers.utils.logging.set_verbosity(log_level)
+ transformers.utils.logging.enable_default_handler()
+ transformers.utils.logging.enable_explicit_format()
+
+ if data_args.dataset_name is not None:
+ # Downloading and loading a dataset from the hub.
+ raw_datasets = load_dataset(
+ data_args.dataset_name,
+ data_args.dataset_config_name,
+ cache_dir=model_args.cache_dir,
+ use_auth_token=True if model_args.use_auth_token else None,
+ )
+ else:
+ data_files = {}
+ if data_args.validation_file is not None:
+ data_files["validation"] = data_args.validation_file
+ extension = data_args.validation_file.split(".")[-1]
+
+ raw_datasets = load_dataset(
+ extension,
+ data_files=data_files,
+ field="data",
+ cache_dir=model_args.cache_dir,
+ use_auth_token=True if model_args.use_auth_token else None,
+ )
+ # See more about loading any type of standard or custom dataset (from files, python dict, pandas DataFrame, etc) at
+ # https://huggingface.co/docs/datasets/loading_datasets.html.
+
+ # Load pretrained model and tokenizer
+ #
+ # Distributed training:
+ # The .from_pretrained methods guarantee that only one local process can concurrently
+ # download model & vocab.
+ config = AutoConfig.from_pretrained(
+ model_args.config_name if model_args.config_name else model_args.model_name_or_path,
+ cache_dir=model_args.cache_dir,
+ revision=model_args.model_revision,
+ use_auth_token=True if model_args.use_auth_token else None,
+ )
+ tokenizer = AutoTokenizer.from_pretrained(
+ model_args.tokenizer_name if model_args.tokenizer_name else model_args.model_name_or_path,
+ cache_dir=model_args.cache_dir,
+ use_fast=True,
+ revision=model_args.model_revision,
+ use_auth_token=True if model_args.use_auth_token else None,
+ keep_accents=model_args.keep_accents,
+ do_lower_case=model_args.do_lower_case
+ )
+ model = AutoModelForQuestionAnswering.from_pretrained(
+ model_args.model_name_or_path,
+ from_tf=bool(".ckpt" in model_args.model_name_or_path),
+ config=config,
+ cache_dir=model_args.cache_dir,
+ revision=model_args.model_revision,
+ use_auth_token=True if model_args.use_auth_token else None,
+ )
+
+ # Tokenizer check: this script requires a fast tokenizer.
+ if not isinstance(tokenizer, PreTrainedTokenizerFast):
+ raise ValueError(
+ "This example script only works for models that have a fast tokenizer. Checkout the big table of models at"
+ " https://huggingface.co/transformers/index.html#supported-frameworks to find the model types that meet"
+ " this requirement"
+ )
+
+ # Preprocessing the datasets.
+ # Preprocessing is slighlty different for training and evaluation.
+ column_names = raw_datasets["validation"].column_names
+
+ question_column_name = "question" if "question" in column_names else column_names[0]
+ context_column_name = "context" if "context" in column_names else column_names[1]
+ answer_column_name = "answers" if "answers" in column_names else column_names[2]
+
+ # Padding side determines if we do (question|context) or (context|question).
+ pad_on_right = tokenizer.padding_side == "right"
+
+ if data_args.max_seq_length > tokenizer.model_max_length:
+ logger.warning(
+ f"The max_seq_length passed ({data_args.max_seq_length}) is larger than the maximum length for the"
+ f"model ({tokenizer.model_max_length}). Using max_seq_length={tokenizer.model_max_length}."
+ )
+ max_seq_length = min(data_args.max_seq_length, tokenizer.model_max_length)
+
+ # Validation preprocessing
+ def prepare_validation_features(examples):
+ # Some of the questions have lots of whitespace on the left, which is not useful and will make the
+ # truncation of the context fail (the tokenized question will take a lots of space). So we remove that
+ # left whitespace
+ examples[question_column_name] = [q.lstrip() for q in examples[question_column_name]]
+
+ # Tokenize our examples with truncation and maybe padding, but keep the overflows using a stride. This results
+ # in one example possible giving several features when a context is long, each of those features having a
+ # context that overlaps a bit the context of the previous feature.
+ tokenized_examples = tokenizer(
+ examples[question_column_name if pad_on_right else context_column_name],
+ examples[context_column_name if pad_on_right else question_column_name],
+ truncation="only_second" if pad_on_right else "only_first",
+ max_length=max_seq_length,
+ stride=data_args.doc_stride,
+ return_overflowing_tokens=True,
+ return_offsets_mapping=True,
+ padding="max_length" if data_args.pad_to_max_length else False,
+ )
+
+ # Since one example might give us several features if it has a long context, we need a map from a feature to
+ # its corresponding example. This key gives us just that.
+ sample_mapping = tokenized_examples.pop("overflow_to_sample_mapping")
+
+ # For evaluation, we will need to convert our predictions to substrings of the context, so we keep the
+ # corresponding example_id and we will store the offset mappings.
+ tokenized_examples["example_id"] = []
+
+ for i in range(len(tokenized_examples["input_ids"])):
+ # Grab the sequence corresponding to that example (to know what is the context and what is the question).
+ sequence_ids = tokenized_examples.sequence_ids(i)
+ context_index = 1 if pad_on_right else 0
+
+ # One example can give several spans, this is the index of the example containing this span of text.
+ sample_index = sample_mapping[i]
+ tokenized_examples["example_id"].append(examples["id"][sample_index])
+
+ # Set to None the offset_mapping that are not part of the context so it's easy to determine if a token
+ # position is part of the context or not.
+ tokenized_examples["offset_mapping"][i] = [
+ (o if sequence_ids[k] == context_index else None)
+ for k, o in enumerate(tokenized_examples["offset_mapping"][i])
+ ]
+
+ return tokenized_examples
+
+ eval_examples = raw_datasets["validation"]
+ if data_args.max_eval_samples is not None:
+ # We will select sample from whole data
+ max_eval_samples = min(len(eval_examples), data_args.max_eval_samples)
+ eval_examples = eval_examples.select(range(max_eval_samples))
+ # Validation Feature Creation
+ with training_args.main_process_first(desc="validation dataset map pre-processing"):
+ eval_dataset = eval_examples.map(
+ prepare_validation_features,
+ batched=True,
+ num_proc=data_args.preprocessing_num_workers,
+ remove_columns=column_names,
+ load_from_cache_file=not data_args.overwrite_cache,
+ desc="Running tokenizer on validation dataset",
+ )
+ if data_args.max_eval_samples is not None:
+ # During Feature creation dataset samples might increase, we will select required samples again
+ max_eval_samples = min(len(eval_dataset), data_args.max_eval_samples)
+ eval_dataset = eval_dataset.select(range(max_eval_samples))
+
+
+ # Data collator
+ # We have already padded to max length if the corresponding flag is True, otherwise we need to pad in the data
+ # collator.
+ data_collator = (
+ default_data_collator
+ if data_args.pad_to_max_length
+ else DataCollatorWithPadding(tokenizer, pad_to_multiple_of=None)
+ )
+
+ # Post-processing:
+ def post_processing_function(examples, features, predictions, stage="eval"):
+ # Post-processing: we match the start logits and end logits to answers in the original context.
+ predictions = postprocess_qa_predictions(
+ examples=examples,
+ features=features,
+ predictions=predictions,
+ version_2_with_negative=data_args.version_2_with_negative,
+ n_best_size=data_args.n_best_size,
+ max_answer_length=data_args.max_answer_length,
+ null_score_diff_threshold=data_args.null_score_diff_threshold,
+ output_dir=training_args.output_dir,
+ log_level=log_level,
+ prefix=stage,
+ )
+ # Format the result to the format the metric expects.
+ if data_args.version_2_with_negative:
+ formatted_predictions = [
+ {"id": str(k), "prediction_text": v, "no_answer_probability": 0.0} for k, v in predictions.items()
+ ]
+ else:
+ formatted_predictions = [{"id": str(k), "prediction_text": v} for k, v in predictions.items()]
+
+ references = [{"id": str(ex["id"]), "answers": ex[answer_column_name]} for ex in examples]
+ return EvalPrediction(predictions=formatted_predictions, label_ids=references)
+
+ metric = evaluate.load("squad_v2" if data_args.version_2_with_negative else "squad")
+
+ def compute_metrics(p: EvalPrediction):
+ return metric.compute(predictions=p.predictions, references=p.label_ids)
+
+ # Initialize our Trainer
+ trainer = QuestionAnsweringTrainer(
+ model=model,
+ args=training_args,
+ train_dataset=None,
+ eval_dataset=eval_dataset,
+ eval_examples=eval_examples,
+ tokenizer=tokenizer,
+ data_collator=data_collator,
+ post_process_function=post_processing_function,
+ compute_metrics=compute_metrics,
+ )
+
+ ############################################################################################
+ logger.info("*"*100)
+ logger.info("\n\n")
+ eval_dataloader = trainer.get_eval_dataloader()
+ batch_size = eval_dataloader.batch_size
+ metric_name = "eval_f1"
+
+ def take_eval_steps(model, trainer, metric_name, save_metrics=False):
+ trainer.model = model
+ start_time = timeit.default_timer()
+ metrics = trainer.evaluate()
+ evalTime = timeit.default_timer() - start_time
+ max_eval_samples = data_args.max_eval_samples \
+ if data_args.max_eval_samples is not None else len(eval_dataset)
+ eval_samples = min(max_eval_samples, len(eval_dataset))
+ samples = eval_samples - (eval_samples % batch_size) \
+ if training_args.dataloader_drop_last else eval_samples
+ if save_metrics:
+ trainer.save_metrics("eval", metrics)
+
+ print('Batch size = %d' % batch_size)
+ print("Finally Eval {} Accuracy: {}".format(metric_name, metrics.get(metric_name)))
+ print("Latency: %.3f ms" % (evalTime / samples * 1000))
+ print("Throughput: {} samples/sec".format(samples / evalTime))
+ summary = {
+ "batch_size": batch_size,
+ "final_{}".format(metric_name): metrics.get(metric_name),
+ "latency (ms)": (evalTime / samples * 1000),
+ "throughput (samples/sec)": (samples / evalTime)
+ }
+ save_path = os.path.join(training_args.output_dir, "summary.json")
+ with open(save_path, "w") as fp:
+ json.dump(summary, fp)
+
+ return metrics.get(metric_name)
+
+ def eval_func(model):
+ return take_eval_steps(model, trainer, metric_name)
+
+ if model_args.tune and os.path.exists(os.path.join(training_args.output_dir, "pytorch_model.bin")) == False:
+ logger.info("************Perform INT8 Quantization using Intel® Neural Compressor using PTQ_METHOD={}, Backend={}************".format(
+ model_args.ptq_method,
+ model_args.backend
+ ))
+ if model_args.backend == "ipex":
+ ipex.nn.utils._model_convert.replace_dropout_with_identity(model)
+
+ recipes = {}
+ approach = "dynamic"
+ if model_args.ptq_method == "dynamic_int8":
+ approach = "dynamic"
+ elif model_args.ptq_method == "static_int8":
+ approach = "static"
+ elif model_args.ptq_method == "static_smooth_int8":
+ recipes={"smooth_quant": True, "smooth_quant_args": {"alpha": 0.5, "folding": True}}
+ approach = "static"
+
+ config = PostTrainingQuantConfig(
+ approach=approach,
+ backend=model_args.backend,
+ recipes=recipes,
+ calibration_sampling_size=data_args.max_eval_samples
+ )
+
+ quantizer = INCQuantizer.from_pretrained(model, eval_fn=eval_func)
+ # Apply quantization and save the resulting model
+ quantizer.quantize(
+ quantization_config=config,
+ calibration_dataset=eval_dataset,
+ batch_size=batch_size,
+ save_directory=training_args.output_dir)
+ else:
+ if model_args.int8:
+ logger.info("************Already INT8 Quantizated model exists at {}. Delete it to Re-Tune!************".format(
+ training_args.output_dir
+ ))
+
+ if model_args.int8:
+ logger.info("************Loading INT8 Quantized Model using PTQ_METHOD={}, Backend={}************".format(
+ model_args.ptq_method,
+ model_args.backend
+ ))
+ model = INCModelForQuestionAnswering.from_pretrained(training_args.output_dir)
+ else:
+ model.eval()
+ if model_args.backend == "ipex":
+ logger.info("************Optimize FP32 Model using Backend={} i.e `ipex.optimize`************".format(
+ model_args.backend
+ ))
+ example_inputs = get_example_inputs(model, eval_dataloader)
+ model = ipex.optimize(model)
+ with torch.no_grad():
+ model = torch.jit.trace(model, example_inputs, strict=False)
+ model = torch.jit.freeze(model)
+
+
+ if model_args.benchmark or model_args.accuracy_only:
+ if model_args.int8:
+ logger.info("************Benchmark INT8 Pytorch Model using Backend={}************".format(
+ model_args.backend
+ ))
+ else:
+ logger.info("************Benchmark FP32 Pytorch Model using Backend={}************".format(
+ model_args.backend
+ ))
+
+ if model_args.benchmark:
+ try:
+ cpu_counts = psutil.cpu_count(logical=False)
+ b_conf = BenchmarkConfig(backend=model_args.backend,
+ warmup=5,
+ iteration=model_args.iters,
+ cores_per_instance=cpu_counts,
+ num_of_instance=1)
+ benchmark.fit(model, b_conf, b_dataloader=eval_dataloader)
+ except Exception:
+ pass
+ else:
+ eval_func(model)
+ ############################################################################################
+
+
+def _mp_fn(index):
+ # For xla_spawn (TPUs)
+ main()
+
+
+if __name__ == "__main__":
+ main()
\ No newline at end of file
diff --git a/nlp/question_answering/trainer_qa.py b/nlp/question_answering/trainer_qa.py
new file mode 100644
index 000000000..5535a3fda
--- /dev/null
+++ b/nlp/question_answering/trainer_qa.py
@@ -0,0 +1,136 @@
+# coding=utf-8
+# Copyright 2020 The HuggingFace Team All rights reserved.
+#
+# Licensed under the Apache License, Version 2.0 (the "License");
+# you may not use this file except in compliance with the License.
+# You may obtain a copy of the License at
+#
+# http://www.apache.org/licenses/LICENSE-2.0
+#
+# Unless required by applicable law or agreed to in writing, software
+# distributed under the License is distributed on an "AS IS" BASIS,
+# WITHOUT WARRANTIES OR CONDITIONS OF ANY KIND, either express or implied.
+# See the License for the specific language governing permissions and
+# limitations under the License.
+"""
+A subclass of `Trainer` specific to Question-Answering tasks
+"""
+import math
+import time
+
+from transformers import Trainer, is_torch_tpu_available
+from transformers.trainer_utils import PredictionOutput, speed_metrics
+
+
+if is_torch_tpu_available(check_device=False):
+ import torch_xla.core.xla_model as xm
+ import torch_xla.debug.metrics as met
+
+
+class QuestionAnsweringTrainer(Trainer):
+ def __init__(self, *args, eval_examples=None, post_process_function=None, **kwargs):
+ super().__init__(*args, **kwargs)
+ self.eval_examples = eval_examples
+ self.post_process_function = post_process_function
+
+ def evaluate(self, eval_dataset=None, eval_examples=None, ignore_keys=None, metric_key_prefix: str = "eval"):
+ eval_dataset = self.eval_dataset if eval_dataset is None else eval_dataset
+ eval_dataloader = self.get_eval_dataloader(eval_dataset)
+ eval_examples = self.eval_examples if eval_examples is None else eval_examples
+
+ # Temporarily disable metric computation, we will do it in the loop here.
+ compute_metrics = self.compute_metrics
+ self.compute_metrics = None
+ eval_loop = self.prediction_loop if self.args.use_legacy_prediction_loop else self.evaluation_loop
+ start_time = time.time()
+ try:
+ output = eval_loop(
+ eval_dataloader,
+ description="Evaluation",
+ # No point gathering the predictions if there are no metrics, otherwise we defer to
+ # self.args.prediction_loss_only
+ prediction_loss_only=True if compute_metrics is None else None,
+ ignore_keys=ignore_keys,
+ metric_key_prefix=metric_key_prefix,
+ )
+ finally:
+ self.compute_metrics = compute_metrics
+ total_batch_size = self.args.eval_batch_size * self.args.world_size
+ if f"{metric_key_prefix}_jit_compilation_time" in output.metrics:
+ start_time += output.metrics[f"{metric_key_prefix}_jit_compilation_time"]
+ output.metrics.update(
+ speed_metrics(
+ metric_key_prefix,
+ start_time,
+ num_samples=output.num_samples,
+ num_steps=math.ceil(output.num_samples / total_batch_size),
+ )
+ )
+ if self.post_process_function is not None and self.compute_metrics is not None and self.args.should_save:
+ # Only the main node write the results by default
+ eval_preds = self.post_process_function(eval_examples, eval_dataset, output.predictions)
+ metrics = self.compute_metrics(eval_preds)
+
+ # Prefix all keys with metric_key_prefix + '_'
+ for key in list(metrics.keys()):
+ if not key.startswith(f"{metric_key_prefix}_"):
+ metrics[f"{metric_key_prefix}_{key}"] = metrics.pop(key)
+ metrics.update(output.metrics)
+ else:
+ metrics = output.metrics
+
+ if self.args.should_log:
+ # Only the main node log the results by default
+ self.log(metrics)
+
+ if self.args.tpu_metrics_debug or self.args.debug:
+ # tpu-comment: Logging debug metrics for PyTorch/XLA (compile, execute times, ops, etc.)
+ xm.master_print(met.metrics_report())
+
+ self.control = self.callback_handler.on_evaluate(self.args, self.state, self.control, metrics)
+ return metrics
+
+ def predict(self, predict_dataset, predict_examples, ignore_keys=None, metric_key_prefix: str = "test"):
+ predict_dataloader = self.get_test_dataloader(predict_dataset)
+
+ # Temporarily disable metric computation, we will do it in the loop here.
+ compute_metrics = self.compute_metrics
+ self.compute_metrics = None
+ eval_loop = self.prediction_loop if self.args.use_legacy_prediction_loop else self.evaluation_loop
+ start_time = time.time()
+ try:
+ output = eval_loop(
+ predict_dataloader,
+ description="Prediction",
+ # No point gathering the predictions if there are no metrics, otherwise we defer to
+ # self.args.prediction_loss_only
+ prediction_loss_only=True if compute_metrics is None else None,
+ ignore_keys=ignore_keys,
+ metric_key_prefix=metric_key_prefix,
+ )
+ finally:
+ self.compute_metrics = compute_metrics
+ total_batch_size = self.args.eval_batch_size * self.args.world_size
+ if f"{metric_key_prefix}_jit_compilation_time" in output.metrics:
+ start_time += output.metrics[f"{metric_key_prefix}_jit_compilation_time"]
+ output.metrics.update(
+ speed_metrics(
+ metric_key_prefix,
+ start_time,
+ num_samples=output.num_samples,
+ num_steps=math.ceil(output.num_samples / total_batch_size),
+ )
+ )
+
+ if self.post_process_function is None or self.compute_metrics is None:
+ return output
+
+ predictions = self.post_process_function(predict_examples, predict_dataset, output.predictions, "predict")
+ metrics = self.compute_metrics(predictions)
+
+ # Prefix all keys with metric_key_prefix + '_'
+ for key in list(metrics.keys()):
+ if not key.startswith(f"{metric_key_prefix}_"):
+ metrics[f"{metric_key_prefix}_{key}"] = metrics.pop(key)
+ metrics.update(output.metrics)
+ return PredictionOutput(predictions=predictions.predictions, label_ids=predictions.label_ids, metrics=metrics)
\ No newline at end of file
diff --git a/nlp/question_answering/utils_qa.py b/nlp/question_answering/utils_qa.py
new file mode 100644
index 000000000..345e0dbda
--- /dev/null
+++ b/nlp/question_answering/utils_qa.py
@@ -0,0 +1,443 @@
+# coding=utf-8
+# Copyright 2020 The HuggingFace Team All rights reserved.
+#
+# Licensed under the Apache License, Version 2.0 (the "License");
+# you may not use this file except in compliance with the License.
+# You may obtain a copy of the License at
+#
+# http://www.apache.org/licenses/LICENSE-2.0
+#
+# Unless required by applicable law or agreed to in writing, software
+# distributed under the License is distributed on an "AS IS" BASIS,
+# WITHOUT WARRANTIES OR CONDITIONS OF ANY KIND, either express or implied.
+# See the License for the specific language governing permissions and
+# limitations under the License.
+"""
+Post-processing utilities for question answering.
+"""
+import collections
+import json
+import logging
+import os
+from typing import Optional, Tuple
+
+import numpy as np
+from tqdm.auto import tqdm
+
+
+logger = logging.getLogger(__name__)
+
+
+def postprocess_qa_predictions(
+ examples,
+ features,
+ predictions: Tuple[np.ndarray, np.ndarray],
+ version_2_with_negative: bool = False,
+ n_best_size: int = 20,
+ max_answer_length: int = 30,
+ null_score_diff_threshold: float = 0.0,
+ output_dir: Optional[str] = None,
+ prefix: Optional[str] = None,
+ log_level: Optional[int] = logging.WARNING,
+):
+ """
+ Post-processes the predictions of a question-answering model to convert them to answers that are substrings of the
+ original contexts. This is the base postprocessing functions for models that only return start and end logits.
+
+ Args:
+ examples: The non-preprocessed dataset (see the main script for more information).
+ features: The processed dataset (see the main script for more information).
+ predictions (:obj:`Tuple[np.ndarray, np.ndarray]`):
+ The predictions of the model: two arrays containing the start logits and the end logits respectively. Its
+ first dimension must match the number of elements of :obj:`features`.
+ version_2_with_negative (:obj:`bool`, `optional`, defaults to :obj:`False`):
+ Whether or not the underlying dataset contains examples with no answers.
+ n_best_size (:obj:`int`, `optional`, defaults to 20):
+ The total number of n-best predictions to generate when looking for an answer.
+ max_answer_length (:obj:`int`, `optional`, defaults to 30):
+ The maximum length of an answer that can be generated. This is needed because the start and end predictions
+ are not conditioned on one another.
+ null_score_diff_threshold (:obj:`float`, `optional`, defaults to 0):
+ The threshold used to select the null answer: if the best answer has a score that is less than the score of
+ the null answer minus this threshold, the null answer is selected for this example (note that the score of
+ the null answer for an example giving several features is the minimum of the scores for the null answer on
+ each feature: all features must be aligned on the fact they `want` to predict a null answer).
+
+ Only useful when :obj:`version_2_with_negative` is :obj:`True`.
+ output_dir (:obj:`str`, `optional`):
+ If provided, the dictionaries of predictions, n_best predictions (with their scores and logits) and, if
+ :obj:`version_2_with_negative=True`, the dictionary of the scores differences between best and null
+ answers, are saved in `output_dir`.
+ prefix (:obj:`str`, `optional`):
+ If provided, the dictionaries mentioned above are saved with `prefix` added to their names.
+ log_level (:obj:`int`, `optional`, defaults to ``logging.WARNING``):
+ ``logging`` log level (e.g., ``logging.WARNING``)
+ """
+ if len(predictions) != 2:
+ raise ValueError("`predictions` should be a tuple with two elements (start_logits, end_logits).")
+ all_start_logits, all_end_logits = predictions
+
+ if len(predictions[0]) != len(features):
+ raise ValueError(f"Got {len(predictions[0])} predictions and {len(features)} features.")
+
+ # Build a map example to its corresponding features.
+ example_id_to_index = {k: i for i, k in enumerate(examples["id"])}
+ features_per_example = collections.defaultdict(list)
+ for i, feature in enumerate(features):
+ features_per_example[example_id_to_index[feature["example_id"]]].append(i)
+
+ # The dictionaries we have to fill.
+ all_predictions = collections.OrderedDict()
+ all_nbest_json = collections.OrderedDict()
+ if version_2_with_negative:
+ scores_diff_json = collections.OrderedDict()
+
+ # Logging.
+ logger.setLevel(log_level)
+ logger.info(f"Post-processing {len(examples)} example predictions split into {len(features)} features.")
+
+ # Let's loop over all the examples!
+ for example_index, example in enumerate(tqdm(examples)):
+ # Those are the indices of the features associated to the current example.
+ feature_indices = features_per_example[example_index]
+
+ min_null_prediction = None
+ prelim_predictions = []
+
+ # Looping through all the features associated to the current example.
+ for feature_index in feature_indices:
+ # We grab the predictions of the model for this feature.
+ start_logits = all_start_logits[feature_index]
+ end_logits = all_end_logits[feature_index]
+ # This is what will allow us to map some the positions in our logits to span of texts in the original
+ # context.
+ offset_mapping = features[feature_index]["offset_mapping"]
+ # Optional `token_is_max_context`, if provided we will remove answers that do not have the maximum context
+ # available in the current feature.
+ token_is_max_context = features[feature_index].get("token_is_max_context", None)
+
+ # Update minimum null prediction.
+ feature_null_score = start_logits[0] + end_logits[0]
+ if min_null_prediction is None or min_null_prediction["score"] > feature_null_score:
+ min_null_prediction = {
+ "offsets": (0, 0),
+ "score": feature_null_score,
+ "start_logit": start_logits[0],
+ "end_logit": end_logits[0],
+ }
+
+ # Go through all possibilities for the `n_best_size` greater start and end logits.
+ start_indexes = np.argsort(start_logits)[-1 : -n_best_size - 1 : -1].tolist()
+ end_indexes = np.argsort(end_logits)[-1 : -n_best_size - 1 : -1].tolist()
+ for start_index in start_indexes:
+ for end_index in end_indexes:
+ # Don't consider out-of-scope answers, either because the indices are out of bounds or correspond
+ # to part of the input_ids that are not in the context.
+ if (
+ start_index >= len(offset_mapping)
+ or end_index >= len(offset_mapping)
+ or offset_mapping[start_index] is None
+ or len(offset_mapping[start_index]) < 2
+ or offset_mapping[end_index] is None
+ or len(offset_mapping[end_index]) < 2
+ ):
+ continue
+ # Don't consider answers with a length that is either < 0 or > max_answer_length.
+ if end_index < start_index or end_index - start_index + 1 > max_answer_length:
+ continue
+ # Don't consider answer that don't have the maximum context available (if such information is
+ # provided).
+ if token_is_max_context is not None and not token_is_max_context.get(str(start_index), False):
+ continue
+
+ prelim_predictions.append(
+ {
+ "offsets": (offset_mapping[start_index][0], offset_mapping[end_index][1]),
+ "score": start_logits[start_index] + end_logits[end_index],
+ "start_logit": start_logits[start_index],
+ "end_logit": end_logits[end_index],
+ }
+ )
+ if version_2_with_negative and min_null_prediction is not None:
+ # Add the minimum null prediction
+ prelim_predictions.append(min_null_prediction)
+ null_score = min_null_prediction["score"]
+
+ # Only keep the best `n_best_size` predictions.
+ predictions = sorted(prelim_predictions, key=lambda x: x["score"], reverse=True)[:n_best_size]
+
+ # Add back the minimum null prediction if it was removed because of its low score.
+ if (
+ version_2_with_negative
+ and min_null_prediction is not None
+ and not any(p["offsets"] == (0, 0) for p in predictions)
+ ):
+ predictions.append(min_null_prediction)
+
+ # Use the offsets to gather the answer text in the original context.
+ context = example["context"]
+ for pred in predictions:
+ offsets = pred.pop("offsets")
+ pred["text"] = context[offsets[0] : offsets[1]]
+
+ # In the very rare edge case we have not a single non-null prediction, we create a fake prediction to avoid
+ # failure.
+ if len(predictions) == 0 or (len(predictions) == 1 and predictions[0]["text"] == ""):
+ predictions.insert(0, {"text": "empty", "start_logit": 0.0, "end_logit": 0.0, "score": 0.0})
+
+ # Compute the softmax of all scores (we do it with numpy to stay independent from torch/tf in this file, using
+ # the LogSumExp trick).
+ scores = np.array([pred.pop("score") for pred in predictions])
+ exp_scores = np.exp(scores - np.max(scores))
+ probs = exp_scores / exp_scores.sum()
+
+ # Include the probabilities in our predictions.
+ for prob, pred in zip(probs, predictions):
+ pred["probability"] = prob
+
+ # Pick the best prediction. If the null answer is not possible, this is easy.
+ if not version_2_with_negative:
+ all_predictions[example["id"]] = predictions[0]["text"]
+ else:
+ # Otherwise we first need to find the best non-empty prediction.
+ i = 0
+ while predictions[i]["text"] == "":
+ i += 1
+ best_non_null_pred = predictions[i]
+
+ # Then we compare to the null prediction using the threshold.
+ score_diff = null_score - best_non_null_pred["start_logit"] - best_non_null_pred["end_logit"]
+ scores_diff_json[example["id"]] = float(score_diff) # To be JSON-serializable.
+ if score_diff > null_score_diff_threshold:
+ all_predictions[example["id"]] = ""
+ else:
+ all_predictions[example["id"]] = best_non_null_pred["text"]
+
+ # Make `predictions` JSON-serializable by casting np.float back to float.
+ all_nbest_json[example["id"]] = [
+ {k: (float(v) if isinstance(v, (np.float16, np.float32, np.float64)) else v) for k, v in pred.items()}
+ for pred in predictions
+ ]
+
+ # If we have an output_dir, let's save all those dicts.
+ if output_dir is not None:
+ if not os.path.isdir(output_dir):
+ raise EnvironmentError(f"{output_dir} is not a directory.")
+
+ prediction_file = os.path.join(
+ output_dir, "predictions.json" if prefix is None else f"{prefix}_predictions.json"
+ )
+ nbest_file = os.path.join(
+ output_dir, "nbest_predictions.json" if prefix is None else f"{prefix}_nbest_predictions.json"
+ )
+ if version_2_with_negative:
+ null_odds_file = os.path.join(
+ output_dir, "null_odds.json" if prefix is None else f"{prefix}_null_odds.json"
+ )
+
+ logger.info(f"Saving predictions to {prediction_file}.")
+ with open(prediction_file, "w") as writer:
+ writer.write(json.dumps(all_predictions, indent=4) + "\n")
+ logger.info(f"Saving nbest_preds to {nbest_file}.")
+ with open(nbest_file, "w") as writer:
+ writer.write(json.dumps(all_nbest_json, indent=4) + "\n")
+ if version_2_with_negative:
+ logger.info(f"Saving null_odds to {null_odds_file}.")
+ with open(null_odds_file, "w") as writer:
+ writer.write(json.dumps(scores_diff_json, indent=4) + "\n")
+
+ return all_predictions
+
+
+def postprocess_qa_predictions_with_beam_search(
+ examples,
+ features,
+ predictions: Tuple[np.ndarray, np.ndarray],
+ version_2_with_negative: bool = False,
+ n_best_size: int = 20,
+ max_answer_length: int = 30,
+ start_n_top: int = 5,
+ end_n_top: int = 5,
+ output_dir: Optional[str] = None,
+ prefix: Optional[str] = None,
+ log_level: Optional[int] = logging.WARNING,
+):
+ """
+ Post-processes the predictions of a question-answering model with beam search to convert them to answers that are substrings of the
+ original contexts. This is the postprocessing functions for models that return start and end logits, indices, as well as
+ cls token predictions.
+
+ Args:
+ examples: The non-preprocessed dataset (see the main script for more information).
+ features: The processed dataset (see the main script for more information).
+ predictions (:obj:`Tuple[np.ndarray, np.ndarray]`):
+ The predictions of the model: two arrays containing the start logits and the end logits respectively. Its
+ first dimension must match the number of elements of :obj:`features`.
+ version_2_with_negative (:obj:`bool`, `optional`, defaults to :obj:`False`):
+ Whether or not the underlying dataset contains examples with no answers.
+ n_best_size (:obj:`int`, `optional`, defaults to 20):
+ The total number of n-best predictions to generate when looking for an answer.
+ max_answer_length (:obj:`int`, `optional`, defaults to 30):
+ The maximum length of an answer that can be generated. This is needed because the start and end predictions
+ are not conditioned on one another.
+ start_n_top (:obj:`int`, `optional`, defaults to 5):
+ The number of top start logits too keep when searching for the :obj:`n_best_size` predictions.
+ end_n_top (:obj:`int`, `optional`, defaults to 5):
+ The number of top end logits too keep when searching for the :obj:`n_best_size` predictions.
+ output_dir (:obj:`str`, `optional`):
+ If provided, the dictionaries of predictions, n_best predictions (with their scores and logits) and, if
+ :obj:`version_2_with_negative=True`, the dictionary of the scores differences between best and null
+ answers, are saved in `output_dir`.
+ prefix (:obj:`str`, `optional`):
+ If provided, the dictionaries mentioned above are saved with `prefix` added to their names.
+ log_level (:obj:`int`, `optional`, defaults to ``logging.WARNING``):
+ ``logging`` log level (e.g., ``logging.WARNING``)
+ """
+ if len(predictions) != 5:
+ raise ValueError("`predictions` should be a tuple with five elements.")
+ start_top_log_probs, start_top_index, end_top_log_probs, end_top_index, cls_logits = predictions
+
+ if len(predictions[0]) != len(features):
+ raise ValueError(f"Got {len(predictions[0])} predictions and {len(features)} features.")
+
+ # Build a map example to its corresponding features.
+ example_id_to_index = {k: i for i, k in enumerate(examples["id"])}
+ features_per_example = collections.defaultdict(list)
+ for i, feature in enumerate(features):
+ features_per_example[example_id_to_index[feature["example_id"]]].append(i)
+
+ # The dictionaries we have to fill.
+ all_predictions = collections.OrderedDict()
+ all_nbest_json = collections.OrderedDict()
+ scores_diff_json = collections.OrderedDict() if version_2_with_negative else None
+
+ # Logging.
+ logger.setLevel(log_level)
+ logger.info(f"Post-processing {len(examples)} example predictions split into {len(features)} features.")
+
+ # Let's loop over all the examples!
+ for example_index, example in enumerate(tqdm(examples)):
+ # Those are the indices of the features associated to the current example.
+ feature_indices = features_per_example[example_index]
+
+ min_null_score = None
+ prelim_predictions = []
+
+ # Looping through all the features associated to the current example.
+ for feature_index in feature_indices:
+ # We grab the predictions of the model for this feature.
+ start_log_prob = start_top_log_probs[feature_index]
+ start_indexes = start_top_index[feature_index]
+ end_log_prob = end_top_log_probs[feature_index]
+ end_indexes = end_top_index[feature_index]
+ feature_null_score = cls_logits[feature_index]
+ # This is what will allow us to map some the positions in our logits to span of texts in the original
+ # context.
+ offset_mapping = features[feature_index]["offset_mapping"]
+ # Optional `token_is_max_context`, if provided we will remove answers that do not have the maximum context
+ # available in the current feature.
+ token_is_max_context = features[feature_index].get("token_is_max_context", None)
+
+ # Update minimum null prediction
+ if min_null_score is None or feature_null_score < min_null_score:
+ min_null_score = feature_null_score
+
+ # Go through all possibilities for the `n_start_top`/`n_end_top` greater start and end logits.
+ for i in range(start_n_top):
+ for j in range(end_n_top):
+ start_index = int(start_indexes[i])
+ j_index = i * end_n_top + j
+ end_index = int(end_indexes[j_index])
+ # Don't consider out-of-scope answers (last part of the test should be unnecessary because of the
+ # p_mask but let's not take any risk)
+ if (
+ start_index >= len(offset_mapping)
+ or end_index >= len(offset_mapping)
+ or offset_mapping[start_index] is None
+ or len(offset_mapping[start_index]) < 2
+ or offset_mapping[end_index] is None
+ or len(offset_mapping[end_index]) < 2
+ ):
+ continue
+
+ # Don't consider answers with a length negative or > max_answer_length.
+ if end_index < start_index or end_index - start_index + 1 > max_answer_length:
+ continue
+ # Don't consider answer that don't have the maximum context available (if such information is
+ # provided).
+ if token_is_max_context is not None and not token_is_max_context.get(str(start_index), False):
+ continue
+ prelim_predictions.append(
+ {
+ "offsets": (offset_mapping[start_index][0], offset_mapping[end_index][1]),
+ "score": start_log_prob[i] + end_log_prob[j_index],
+ "start_log_prob": start_log_prob[i],
+ "end_log_prob": end_log_prob[j_index],
+ }
+ )
+
+ # Only keep the best `n_best_size` predictions.
+ predictions = sorted(prelim_predictions, key=lambda x: x["score"], reverse=True)[:n_best_size]
+
+ # Use the offsets to gather the answer text in the original context.
+ context = example["context"]
+ for pred in predictions:
+ offsets = pred.pop("offsets")
+ pred["text"] = context[offsets[0] : offsets[1]]
+
+ # In the very rare edge case we have not a single non-null prediction, we create a fake prediction to avoid
+ # failure.
+ if len(predictions) == 0:
+ # Without predictions min_null_score is going to be None and None will cause an exception later
+ min_null_score = -2e-6
+ predictions.insert(0, {"text": "", "start_logit": -1e-6, "end_logit": -1e-6, "score": min_null_score})
+
+ # Compute the softmax of all scores (we do it with numpy to stay independent from torch/tf in this file, using
+ # the LogSumExp trick).
+ scores = np.array([pred.pop("score") for pred in predictions])
+ exp_scores = np.exp(scores - np.max(scores))
+ probs = exp_scores / exp_scores.sum()
+
+ # Include the probabilities in our predictions.
+ for prob, pred in zip(probs, predictions):
+ pred["probability"] = prob
+
+ # Pick the best prediction and set the probability for the null answer.
+ all_predictions[example["id"]] = predictions[0]["text"]
+ if version_2_with_negative:
+ scores_diff_json[example["id"]] = float(min_null_score)
+
+ # Make `predictions` JSON-serializable by casting np.float back to float.
+ all_nbest_json[example["id"]] = [
+ {k: (float(v) if isinstance(v, (np.float16, np.float32, np.float64)) else v) for k, v in pred.items()}
+ for pred in predictions
+ ]
+
+ # If we have an output_dir, let's save all those dicts.
+ if output_dir is not None:
+ if not os.path.isdir(output_dir):
+ raise EnvironmentError(f"{output_dir} is not a directory.")
+
+ prediction_file = os.path.join(
+ output_dir, "predictions.json" if prefix is None else f"{prefix}_predictions.json"
+ )
+ nbest_file = os.path.join(
+ output_dir, "nbest_predictions.json" if prefix is None else f"{prefix}_nbest_predictions.json"
+ )
+ if version_2_with_negative:
+ null_odds_file = os.path.join(
+ output_dir, "null_odds.json" if prefix is None else f"{prefix}_null_odds.json"
+ )
+
+ logger.info(f"Saving predictions to {prediction_file}.")
+ with open(prediction_file, "w") as writer:
+ writer.write(json.dumps(all_predictions, indent=4) + "\n")
+ logger.info(f"Saving nbest_preds to {nbest_file}.")
+ with open(nbest_file, "w") as writer:
+ writer.write(json.dumps(all_nbest_json, indent=4) + "\n")
+ if version_2_with_negative:
+ logger.info(f"Saving null_odds to {null_odds_file}.")
+ with open(null_odds_file, "w") as writer:
+ writer.write(json.dumps(scores_diff_json, indent=4) + "\n")
+
+ return all_predictions, scores_diff_json
\ No newline at end of file
diff --git a/nlp/requirements.txt b/nlp/requirements.txt
new file mode 100644
index 000000000..3f3af16a2
--- /dev/null
+++ b/nlp/requirements.txt
@@ -0,0 +1,18 @@
+pydantic==1.10.8
+datasets==2.12.0
+evaluate==0.4.0
+mlflow==2.3.2
+accelerate==0.20.3
+nltk==3.8.1
+torch==2.0.1
+transformers==4.29.2
+pandas==2.0.2
+modin==0.22.1
+wordcloud==1.9.2
+matplotlib==3.7.1
+optimum[neural-compressor]==1.8.7
+neural-compressor==2.1.1
+webvtt-py==0.4.6
+intel_extension_for_pytorch==2.0.100
+scikit-learn==1.2.2
+scikit-learn-intelex==2023.1.1
diff --git a/nlp/utils/preprocess_subtitle.py b/nlp/utils/preprocess_subtitle.py
new file mode 100644
index 000000000..12d8bb1d0
--- /dev/null
+++ b/nlp/utils/preprocess_subtitle.py
@@ -0,0 +1,90 @@
+
+#!/usr/bin/env python
+# coding=utf-8
+# Copyright 2023 C5ailabs Team (Authors: Rohit Sroch) All rights reserved.
+#
+# Licensed under the Apache License, Version 2.0 (the "License");
+# you may not use this file except in compliance with the License.
+# You may obtain a copy of the License at
+#
+# http://www.apache.org/licenses/LICENSE-2.0
+#
+# Unless required by applicable law or agreed to in writing, software
+# distributed under the License is distributed on an "AS IS" BASIS,
+# WITHOUT WARRANTIES OR CONDITIONS OF ANY KIND, either express or implied.
+# See the License for the specific language governing permissions and
+# limitations under the License.
+"""
+Preprocess course video subtitle (.vtt) file.
+"""
+import argparse
+import os
+import webvtt
+from glob import glob
+
+def preprocess_subtitle_vtt(subtitle_fpath, min_text_len=20):
+ subtitles = webvtt.read(subtitle_fpath)
+
+ preprocessed_subtitles = []
+ current_subtitle = ""
+ current_start = ""
+
+ for subtitle in subtitles:
+ subtitle_text = " ".join(subtitle.text.strip().split("\n")).strip()
+ current_subtitle += subtitle_text + " "
+
+ if len(current_subtitle) >= min_text_len:
+ preprocessed_subtitles.append(webvtt.Caption(current_start, subtitle.end, text=current_subtitle.strip()))
+ current_subtitle = ""
+ current_start = ""
+ elif not current_start:
+ current_start = subtitle.start
+
+ if current_subtitle:
+ preprocessed_subtitles.append(webvtt.Caption(current_start, subtitle.end, text=current_subtitle.strip()))
+
+ psubtitle = webvtt.WebVTT()
+ psubtitle.captions.extend(preprocessed_subtitles)
+
+ return psubtitle
+
+
+def main(args):
+
+ # format of courses folder structure is courses/{topic_name}/Study-Material/{week_name}/{subtopic_name}/subtitle-en.vtt
+ path = os.path.join(args.course_dir, "*/Study-Material/*/*/*.vtt")
+ subtitle_fpaths = glob(path)
+
+ for subtitle_fpath in subtitle_fpaths:
+ print("*"*100)
+ print("Preprocess subtitle: {}".format(subtitle_fpath))
+ psubtitle = preprocess_subtitle_vtt(
+ subtitle_fpath, args.min_text_len)
+
+ psubtitle_fpath = subtitle_fpath.replace(
+ ".vtt", "-processed.vtt")
+ #psubtitle_fpath = subtitle_fpath
+ psubtitle.save(psubtitle_fpath)
+ print("\n")
+
+
+if __name__ == "__main__":
+
+ parser = argparse.ArgumentParser(description="Preprocess course video subtitle (.vtt) file")
+
+ parser.add_argument(
+ "--course_dir",
+ type=str,
+ help="base directory containing courses",
+ default="../../dataset/courses"
+ )
+ parser.add_argument(
+ "--min_text_len",
+ type=int,
+ default=500,
+ help="Minimum length of each subtitle text (in chars)"
+ )
+
+ args = parser.parse_args()
+
+ main(args)
\ No newline at end of file
diff --git a/nlp/utils/run_eda.py b/nlp/utils/run_eda.py
new file mode 100644
index 000000000..78fbd5623
--- /dev/null
+++ b/nlp/utils/run_eda.py
@@ -0,0 +1,94 @@
+
+#!/usr/bin/env python
+# coding=utf-8
+# Copyright 2023 C5ailabs Team All rights reserved.
+#
+# Licensed under the Apache License, Version 2.0 (the "License");
+# you may not use this file except in compliance with the License.
+# You may obtain a copy of the License at
+#
+# http://www.apache.org/licenses/LICENSE-2.0
+#
+# Unless required by applicable law or agreed to in writing, software
+# distributed under the License is distributed on an "AS IS" BASIS,
+# WITHOUT WARRANTIES OR CONDITIONS OF ANY KIND, either express or implied.
+# See the License for the specific language governing permissions and
+# limitations under the License.
+"""
+Perform Basic EDA for course content.
+"""
+import argparse
+import os
+import webvtt
+import modin.pandas as pd
+import matplotlib.pyplot as plt
+from wordcloud import WordCloud
+from sklearn.feature_extraction.text import TfidfVectorizer
+from glob import glob
+
+def read_subtitle_vtt(subtitle_fpaths):
+
+ subtitle_data = []
+ for subtitle_fpath in subtitle_fpaths:
+ subtitles = webvtt.read(subtitle_fpath)
+ for idx, caption in enumerate(subtitles):
+ subtitle_data.append([idx, caption.text])
+
+ df = pd.DataFrame(subtitle_data, columns=['index', 'caption_text'])
+
+ return df
+
+def extract_top_phrases_tfidf(df, text_column):
+ vectorizer = TfidfVectorizer(stop_words="english", ngram_range=(1, 1), max_df=0.5, min_df=0.005)
+ df = df[df[text_column].notna()]
+ df[text_column] = df[text_column].astype(str).str.lower()
+ text_data = df[text_column].values
+ tfidf_matrix = vectorizer.fit_transform(text_data).toarray()
+ df_top_phrases = pd.DataFrame(tfidf_matrix, columns=vectorizer.get_feature_names_out())
+ top_phrases = df_top_phrases.astype(bool).sum(axis=0).sort_values(ascending=False).index
+ df_top_phrases = df_top_phrases.astype(bool).sum(axis=0).sort_values(ascending=False)
+
+ return df_top_phrases, top_phrases, df
+
+def generate_wordcloud(phrase_counts):
+ if len(phrase_counts) > 100:
+ wc = WordCloud(
+ width=800, height=400, max_words=100, background_color="white").generate_from_frequencies(
+ phrase_counts[0:100])
+ else:
+ wc = WordCloud(
+ width=800, height=400, max_words=100, background_color="white").generate_from_frequencies(
+ phrase_counts[0:len(phrase_counts)])
+ plt.figure(figsize=(10, 20))
+ plt.imshow(wc, interpolation='bilinear')
+ plt.axis('off')
+
+ return plt
+
+def main(args):
+
+ path = os.path.join(args.course_dir, "*/Study-Material/*/*/*.vtt")
+ subtitle_fpaths = glob(path)
+
+ from distributed import Client
+ client = Client()
+
+ df = read_subtitle_vtt(subtitle_fpaths)
+
+ phrase_counts, _, _ = extract_top_phrases_tfidf(df, 'caption_text')
+ wordcloud = generate_wordcloud(phrase_counts)
+ plt.savefig('EDA-Worldcloud.png', bbox_inches='tight')
+
+if __name__ == "__main__":
+ parser = argparse.ArgumentParser(description='Perform Basic EDA on given dataset')
+
+ parser.add_argument(
+ "--course_dir",
+ type=str,
+ help="base directory containing courses",
+ default="../../dataset/courses"
+ )
+
+ args = parser.parse_args()
+ main(args)
+
diff --git a/ppt/.DS_Store b/ppt/.DS_Store
new file mode 100644
index 000000000..7d8b6fab3
Binary files /dev/null and b/ppt/.DS_Store differ
diff --git a/ppt/Intel-oneAPI-Hackathon-Implementation.pdf b/ppt/Intel-oneAPI-Hackathon-Implementation.pdf
new file mode 100644
index 000000000..e096e80e5
Binary files /dev/null and b/ppt/Intel-oneAPI-Hackathon-Implementation.pdf differ
diff --git a/webapp/.DS_Store b/webapp/.DS_Store
new file mode 100644
index 000000000..8137e800c
Binary files /dev/null and b/webapp/.DS_Store differ
diff --git a/webapp/.streamlit/config.toml b/webapp/.streamlit/config.toml
new file mode 100644
index 000000000..6f00fb12f
--- /dev/null
+++ b/webapp/.streamlit/config.toml
@@ -0,0 +1,19 @@
+[theme]
+
+# Used to style primary interface elements. It's the color displayed most frequently across your app's
+# screens and components. Examples of widgets using this color are st.slider and st.checkbox.
+primaryColor = "#ff531a"
+
+# Background color for the main container.
+backgroundColor = "#ecf2f9"
+
+# Used as the background for most widgets. Examples of widgets with this background are st.sidebar,
+# st.text_input, st.date_input.
+secondaryBackgroundColor = "#f2e6d9"
+
+# Font color for the page.
+textColor = "#323234"
+
+# Font family (serif | sans serif | mono) for the page. Will not impact code areas.
+# Default: "sans serif"
+font = "sans serif"
\ No newline at end of file
diff --git a/webapp/.streamlit/secrets.toml b/webapp/.streamlit/secrets.toml
new file mode 100644
index 000000000..4ef0c962b
--- /dev/null
+++ b/webapp/.streamlit/secrets.toml
@@ -0,0 +1,5 @@
+# .streamlit/secrets.toml
+
+[passwords]
+# Follow the rule: username = "password"
+admin = "Admin@123"
\ No newline at end of file
diff --git a/webapp/Dockerfile b/webapp/Dockerfile
new file mode 100644
index 000000000..2ab703848
--- /dev/null
+++ b/webapp/Dockerfile
@@ -0,0 +1,13 @@
+FROM python:3.9
+
+WORKDIR /opt
+COPY . .
+
+RUN pip install --upgrade pip
+
+RUN pip install -r requirements.txt
+
+EXPOSE 8502
+ENV PORT 8502
+
+ENTRYPOINT ["streamlit", "run", "app.py", "--server.port=8502", "--server.address=0.0.0.0"]
\ No newline at end of file
diff --git a/webapp/app.py b/webapp/app.py
new file mode 100644
index 000000000..60966bfb2
--- /dev/null
+++ b/webapp/app.py
@@ -0,0 +1,124 @@
+import streamlit as st
+
+from PIL import Image
+from pathlib import Path
+from config import BASE_DIR
+from ui.ui_manager import *
+
+from utils.logging_handler import Logger
+
+def write_header():
+ """Writes the header part of the UI
+ """
+ # st.markdown("---")
+ st.title(':blue[LEAP (Learning Enhancement and Assistance Platform)]')
+ # st.markdown('''
+ # - Intel One API hackathon implementation of LEAP platform
+ # ''')
+
+
+def write_footer():
+ """Writes the footer part of the UI
+ """
+ st.sidebar.markdown("---")
+ st.sidebar.warning("Please note that this tool is only for demo purpose")
+ st.sidebar.text('''
+ © Copyright 2023, Course5 AI Labs.
+ ''')
+
+
+def write_ui():
+ """Handles the major part of the UI
+ """
+
+ # Sets up the basic UI with title and logo
+ st.sidebar.title("Course5 AI Labs")
+ img = Image.open(Path(BASE_DIR) / 'imgs/c5-logo.jpeg')
+ st.sidebar.image(img)
+
+ if "demo_started" not in st.session_state:
+ # Handles the initial page which displays the process flow
+ st.session_state["demo_started"] = False
+ img = Image.open(Path(BASE_DIR) / 'imgs/process-flow.png')
+ st.image(img, use_column_width=True)
+ if st.button(label="Start Demo", use_container_width=True):
+ st.session_state["demo_started"] = True
+ elif "viva_mode" in st.session_state:
+ display_course_banner(st.session_state["course_selected"])
+ st.markdown("---")
+ display_viva_chat_bot(st.session_state["course_selected"])
+ elif "course_selected" not in st.session_state:
+ # Handles the page to display the course selection page
+ st.markdown("---")
+ display_courses()
+ elif "video_selected" not in st.session_state:
+ # Handles the page to display course contents from which a video can be selected
+ display_course_banner(st.session_state["course_selected"])
+ display_video_tabs(st.session_state["course_selected"])
+ else:
+ # Handles the UI to have Q&A on selected video
+ st.markdown("---")
+ video_selected = st.session_state["video_selected"]
+ # Display video and subtitles on UI
+ display_video_content(Path(video_selected))
+ # Chat window
+ display_qa_chat_bot()
+
+
+def check_password():
+ """Returns `True` if the user had a correct password."""
+
+ def password_entered():
+ """Checks whether a password entered by the user is correct."""
+ if (st.session_state["username"] in st.secrets["passwords"]
+ and st.session_state["password"]
+ == st.secrets["passwords"][st.session_state["username"]]):
+ st.session_state["password_correct"] = True
+ del st.session_state["password"] # don't store username + password
+ del st.session_state["username"]
+ else:
+ st.session_state["password_correct"] = False
+
+ if "password_correct" not in st.session_state:
+ # First run, show inputs for username + password.
+ st.text_input("Username", on_change=password_entered, key="username")
+ st.text_input("Password",
+ type="password",
+ on_change=password_entered,
+ key="password")
+ return False
+ elif not st.session_state["password_correct"]:
+ # Password not correct, show input + error.
+ st.text_input("Username", on_change=password_entered, key="username")
+ st.text_input("Password",
+ type="password",
+ on_change=password_entered,
+ key="password")
+ st.error("😕 User not known or password incorrect")
+ return False
+ else:
+ # Password correct.
+ return True
+
+def production_mode():
+ hide_streamlit_style = """
+
+ """
+ st.markdown(hide_streamlit_style, unsafe_allow_html=True)
+ return
+
+if __name__ == '__main__':
+ img = Image.open(Path(BASE_DIR) / 'imgs/c5-favicon.jpeg')
+ st.set_page_config(page_title='LEAP',
+ page_icon=img,
+ layout='wide')
+
+ #if check_password():
+ #production_mode()
+ write_header()
+ write_ui()
+ write_footer()
diff --git a/webapp/common.py b/webapp/common.py
new file mode 100644
index 000000000..d31b7c37d
--- /dev/null
+++ b/webapp/common.py
@@ -0,0 +1,117 @@
+import os
+import streamlit as st
+import speech_recognition as sr
+from datetime import datetime
+from speech_recognition import UnknownValueError
+
+from utils.logging_handler import Logger
+
+@st.cache_data
+def convert_stt(audio_bytes):
+ """Listens for any user input in chatbot UI. Inputs can be via text or via microphone.
+ """
+ recognized_text = None
+ if audio_bytes:
+ try:
+ Logger.info("Running STT...")
+ r = sr.Recognizer()
+ audio_data = sr.AudioData(
+ frame_data=audio_bytes, sample_rate=16000, sample_width=4)
+ recognized_text = str(
+ r.recognize_whisper(audio_data=audio_data))
+ Logger.info(f"STT recognized text: {recognized_text}")
+ except UnknownValueError as unrecognized_audio_error:
+ Logger.exception(unrecognized_audio_error)
+
+ return recognized_text
+
+def get_viva_context(base_dir, topic_name):
+ """Get the context for specific topic for Viva Exam
+ """
+ dir_path = os.path.join(base_dir, topic_name, "Viva-Material")
+ context = ""
+ for curr_path in os.listdir(dir_path):
+ if ".txt" in curr_path:
+ with open(os.path.join(dir_path, curr_path), "r", encoding='utf-8') as f:
+ data = f.read()
+ context += str(data) + "\n\n"
+
+ return context
+
+def load_course_material(base_dir):
+ """Load the course material
+ """
+ course_material = {"course_names": []}
+ # courses/{topic_name}/{material_type}/{week_name}/{sub_topic_name}/
+ for topic_name in sorted(os.listdir(base_dir)):
+ curr_dir = os.path.join(base_dir, topic_name)
+ if os.path.isdir(curr_dir) is False:
+ continue
+ course_material["course_names"].append(topic_name)
+ if topic_name not in course_material:
+ course_material[topic_name] = {}
+
+ for _type in sorted(os.listdir(curr_dir)):
+ _curr_dir = os.path.join(curr_dir, _type)
+
+ if os.path.isfile(_curr_dir):
+ if _type.split('.')[-1] in ["jpeg", "png", "jpg"]:
+ course_material[topic_name][
+ "logo_path"] = os.path.join(curr_dir, _type)
+ continue
+
+ if os.path.isdir(_curr_dir) is False:
+ continue
+
+ if _type == "Study-Material":
+ course_material[topic_name][_type] = {"week_names": []}
+
+ for week_name in sorted(os.listdir(_curr_dir)):
+ __curr_dir = os.path.join(_curr_dir, week_name)
+ if os.path.isdir(__curr_dir) is False:
+ continue
+
+ course_material[topic_name][_type]["week_names"].append(week_name)
+ course_material[topic_name][_type][week_name] = {"subtopic_names": []}
+ for subtopic_name in sorted(os.listdir(__curr_dir)):
+ ___curr_dir = os.path.join(__curr_dir, subtopic_name)
+ if os.path.isdir(___curr_dir) is False:
+ continue
+
+ course_material[topic_name][_type][week_name]["subtopic_names"].append(
+ subtopic_name)
+ if subtopic_name not in course_material[topic_name][_type][week_name]:
+ course_material[topic_name][_type][week_name][subtopic_name] = {}
+
+ for file_name in sorted(os.listdir(___curr_dir)):
+ file_path = os.path.join(___curr_dir, file_name)
+ extension = file_name.split('.')[-1]
+ if os.path.isfile(file_path):
+ if extension == "mp4":
+ course_material[topic_name][_type][week_name][subtopic_name]["video_file"] = file_path
+ elif extension == "pdf":
+ course_material[topic_name][_type][week_name][subtopic_name]["doc_file"] = file_path
+ elif extension == "vtt":
+ course_material[topic_name][_type][week_name][subtopic_name]["subtitle_file"] = file_path
+
+ elif _type == "Viva-Material":
+ course_material[topic_name][_type] = {"context_files": []}
+ for file_name in sorted(os.listdir(_curr_dir)):
+ file_path = os.path.join(_curr_dir, file_name)
+ extension = file_name.split('.')[-1]
+ if os.path.isfile(file_path):
+ if extension == "txt":
+ course_material[topic_name][_type]["context_files"].append(
+ file_path
+ )
+
+ return course_material
+
+
+def time_to_seconds(time_string):
+ # Parse the time string and create a datetime object
+ time_obj = datetime.strptime(time_string, "%H:%M:%S.%f")
+
+ # Extract the total seconds from the datetime object
+ seconds = (time_obj.hour * 3600) + (time_obj.minute * 60) + time_obj.second + (time_obj.microsecond / 1000000)
+ return int(seconds)
diff --git a/webapp/config.py b/webapp/config.py
new file mode 100644
index 000000000..f8a9b9324
--- /dev/null
+++ b/webapp/config.py
@@ -0,0 +1,16 @@
+BASE_DIR = 'static'
+DATASET_COURSE_BASE_DIR = "./dataset/courses/"
+
+API_CONFIG = {
+ "server_host": "leap-api",
+ "server_port": "8500",
+ "ask_doubt": {
+ "max_answer_length": 30,
+ "max_seq_length": 384,
+ "top_n": 2,
+ "top_k": 1
+ },
+ "ai_examiner": {
+ "viva_ask_question_types": ["Open Ended", "Single Choice", "Multiple Choice", "Yes or No Choice"]
+ }
+}
\ No newline at end of file
diff --git a/webapp/requirements.txt b/webapp/requirements.txt
new file mode 100644
index 000000000..c3d301bbc
--- /dev/null
+++ b/webapp/requirements.txt
@@ -0,0 +1,12 @@
+streamlit==1.24.0
+streamlit-chat==0.1.1
+Pillow==9.5.0
+audio_recorder_streamlit==0.0.8
+openai-whisper==20230314
+soundfile==0.12.1
+PyYAML==6.0
+moviepy==1.0.3
+pydub==0.25.1
+SpeechRecognition==3.10.0
+requests==2.31.0
+webvtt-py==0.4.6
\ No newline at end of file
diff --git a/webapp/static/.DS_Store b/webapp/static/.DS_Store
new file mode 100644
index 000000000..9acdb4a37
Binary files /dev/null and b/webapp/static/.DS_Store differ
diff --git a/webapp/static/.gitignore b/webapp/static/.gitignore
new file mode 100644
index 000000000..e69de29bb
diff --git a/webapp/static/csv/.gitignore b/webapp/static/csv/.gitignore
new file mode 100644
index 000000000..e69de29bb
diff --git a/webapp/static/imgs/.DS_Store b/webapp/static/imgs/.DS_Store
new file mode 100644
index 000000000..97d867c8a
Binary files /dev/null and b/webapp/static/imgs/.DS_Store differ
diff --git a/webapp/static/imgs/.gitignore b/webapp/static/imgs/.gitignore
new file mode 100644
index 000000000..e69de29bb
diff --git a/webapp/static/imgs/c5-favicon.jpeg b/webapp/static/imgs/c5-favicon.jpeg
new file mode 100644
index 000000000..71275a4ad
Binary files /dev/null and b/webapp/static/imgs/c5-favicon.jpeg differ
diff --git a/webapp/static/imgs/c5-logo.jpeg b/webapp/static/imgs/c5-logo.jpeg
new file mode 100644
index 000000000..c854ed071
Binary files /dev/null and b/webapp/static/imgs/c5-logo.jpeg differ
diff --git a/webapp/static/imgs/process-flow.png b/webapp/static/imgs/process-flow.png
new file mode 100644
index 000000000..f8158207b
Binary files /dev/null and b/webapp/static/imgs/process-flow.png differ
diff --git a/webapp/static/imgs/user-icon.png b/webapp/static/imgs/user-icon.png
new file mode 100644
index 000000000..e54f14f6e
Binary files /dev/null and b/webapp/static/imgs/user-icon.png differ
diff --git a/webapp/static/video/.gitignore b/webapp/static/video/.gitignore
new file mode 100644
index 000000000..e69de29bb
diff --git a/webapp/static/widgets/.gitignore b/webapp/static/widgets/.gitignore
new file mode 100644
index 000000000..e69de29bb
diff --git a/webapp/ui/__init__.py b/webapp/ui/__init__.py
new file mode 100644
index 000000000..e69de29bb
diff --git a/webapp/ui/api_handler.py b/webapp/ui/api_handler.py
new file mode 100644
index 000000000..da08ec793
--- /dev/null
+++ b/webapp/ui/api_handler.py
@@ -0,0 +1,113 @@
+import requests
+
+from utils.logging_handler import Logger
+
+
+class PredictAskDoubt(object):
+ """Class to use AskDoubt API"""
+
+ def __init__(self, server_config):
+ self.server_config = server_config
+
+ # @st.cache_data
+ def predict_ask_doubt(self, payload):
+ """POST request"""
+
+ url = "http://{}:{}/leap/api/v1/ask-doubt".format(
+ self.server_config["server_host"],
+ self.server_config["server_port"])
+
+ Logger.info(f"Sending request to {url}")
+ Logger.info(f"Request payload: {payload}")
+ response = requests.post(url=url,
+ headers={
+ 'Content-Type': 'application/json',
+ },
+ json=payload)
+ Logger.info("Response: {response}")
+ processed_response = self._process_response(response=response)
+ Logger.info(f"Processed Response: {processed_response}")
+ return processed_response
+
+ def _process_response(self, response):
+ """Checks for API errors and returns a processed response.
+ Response will be a triplet containing answer, context, and meta-data.
+
+ Args:
+ response (_type_): _description_
+
+ Returns:
+ _type_: _description_
+ """
+ if response.status_code == 200:
+ data = response.json()
+ results = data["data"]
+ return self._find_answer(results)
+ else:
+ Logger.error("Error Message:", response.text)
+ return "Could not find answer, check your network...", None, None
+
+ def _find_answer(self, results):
+ """Processes response to get answer, context, and any meta-data for response.
+
+ Args:
+ results (_type_): _description_
+
+ Returns:
+ _type_: _description_
+ """
+ answer = results['answer']
+ relevant_context_id = results['relevant_context_id']
+ if answer:
+ if relevant_context_id > -1:
+ relevant_context = results['relevant_contexts'][relevant_context_id]
+ return answer, relevant_context['context'], relevant_context['metadata']
+ else:
+ return answer, None, None
+ else:
+ return "Sorry, I couldn't find an answer in course material", None, None
+
+class PredictAIExaminer(object):
+ """Class to use AIExaminer API"""
+
+ def __init__(self, server_config):
+ self.server_config = server_config
+
+ def predict_aiexaminer_ask_question(self, payload):
+ """POST request"""
+ url = "http://{}:{}/leap/api/v1/ai-examiner/ask-question".format(
+ self.server_config["server_host"],
+ self.server_config["server_port"])
+ response = requests.post(url=url,
+ headers={
+ 'Content-Type': 'application/json',
+ },
+ json=payload).json()
+
+ return response
+
+ def predict_aiexaminer_eval_answer(self, payload):
+ """POST request"""
+ url = "http://{}:{}/leap/api/v1/ai-examiner/eval-answer".format(
+ self.server_config["server_host"],
+ self.server_config["server_port"])
+ response = requests.post(url=url,
+ headers={
+ 'Content-Type': 'application/json',
+ },
+ json=payload).json()
+
+ return response
+
+ def predict_aiexaminer_hint_motivate(self, payload):
+ """POST request"""
+ url = "http://{}:{}/leap/api/v1/ai-examiner/hint-motivate".format(
+ self.server_config["server_host"],
+ self.server_config["server_port"])
+ response = requests.post(url=url,
+ headers={
+ 'Content-Type': 'application/json',
+ },
+ json=payload).json()
+
+ return response
\ No newline at end of file
diff --git a/webapp/ui/chatbot.py b/webapp/ui/chatbot.py
new file mode 100644
index 000000000..e1ff9fc1c
--- /dev/null
+++ b/webapp/ui/chatbot.py
@@ -0,0 +1,282 @@
+"""Class for managing chatbot in UI
+"""
+import random
+from pathlib import Path
+import streamlit as st
+
+from streamlit_chat import message
+from audio_recorder_streamlit import audio_recorder
+from common import convert_stt
+
+from ui.api_handler import PredictAskDoubt, PredictAIExaminer
+from common import get_viva_context
+from config import (
+ API_CONFIG,
+ DATASET_COURSE_BASE_DIR
+)
+
+from utils.logging_handler import Logger
+
+
+class Chatbot:
+ """Manages chatbot UI
+ """
+
+ def __init__(self, chat_box_label: str = "Ask Doubt:", viva_mode: bool = False, callback=None, **kwargs) -> None:
+ """Initializes a chat-bot on UI. In viva mode bot messages will be displayed before user messages.
+
+ Args:
+ chat_box_label (_type_, optional): _description_. Defaults to "Ask your queries:".
+ viva_mode (bool, optional): _description_. Defaults to False.
+ callback (_type_, optional): Function which will be called every time a new bot message is displayed on chat. Defaults to None.
+ """
+ if 'user_message' not in st.session_state:
+ st.session_state['user_message'] = []
+
+ if 'bot_message' not in st.session_state:
+ st.session_state['bot_message'] = []
+
+ if 'bot_message_context' not in st.session_state:
+ st.session_state['bot_message_context'] = []
+
+ if 'bot_message_meta_data' not in st.session_state:
+ st.session_state['bot_message_meta_data'] = []
+
+ if 'chat_input' not in st.session_state:
+ st.session_state['chat_input'] = None
+
+ # container for displaying older messages (before the last two conversations)
+ self.chat_history_container = st.expander("See history")
+ self.chat_container = st.container()
+
+ self.chat_box_label = chat_box_label
+ self.viva_mode = viva_mode
+ self.callback = callback
+ self.kwargs = kwargs
+ # self.chat_query = None
+ self.speech_input = None
+
+ # sets up the chatbot UI layout
+ Logger.info("Initializing the chat-bot UI")
+ self._init()
+
+ self.course_name = kwargs.get("selected_course", None)
+ Logger.info("Selected course: {}".format(self.course_name))
+
+ # for making API calls
+ self.ask_doubt = PredictAskDoubt(server_config=API_CONFIG)
+ self.ai_examiner = PredictAIExaminer(server_config=API_CONFIG)
+
+ def listen_for_inputs(self,):
+ """Generates a response for the input query and displays it in the chat UI.
+ """
+ if self.viva_mode:
+ # Initial question has to be from bot if in viva mode
+ if not st.session_state['bot_message']:
+ bot_message = """ 🎉👏 Congrats 🥳 on completing the course! Let's check your understanding around the topic `{}` with few questions. Here is the question:""".format(
+ self.course_name)
+ # call /leap/api/v1/ai-examiner/ask-question
+ context = get_viva_context(DATASET_COURSE_BASE_DIR, self.course_name)
+ question_type = random.choice(API_CONFIG["ai_examiner"]["viva_ask_question_types"])
+ payload = {
+ "topic": self.course_name,
+ "context": context,
+ "question_type": question_type
+ }
+ output = self.ai_examiner.predict_aiexaminer_ask_question(
+ payload)
+ ai_question = output["data"]["prediction"]["ai_question"]
+ st.session_state["ai_question"] = ai_question
+
+ bot_message += "\n\n" + ai_question
+ st.session_state['bot_message'].append(bot_message)
+ with self.chat_container:
+ self._display_message(message_index=len(
+ st.session_state['bot_message'])-1, is_user=False, more_info=True)
+
+ user_message = st.session_state['chat_input'] if st.session_state['chat_input'] else self.speech_input
+
+ if user_message:
+ if self.viva_mode:
+ st.session_state['user_message'].append(user_message)
+ # call /leap/api/v1/ai-examiner/eval-answer
+ ai_question = st.session_state["ai_question"]
+ payload = {
+ "topic": self.course_name,
+ "ai_question": ai_question,
+ "student_solution": user_message
+ }
+ output = self.ai_examiner.predict_aiexaminer_eval_answer(payload)
+ student_grade = output["data"]["prediction"]["student_grade"]
+
+ if student_grade == "Incorrect":
+ bot_message = "Well try, but your answer is ❌ Incorrect 😔\n\n"
+ # call /leap/api/v1/ai-examiner/hint-motivate
+ output = self.ai_examiner.predict_aiexaminer_hint_motivate(payload)
+ hint = output["data"]["prediction"]["hint"]
+ motivation = output["data"]["prediction"]["motivation"]
+ bot_message += "Hint: {}".format(hint) + "\n\n" + "🤛 {}".format(motivation)
+
+ st.session_state['bot_message'].append(bot_message)
+
+ st.session_state['chat_input'] = None
+ with self.chat_container:
+ self._display_message(message_index=len(
+ st.session_state['user_message'])-1, is_user=True)
+ self._display_message(message_index=len(
+ st.session_state['bot_message'])-1, is_user=False)
+ else:
+ bot_message = "Wow 🥳, That's a ✔️ correct answer. You are doing great! 🚀. Here is the another question:"
+
+ # call /leap/api/v1/ai-examiner/ask-question
+ context = get_viva_context(DATASET_COURSE_BASE_DIR, self.course_name)
+ question_type = random.choice(API_CONFIG["ai_examiner"]["viva_ask_question_types"])
+ payload = {
+ "topic": self.course_name,
+ "context": context,
+ "question_type": question_type
+ }
+ output = self.ai_examiner.predict_aiexaminer_ask_question(
+ payload)
+ ai_question = output["data"]["prediction"]["ai_question"]
+ st.session_state["ai_question"] = ai_question
+
+ bot_message += "\n\n" + ai_question
+ st.session_state['bot_message'].append(bot_message)
+
+ st.session_state['chat_input'] = None
+ with self.chat_container:
+ self._display_message(message_index=len(
+ st.session_state['bot_message'])-1, is_user=False)
+ else:
+ payload = {
+ "question": user_message,
+ "max_answer_length": API_CONFIG["ask_doubt"]["max_answer_length"],
+ "max_seq_length": API_CONFIG["ask_doubt"]["max_seq_length"],
+ "top_n": API_CONFIG["ask_doubt"]["top_n"],
+ "top_k": API_CONFIG["ask_doubt"]["top_k"]
+ }
+ bot_message, context, meta_data = self.ask_doubt.predict_ask_doubt(payload)
+ st.session_state['user_message'].append(user_message)
+ st.session_state['bot_message'].append(bot_message)
+ st.session_state['bot_message_context'].append(context)
+ st.session_state['bot_message_meta_data'].append(meta_data)
+
+ st.session_state['chat_input'] = None
+ with self.chat_container:
+ self._display_message(message_index=len(
+ st.session_state['user_message'])-1, is_user=True)
+ self._display_message(message_index=len(
+ st.session_state['bot_message'])-1, is_user=False, more_info=True)
+
+ def _display_message(self, message_index: int, is_user: bool, more_info: bool = False):
+ """Displays the message on chatbot UI which has the given index.
+ Message will be styled as user message or as bot message depending on is_user value.
+
+ Args:
+ message_index (int): _description_
+ is_user (bool): whether this is a user message or a bot message
+ """
+ if is_user:
+ if message_index < len(st.session_state['user_message']):
+ message(st.session_state['user_message'][message_index],
+ is_user=True, key=str(message_index) + '_user', avatar_style='adventurer-neutral', seed='Loki')
+ else:
+ if message_index < len(st.session_state['bot_message']):
+ message(st.session_state["bot_message"][message_index], key=str(
+ message_index), avatar_style='bottts', seed='Midnight')
+ if not self.viva_mode:
+ # TODO: currently tested for normal mode only
+ if more_info:
+ with st.expander("Get More info"):
+ if self.callback:
+ self.callback(
+ st.session_state['bot_message_meta_data'][message_index], **self.kwargs)
+ st.caption(
+ st.session_state['bot_message_context'][message_index])
+
+ def _display_message_pairs(self, message_pair_index: int, more_info: bool = False):
+ """Displays the message pair in the chat box UI
+
+ Args:video_selected
+ message_pair_index (int): _description_
+ """
+
+ # display user message
+ # Refer: https://www.dicebear.com/styles for changing avatar_style
+ if not self.viva_mode:
+ self._display_message(
+ message_index=message_pair_index, is_user=True, more_info=more_info)
+ self._display_message(
+ message_index=message_pair_index, is_user=False, more_info=more_info)
+ else:
+ self._display_message(
+ message_index=message_pair_index, is_user=False, more_info=more_info)
+ self._display_message(
+ message_index=message_pair_index, is_user=True, more_info=more_info)
+
+ def _display_chat_history(self):
+ """Displays a chat-box on the UI where messages will be displayed.
+
+ Returns:
+ _type_: Containers used to display chat messages and chat history
+ """
+
+ with self.chat_container:
+ if st.session_state['user_message'] or st.session_state['bot_message']:
+ total_message_pairs = max(len(st.session_state['user_message']), len(
+ st.session_state['bot_message']))
+ for i in range(total_message_pairs):
+ if total_message_pairs > 1 and i < total_message_pairs-1:
+ with self.chat_history_container:
+ self._display_message_pairs(message_pair_index=i)
+ else:
+ self._display_message_pairs(
+ message_pair_index=i, more_info=True)
+
+ def _set_chat_query(self):
+ """Sets the chat_query for processing and clears the input box
+ """
+ st.session_state['chat_input'] = st.session_state.chat_box
+ st.session_state.chat_box = ''
+
+ def _init(self):
+ """Displays the chat window on the UI.
+ """
+
+ with st.container():
+ st.markdown(
+ """
+
+ """,
+ unsafe_allow_html=True
+ )
+
+ # display all the previous chats till now
+ self._display_chat_history()
+
+ # query column for typing input and speech column for microphone input
+ query_column, speech_column = st.columns([22, 1])
+
+ with query_column:
+ st.text_input(label=self.chat_box_label,
+ key='chat_box', on_change=self._set_chat_query)
+
+ with speech_column:
+ audio_bytes = audio_recorder(
+ text="",
+ recording_color="#d63f31",
+ neutral_color="#6aa36f",
+ icon_name="microphone",
+ icon_size="2x",
+ sample_rate=16000,
+ key='mic'
+ )
+ if audio_bytes:
+ self.speech_input = convert_stt(audio_bytes)
diff --git a/webapp/ui/ui_manager.py b/webapp/ui/ui_manager.py
new file mode 100644
index 000000000..1a2b36d07
--- /dev/null
+++ b/webapp/ui/ui_manager.py
@@ -0,0 +1,197 @@
+"""Utility functions for handling displaying of different widgets on UI
+"""
+import os
+import base64
+import webvtt
+import streamlit as st
+import streamlit.components.v1 as components
+from pathlib import Path
+from ui.chatbot import Chatbot
+from common import time_to_seconds, load_course_material
+from config import DATASET_COURSE_BASE_DIR
+
+from utils.logging_handler import Logger
+
+# load the course material
+course_material = load_course_material(DATASET_COURSE_BASE_DIR)
+courses = course_material["course_names"]
+course_logos = [course_material[course]["logo_path"] for course in courses]
+
+def display_video(video_path: Path, start_time: int = 0, add_style=True, width=500, height=400):
+ """Displays a video player with the given width and height if add_style is True (default)
+
+ Args:
+ video_path (Path): _description_
+ add_style (bool, optional): _description_. Defaults to True.
+ width (int, optional): _description_. Defaults to 200.
+ height (int, optional): _description_. Defaults to 100.
+ """
+ if add_style:
+ # Set the CSS style to adjust the size of the video
+ thumbnail_style = f"""
+ video {{
+ width: {width}px !important;
+ height: {height}px !important;
+ }}
+ """
+ st.markdown(f'',
+ unsafe_allow_html=True)
+ st.video(str(video_path), start_time=start_time)
+
+
+def set_chat_window_style():
+ """Customizes the style of the chat window
+ """
+ css = """
+
+ """
+ components.html(css, height=0)
+
+
+def set_session_state(state_name, state_value):
+ st.session_state[state_name] = state_value
+
+
+def display_course_banner(course_name):
+ """Displays a course banner for the given course.
+
+ Args:
+ course_name (_type_): _description_
+ """
+ course_index = courses.index(course_name)
+ image_style = """
+ img {
+ # width: 1000px !important;
+ height: 200px !important;
+ }
+ """
+ st.markdown(f'', unsafe_allow_html=True)
+ st.image(str(course_logos[course_index]), use_column_width=True)
+
+
+def display_courses():
+ """Creates a display for courses arranged in 2 columns.
+ """
+ image_style = """
+ img {
+ width: 200px !important;
+ height: 100px !important;
+ }
+ """
+ # st.markdown(f'', unsafe_allow_html=True)
+ for i in range(0, len(course_logos), 2):
+ col_1, col_2 = st.columns([1, 1])
+ with col_1:
+ with st.container():
+ st.image(str(course_logos[i]), use_column_width=True)
+ st.button(label=courses[i], use_container_width=True, on_click=set_session_state, kwargs={
+ "state_name": "course_selected", "state_value": courses[i]})
+ with col_2:
+ if i+1 < len(course_logos):
+ with st.container():
+ st.image(str(course_logos[i+1]), use_column_width=True)
+ st.button(label=courses[i+1], use_container_width=True, on_click=set_session_state, kwargs={
+ "state_name": "course_selected", "state_value": courses[i+1]})
+
+
+def display_video_content(video_file: Path):
+ """Displays the video on the UI along with its subtitle on right side
+
+ Args:
+ video_file (Path): _description_
+ """
+ Logger.info("Selected video: {}".format(str(video_file)))
+ # Create two columns with a width ratio of 2:1
+ video_panel, text_panel = st.columns([1.5, 1])
+
+ # Content for the left column
+ with video_panel:
+ # st.header("Video Lectures")
+ display_video(video_path=video_file, add_style=False)
+
+ # Content for the right column
+ with text_panel:
+ doc_file = os.path.join("/".join(str(video_file).split("/")[:-1]), "subtitle-en.vtt")
+ subtitles = webvtt.read(doc_file)
+ transcript = ""
+ for subtitle in subtitles:
+ start, end = subtitle.start, subtitle.end
+ subtitle_text = " ".join(subtitle.text.strip().split("\n")).strip()
+ transcript += "{} --> {}\n{}\n\n".format(start, end, subtitle_text)
+
+ st.text_area(label="Video Transcript:",
+ value=transcript, height=280)
+
+ st.markdown("---")
+
+
+def display_qa_chat_bot():
+ """Displays a chat-bot on UI for QA
+ """
+ # display_chat()
+ chatbot = Chatbot(callback=callback_video_player, video_path=Path(
+ st.session_state['video_selected']))
+ chatbot.listen_for_inputs()
+
+
+def callback_video_player(meta_data, video_path: Path):
+ start_time = time_to_seconds(time_string=meta_data["start_timestamp"])
+ display_video(video_path=video_path, start_time=start_time)
+
+
+def display_viva_chat_bot(selected_course):
+ """Displays a chat-bot on UI for taking VIVA
+ """
+ # display_chat()
+ chatbot = Chatbot(chat_box_label="", viva_mode=True, selected_course=selected_course)
+ chatbot.listen_for_inputs()
+
+
+def display_video_tabs(selected_course):
+ """Creates a UI for selecting videos for the selected course. Videos are arranged in 3 columns.
+ All videos from the config path is displayed.
+ """
+ study_material = course_material[selected_course]["Study-Material"]
+ week_names = study_material["week_names"]
+
+ week_tabs = st.tabs(week_names)
+ for week_name, week_tab in zip(week_names, week_tabs):
+ with week_tab:
+ subtopic_names = study_material[week_name]["subtopic_names"]
+ col_1, col_2 = st.columns([1, 1])
+
+ for i in range(0, len(subtopic_names), 2):
+
+ with col_1:
+ video_path = study_material[week_name][subtopic_names[i]].get("video_file", None)
+ subtopic_name = subtopic_names[i]
+ if video_path is not None:
+ with st.container():
+ display_video(video_path=video_path, add_style=False)
+ st.button(label=subtopic_name, key=f"{week_tab}{i}", use_container_width=True, on_click=set_session_state, kwargs={
+ "state_name": "video_selected", "state_value": video_path })
+
+ with col_2:
+ if i+1 < len(subtopic_names):
+ video_path = study_material[week_name][subtopic_names[i+1]].get("video_file", None)
+ subtopic_name = subtopic_names[i+1]
+ if video_path is not None:
+ with st.container():
+ display_video(
+ video_path=video_path, add_style=False)
+ st.button(label=subtopic_name , key=f"{week_tab}{i+1}", use_container_width=True, on_click=set_session_state, kwargs={
+ "state_name": "video_selected", "state_value": video_path})
+
+ st.markdown("---")
+ st.button(label="Course Viva Exam", use_container_width=True, on_click=set_session_state, kwargs={
+ "state_name": "viva_mode",
+ "state_value": True
+ })
diff --git a/webapp/utils/__init__.py b/webapp/utils/__init__.py
new file mode 100644
index 000000000..e69de29bb
diff --git a/webapp/utils/errors.py b/webapp/utils/errors.py
new file mode 100644
index 000000000..8c5e9f444
--- /dev/null
+++ b/webapp/utils/errors.py
@@ -0,0 +1,9 @@
+"""class for handling errors"""
+
+
+class ResourceNotFoundException(Exception):
+ """Class for custom Exceptions"""
+
+ def __init__(self, message="Resource not found"):
+ self.message = message
+ super().__init__(self.message)
\ No newline at end of file
diff --git a/webapp/utils/logging_handler.py b/webapp/utils/logging_handler.py
new file mode 100644
index 000000000..853a459b7
--- /dev/null
+++ b/webapp/utils/logging_handler.py
@@ -0,0 +1,30 @@
+"""class and methods for logs handling."""
+
+import logging
+
+logging.basicConfig(
+ format='%(asctime)s:%(levelname)s:%(message)s', level=logging.DEBUG)
+
+
+class Logger():
+ """class def handling logs."""
+
+ @staticmethod
+ def info(message):
+ """Display info logs."""
+ logging.info(message)
+
+ @staticmethod
+ def warning(message):
+ """Display warning logs."""
+ logging.warning(message)
+
+ @staticmethod
+ def debug(message):
+ """Display debug logs."""
+ logging.debug(message)
+
+ @staticmethod
+ def error(message):
+ """Display error logs."""
+ logging.error(message)